Lesson 12: Tests for Variances
Lesson 12: Tests for VariancesContinuing our development of hypothesis tests for various population parameters, in this lesson, we'll focus on hypothesis tests for population variances. Specifically, we'll develop:
- a hypothesis test for testing whether a single population variance \(\sigma^2\) equals a particular value
- a hypothesis test for testing whether two population variances are equal
12.1 - One Variance
12.1 - One VarianceYeehah again! The theoretical work for developing a hypothesis test for a population variance \(\sigma^2\) is already behind us. Recall that if you have a random sample of size n from a normal population with (unknown) mean \(\mu\) and variance \(\sigma^2\), then:
\(\chi^2=\dfrac{(n-1)S^2}{\sigma^2}\)
follows a chi-square distribution with n−1 degrees of freedom. Therefore, if we're interested in testing the null hypothesis:
\(H_0 \colon \sigma^2=\sigma^2_0\)
against any of the alternative hypotheses:
\(H_A \colon\sigma^2 \neq \sigma^2_0,\quad H_A \colon\sigma^2<\sigma^2_0,\text{ or }H_A \colon\sigma^2>\sigma^2_0\)
we can use the test statistic:
\(\chi^2=\dfrac{(n-1)S^2}{\sigma^2_0}\)
and follow the standard hypothesis testing procedures. Let's take a look at an example.
Example 12-1
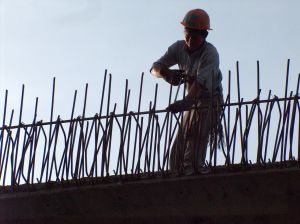
A manufacturer of hard safety hats for construction workers is concerned about the mean and the variation of the forces its helmets transmits to wearers when subjected to an external force. The manufacturer has designed the helmets so that the mean force transmitted by the helmets to the workers is 800 pounds (or less) with a standard deviation to be less than 40 pounds. Tests were run on a random sample of n = 40 helmets, and the sample mean and sample standard deviation were found to be 825 pounds and 48.5 pounds, respectively.
Do the data provide sufficient evidence, at the \(\alpha = 0.05\) level, to conclude that the population standard deviation exceeds 40 pounds?
Answer
We're interested in testing the null hypothesis:
\(H_0 \colon \sigma^2=40^2=1600\)
against the alternative hypothesis:
\(H_A \colon\sigma^2>1600\)
Therefore, the value of the test statistic is:
\(\chi^2=\dfrac{(40-1)48.5^2}{40^2}=57.336\)
Is the test statistic too large for the null hypothesis to be true? Well, the critical value approach would have us finding the threshold value such that the probability of rejecting the null hypothesis if it were true, that is, of committing a Type I error, is small... 0.05, in this case. Using Minitab (or a chi-square probability table), we see that the cutoff value is 54.572:
That is, we reject the null hypothesis in favor of the alternative hypothesis if the test statistic \(\chi^2\) is greater than 54.572. It is. That is, the test statistic falls in the rejection region:
Therefore, we conclude that there is sufficient evidence, at the 0.05 level, to conclude that the population standard deviation exceeds 40.
Of course, the P-value approach yields the same conclusion. In this case, the P-value is the probablity that we would observe a chi-square(39) random variable more extreme than 57.336:
As the drawing illustrates, the P-value is 0.029 (as determined using the chi-square probability calculator in Minitab). Because \(P = 0.029 ≤ 0.05\), we reject the null hypothesis in favor of the alternative hypothesis.
Do the data provide sufficient evidence, at the \(\alpha = 0.05\) level, to conclude that the population standard deviation differs from 40 pounds?
Answer
In this case, we're interested in testing the null hypothesis:
\(H_0 \colon \sigma^2=40^2=1600\)
against the alternative hypothesis:
\(H_A \colon\sigma^2 \neq 1600\)
The value of the test statistic remains the same. It is again:
\(\chi^2=\dfrac{(40-1)48.5^2}{40^2}=57.336\)
Now, is the test statistic either too large or too small for the null hypothesis to be true? Well, the critical value approach would have us dividing the significance level \(\alpha = 0.05\) into 2, to get 0.025, and putting one of the halves in the left tail, and the other half in the other tail. Doing so (and using Minitab to get the cutoff values), we get that the lower cutoff value is 23.654 and the upper cutoff value is 58.120:
That is, we reject the null hypothesis in favor of the two-sided alternative hypothesis if the test statistic \(\chi^2\) is either smaller than 23.654 or greater than 58.120. It is not. That is, the test statistic does not fall in the rejection region:
Therefore, we fail to reject the null hypothesis. There is insufficient evidence, at the 0.05 level, to conclude that the population standard deviation differs from 40.
Of course, the P-value approach again yields the same conclusion. In this case, we simply double the P-value we obtained for the one-tailed test yielding a P-value of 0.058:
\(P=2\times P\left(\chi^2_{39}>57.336\right)=2\times 0.029=0.058\)
Because \(P = 0.058 > 0.05\), we fail to reject the null hypothesis in favor of the two-sided alternative hypothesis.
The above example illustrates an important fact, namely, that the conclusion for the one-sided test does not always agree with the conclusion for the two-sided test. If you have reason to believe that the parameter will differ from the null value in a particular direction, then you should conduct the one-sided test.
12.2 - Two Variances
12.2 - Two VariancesLet's now recall the theory necessary for developing a hypothesis test for testing the equality of two population variances. Suppose \(X_1 , X_2 , \dots, X_n\) is a random sample of size n from a normal population with mean \(\mu_X\) and variance \(\sigma^2_X\). And, suppose, independent of the first sample, \(Y_1 , Y_2 , \dots, Y_m\) is another random sample of size m from a normal population with \(\mu_Y\) and variance \(\sigma^2_Y\). Recall then, in this situation, that:
\(\dfrac{(n-1)S^2_X}{\sigma^2_X} \text{ and } \dfrac{(m-1)S^2_Y}{\sigma^2_Y}\)
have independent chi-square distributions with n−1 and m−1 degrees of freedom, respectively. Therefore:
\( {\displaystyle F=\frac{\left[\frac{\color{red}\cancel {\color{black}(n-1)} \color{black}S_{X}^{2}}{\sigma_{x}^{2}} /\color{red}\cancel {\color{black}(n- 1)}\color{black}\right]}{\left[\frac{\color{red}\cancel {\color{black}(m-1)} \color{black}S_{Y}^{2}}{\sigma_{Y}^{2}} /\color{red}\cancel {\color{black}(m-1)}\color{black}\right]}=\frac{S_{X}^{2}}{S_{Y}^{2}} \cdot \frac{\sigma_{Y}^{2}}{\sigma_{X}^{2}}} \)
follows an F distribution with n−1 numerator degrees of freedom and m−1 denominator degrees of freedom. Therefore, if we're interested in testing the null hypothesis:
\(H_0 \colon \sigma^2_X=\sigma^2_Y\) (or equivalently \(H_0 \colon\dfrac{\sigma^2_Y}{\sigma^2_X}=1\))
against any of the alternative hypotheses:
\(H_A \colon \sigma^2_X \neq \sigma^2_Y,\quad H_A \colon \sigma^2_X >\sigma^2_Y,\text{ or }H_A \colon \sigma^2_X <\sigma^2_Y\)
we can use the test statistic:
\(F=\dfrac{S^2_X}{S^2_Y}\)
and follow the standard hypothesis testing procedures. When doing so, we might also want to recall this important fact about the F-distribution:
\(F_{1-(\alpha/2)}(n-1,m-1)=\dfrac{1}{F_{\alpha/2}(m-1,n-1)}\)
so that when we use the critical value approach for a two-sided alternative:
\(H_A \colon\sigma^2_X \neq \sigma^2_Y\)
we reject if the test statistic F is too large:
\(F \geq F_{\alpha/2}(n-1,m-1)\)
or if the test statistic F is too small:
\(F \leq F_{1-(\alpha/2)}(n-1,m-1)=\dfrac{1}{F_{\alpha/2}(m-1,n-1)}\)
Okay, let's take a look at an example. In the last lesson, we performed a two-sample t-test (as well as Welch's test) to test whether the mean fastest speed driven by the population of male college students differs from the mean fastest speed driven by the population of female college students. When we performed the two-sample t-test, we just assumed the population variances were equal. Let's revisit that example again to see if our assumption of equal variances is valid.
Example 12-2
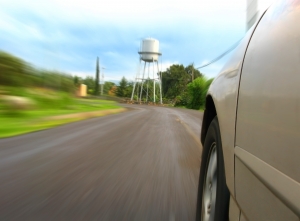
A psychologist was interested in exploring whether or not male and female college students have different driving behaviors. The particular statistical question she framed was as follows:
Is the mean fastest speed driven by male college students different than the mean fastest speed driven by female college students?
The psychologist conducted a survey of a random \(n = 34\) male college students and a random \(m = 29\) female college students. Here is a descriptive summary of the results of her survey:
Males (X) | Females (Y) |
---|---|
\(n = 34\) |
\(m = 29\) \(\bar{y} = 90.9\) \(s_y = 12.2\) |
Is there sufficient evidence at the \(\alpha = 0.05\) level to conclude that the variance of the fastest speed driven by male college students differs from the variance of the fastest speed driven by female college students?
Answer
We're interested in testing the null hypothesis:
\(H_0 \colon \sigma^2_X=\sigma^2_Y\)
against the alternative hypothesis:
\(H_A \colon\sigma^2_X \neq \sigma^2_Y\)
The value of the test statistic is:
\(F=\dfrac{12.2^2}{20.1^2}=0.368\)
(Note that I intentionally put the variance of what we're calling the Y sample in the numerator and the variance of what we're calling the X sample in the denominator. I did this only so that my results match the Minitab output we'll obtain on the next page. In doing so, we just need to make sure that we keep track of the correct numerator and denominator degrees of freedom.) Using the critical value approach, we divide the significance level \(\alpha = 0.05\) into 2, to get 0.025, and put one of the halves in the left tail, and the other half in the other tail. Doing so, we get that the lower cutoff value is 0.478 and the upper cutoff value is 2.0441:
Because the test statistic falls in the rejection region, that is, because \(F = 0.368 ≤ 0.478\), we reject the null hypothesis in favor of the alternative hypothesis. There is sufficient evidence at the \(\alpha = 0.05\) level to conclude that the population variances are not equal. Therefore, the assumption of equal variances that we made when performing the two-sample t-test on these data in the previous lesson does not appear to be valid. It would behoove us to use Welch's t-test instead.
12.3 - Using Minitab
12.3 - Using MinitabIn each case, we'll illustrate how to perform the hypothesis tests of this lesson using summarized data.
Hypothesis Test for One Variance
-
Under the Stat menu, select Basic Statistics, and then select 1 Variance...:
-
In the pop-up window that appears, in the box labeled Data, select Sample standard deviation (or alternatively Sample variance). In the box labeled Sample size, type in the size n of the sample. In the box labeled Sample standard deviation, type in the sample standard deviation. Click on the box labeled Perform hypothesis test, and in the box labeled Value, type in the Hypothesized standard deviation (or alternatively the Hypothesized variance):
-
Click on the button labeled Options... In the pop-up window that appears, for the box labeled Alternative, select either less than, greater than, or not equal depending on the direction of the alternative hypothesis:
Then, click on OK to return to the main pop-up window.
-
Then, upon clicking OK on the main pop-up window, the output should appear in the Session window:
95% Confidence IntervalsMethod CI for
StDevCI for
VarianceChi-Square (39.7, 62.3) (1578, 3878) Method Test
StatisticDF P-Value Chi-Square 57.34 39 0.059
Hypothesis Test for Two Variances
-
Under the Stat menu, select Basic Statistics, and then select 2 Variances...:
-
In the pop-up window that appears, in the box labeled Data, select Sample standard deviations (or alternatively Sample variances). In the box labeled Sample size, type in the size n of the First sample and m of the Second sample. In the box labeled Standard deviation, type in the sample standard deviations for the First and Second samples:
-
Click on the button labeled Options... In the pop-up window that appears, in the box labeled Value, type in the Hypothesized ratio of the standard deviations (or the Hypothesized ratio of the variances). For the box labeled Alternative, select either less than, greater than, or not equal depending on the direction of the alternative hypothesis:
Then, click on OK to return to the main pop-up window.
-
Then, upon clicking OK on the main pop-up window, the output should appear in the Session window:
Test and CI for Two Variances
Method
Null hypothesis Sigma(1) / Sigma(2) = 1
Alternative hypothesis Sigma(1) / Sigma(2) not = 1
Significance level Alpha = 0.05
StatisticsSample N StDev Variance 1 29 12.200 148.840 2 34 20.100 404.010 Ratio of standard deviations = 0.607
Ratio of variances = 0.368
95% Confidence IntervalsDistribution
of DataCI for StDev Ratio CI for
Variance RatioNormal (0.425, 0.877) (0.180, 0.770) Method DF1 DF2 Test
StatisticP-Value F Test (normal) 28 33 0.37 0.009
-