Overview: Section
We've learned some of the very basics about research studies that compare two or more samples of one variable. Now we will explore this topic in more detail. We first need to learn a few terms. These include:
- experimental unit
- explanatory variable
- treatment
- response (outcome) variable
- confounding variable
The experimental unit is the smallest basic object to which one can assign different conditions (treatments.) In research studies, the experimental unit does not always have to be a person. In fact, the statistical terminology that is associated with research studies actually came from studies done in agriculture. Examples of an experimental unit include:
- person
- animal
- plant
- set of twins
- married couple
- plot of land
- building
The explanatory variable is the variable used to form or define the different samples. In randomized experiments, one explanatory variable is the variable that is used to explain differences in the groups. In this instance, the explanatory variable can also be called a treatment when each experimental unit is randomly assigned a certain condition. Examples of explanatory variables include:
- gender
- type of plant
- type of drug
- type of medical procedure
- teaching method
You should note that gender and type of plant cannot be called treatments because one cannot randomly assign gender or type of plant.
The response (outcome) variable is the outcome of the study that is either measured or counted. We have seen the response (outcome) variable in previous lessons. Examples of response variables include:
- height
- weight
- temperature
- classification of whether a person is a vegetarian
- classification of symptom severity for an illness
Of course, some variables may play different roles in different studies. For example, in an experiment to see whether a new diet might be held in reducing your weight; weight is the response variable and whether you used the new diet or not would be the explanatory variable. On the other hand, in an observational study to examine how a person's weight might affect their heart rate; weight would play the role of an explanatory variable and heart rate would be the response variable.
A confounding variable is a variable that affects the response variable and is also related to the explanatory variable. The effect of a confounding variable on the response variable cannot be separated from the effect of the explanatory variable. Therefore, we cannot clearly determine that the explanatory variable is solely responsible for any effect on the response or outcome variable when a confounding variable is present. Confounding variables are problematic in observational studies.
Example 2.3 Section
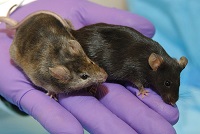
Laboratory experiments conducted in the 1980s showed that pregnant mice exposed to high does of ultrasound gave birth to lower weight infant mice than unexposed mice (in fact the higher the dose the greater the effect on birthweights). This worried obstetricians who feared that sonograms given to women during pregnancy might cause lower weights in their children. Researchers at Johns Hopkins University Hospital then examined the birthweights of infants of mothers who had sonograms versus those whose mothers had no such exposure. They found that the 1598 infants who had been exposed averaged a couple of ounces lower in weight than the 944 infants whose mothers did not have a sonogram. However, the women who got sonograms were more likely to have had twins in the past and were more likely to be over 40 years old. Having twins or being over 40 are examples of confounding variables in this study since they provide an alternate explanation for the data. You can not tell whether it was the sonogram that caused the lower birth weights or just the confounding medical reasons for getting the sonogram in the first place. Later experimental evidence in humans did not show sonograms to have any effect (see Abramowicz et al, 2008 for a review).