Lesson 28: Choosing Appropriate Statistical Methods
Lesson 28: Choosing Appropriate Statistical MethodsIf we take a look back at where we've been this semester, we can quickly get the feeling as if we hiked the entire length of the 2,180 mile long Appalachian Trail. Just think about it! Among other things, we've learned about:
- Point estimation, including maximum likelihood estimation, method of moments, and sufficiency
- Confidence intervals for means, differences in two means, variances, proportions, and differences in two proportions
- Determining the sample size necessary to estimate a parameter with a certain error \(\epsilon\)
- Linear regression as a way of estimating and testing for the existence of a linear relationship between two continuous variables
- Hypothesis testing, including best critical regions and likelihood ratio tests
- Hypothesis tests for means, the equality of two means, variances, proportions and the equality of two proportions
- Determining the sample size necessary to conduct a hypothesis test for a parameter with a certain power
- One-factor analysis of variance as a way of testing for the equality of three or more population means
- Two-factor analysis of variance as a way of testing for the effect of one or more qualitative factors on a continuous variable
- Chi-square goodness-of-fit tests and contingency tables
- Using order statistics to derive distribution-free confidence intervals for percentiles
- Nonparametric methods, such as the sign test, the Wilcoxon signed rank test, the run test, and the test for randomness
- Using the Kolmogorov-Smirnov test statistic to test for the equality of a particular distribution function \(F_{0}(x)\)
- Bayesian methods
That's all well and good, but we haven't really yet had much practice with putting it altogether to choose which of the above statistical methods would be most appropriate for any given situation. For example, suppose we were interested in learning how many times each semester Penn State students go "home." What statistical method(s) would be most appropriate for answering our research question? Or, suppose we were interested in determining whether or not a higher percentage of Alaskans commit suicide than non-Alaskans. What statistical methods could we use? These are the kinds of questions we'll tackle in this lesson. The algorithm that I propose in this lesson is perhaps not flawless, but by using it, I can almost always figure out what kind of analysis is appropriate for any given situation. Choosing the correct analysis depends, at the very least, on the answer to the following four questions:
- What type of response variable do we have? More specifically, is it a continuous or categorical variable?
- How many groups are being studied or compared? Is it one, two, or more?
- What is the research question? Are we asking "is it this," so that we need to conduct a hypothesis test? Or are we asking "what is it," so that we need to calculate a point estimate or a confidence interval?
- What assumptions can we safely make about the data? Can we assume that the data are normally distributed? Can we assume the variances of two populations are equal? Are the groups dependent or independent?
As you'll soon see, upon working through the material in this lesson, choosing the correct analysis hinges on the answers to these questions. We'll first start by considering the methods that are available to us when we have one categorical (or perhaps, more specifically, binary) variable. Then, we'll move to the situation in which we have one continuous response variable. And, then two continuous measurements, before concluding with some practice questions.
28.1 - One Categorical Response
28.1 - One Categorical ResponseLet's start by considering only those methods that are appropriate for the case in which we have a binary response. You know... that means just two possible outcomes... such as, smoker or non-smoker? blue eyes or not? loves statistics or doesn't? Then, consider only those methods that are appropriate for the case in which we are studying just one group... such as, college seniors, women over the age of 60, or ash trees.
One Group with a Binary Response
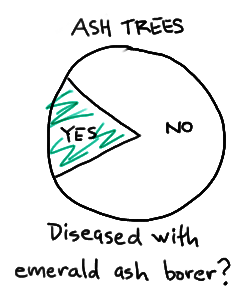
Suppose we are interested in learning the extent to which the population of ash trees in the eastern United States is diseased with the emerald ash borer. Well, in that situation, we are studying just one group, namely, the population of ash trees in the eastern United States. Then, we take a random sample of n ash trees from that population and determine whether or not each tree is diseased with the emerald ash borer. In that situation, we have a binary response, namely, either the tree is or is not diseased. As soon as we determine that we are studying one group with a binary response, we should be thinking proportions, proportions, proportions. That is, a proportion is a natural way of summarizing the observed data, so therefore the statistical methods we should consider using must necessarily concern proportions. Specifically, our options are:
- performing a Z-test for one proportion
- performing a chi-square test
- calculating a Z-interval for one proportion
What we choose depends on our specific research question. If we are just interested in determining whether a majority \((p > 0.50)\) of the ash trees are diseased, a Z-test for one proportion will suffice. If we have some previous idea about the value of the proportion, \(p_0\), say of diseased trees in mind, and don't care whether the proportion is now smaller or larger than \(p_0\), then a chi-square test will suffice, as it allows for two-sided alternative hypotheses. Of course, we could just as well perform a two-sided Z-test for one proportion in that case. The P-values, and hence the final decisions, will be the same. If, on the other hand, we are only interested in estimating the unknown proportion p of diseased ash trees in the eastern United States, then we should calculate a 95% Z-interval for one proportion.
I always like to say that deciding whether to go the hypothesis test or confidence interval route depends on whether the research question involves a "is it this" or "what is it" question. That is, the research question "is the proportion of diseased trees different from 0.4?" involves conducting a hypothesis test, whereas the research question "what is the proportion of diseased trees?" involves calculating a confidence interval.
Once we've determined the appropriate statistical method, we can turn to a statistical analysis package, such as Minitab, to help with the analysis. In Minitab, we use the commands:
- Stat >> Basic Stat >> 1 Proportion... to conduct a Z-test for one proportion or to calculate a Z-interval for one proportion
- Stat >> Tables >> Cross Tabulation and Chi-Square... to conduct a chi-square test
The details about how to perform the analyses in Minitab, as well as about the assumptions that must be made, can be found in the relevant lessons.
Example 28-1
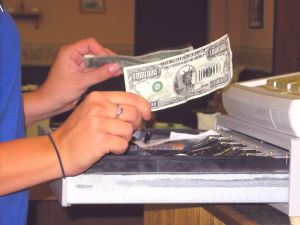
Do a majority of college students work during the semester?
Answer
The research question involves the study of one group, namely college students. The research question involves a binary response... either a student does or does not work during the semester. The research question is an "is it this?" question, and therefore involves conducting a hypothesis test. If p is the (unknown) proportion of college students who work during the semester, then we are specifically interested in testing the null hypothesis \(H_0: p = 0.50\) against the alternative hypotheses \(H_A: p > 0.50\). We can enter the resulting data into Minitab and then ask Minitab to conduct a Z-test for one proportion for us.
Incidentally, this discussion of whether or not we should conduct a hypothesis test or calculate a confidence interval is a bit like splitting hairs. That's because, as you might recall, a confidence interval can always be used to answer an "is it this?" question, too. For example, in this case, we could calculate a confidence interval for p, and then if the confidence interval only contains values greater than 0.50, then we can reject the null hypothesis \(H_0: p = 0.50\) in favor of the alternative hypotheses \(H_A: p > 0.50\). In practice, most statisticians do both, that is, conduct and report the results of both the hypothesis test and the confidence interval.
Example 28-2
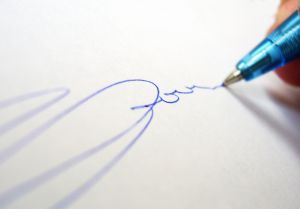
What proportion of college students have an E in their last name?
Answer
The research question involves the study of one group, namely college students. The research question involves a binary response... either a student does or does not have an E in his or her last name. The research question is a "what is it?" question, and therefore involves calculating a confidence interval. If p is the (unknown) proportion of college students who have an E in their last name, then we are specifically interested in estimating p. We can enter the resulting data into Minitab and then ask Minitab to calculate a Z-interval for one proportion for us.
Two Groups with a Binary Response
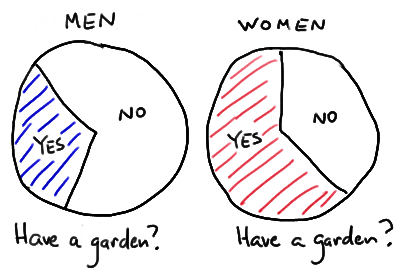
Suppose we are interested in learning the extent to which the population of American men and the population of American women have a garden. In this case, we are clearly studying two groups, namely, the population of American men and the population of American women. Then, we take a random sample of \(n_1\) men and \(n_2\) women from each population and determine whether or not each person has a garden. In this case, we have a binary response, namely, either the person has a garden or does not. As soon as we determine that we are studying two groups with a binary response, we should be thinking two proportions, two proportions, two proportions. That is, a proportion is a natural way of summarizing the data observed from each population, so therefore the statistical methods we should consider using must necessarily concern two proportions. Specifically, our options are:
- performing a Z-test for two proportions
- performing a chi-square test
- calculating a Z-interval for two proportions
What we choose depends on our specific research question. Again, if the research question is an "is it this?" question, then we'd want to conduct a hypothesis test, whereas if it's a "what is it?" question, we'd want to calculate a confidence interval. For example, if we're only interested in determining whether or not the two population proportions \(p_1\) and \(p_2\) are equal, then either the Z-test for two proportions or the chi-square test would suffice. On the other hand, if we are interested in quantifying the extent to which the two proportions differ (or not), then we'd better calculate a confidence interval.
Again, once we've determined the appropriate statistical method, we can turn to a statistical analysis package, such as Minitab, to help with the analysis. In Minitab, we use the commands:
- Stat >> Basic Stat >> 2 Proportions... to conduct a Z-test for two proportions or to calculate a Z-interval for two proportions
- Stat >> Tables >> Cross Tabulation and Chi-Square... to conduct a chi-square test
The details about how to perform the analyses in Minitab, as well as about the assumptions that must be made, can be found in the relevant lessons.
Example 28-3
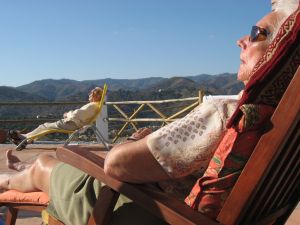
Do elderly males and elderly females snore at a different rate?
Answer
The research question involves the study of two groups, namely elderly males and elderly females. The research question involves a binary response... either a person does or does not snore. The research question is an "is it this?" question, and therefore involves conducting a hypothesis test. In this case, the question involves determining whether or not the difference in the two proportions \(p_1\) and \(p_2\) is 0. That is, if p1 is the (unknown) proportion of elderly males who snore, and \(p_2\) is the (unknown) proportion of elderly females who snore, then we are specifically interested in testing the null hypothesis \(H_0: p_1−p_2 = 0\) against the alternative hypotheses \(H_A: p_1−p_2 ≠ 0\). We can enter the resulting data into Minitab and then ask Minitab to conduct either a chi-square test or a Z-test for two proportions for us.
All of the examples that we have considered thus far on this page have involved a binary response variable. Let's now consider the possibility that the response is a general categorical variable.
Two or More Groups with a Categorical Response
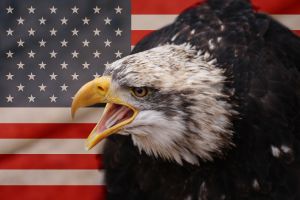
Suppose we are interested in determining whether preference for one of four presidential candidates is independent of a voter's affiliation with a major political party (Democrat, Republican, or Independent). In this case, we are studying three groups, namely, the population of Democrat voters, the population of Republican voters, and the population of Independent voters. Then, we take a random sample of \(n_1\) Democrats, \(n_2\) Republicans, and \(n_3\) Independents, and determine whether each person prefers candidate A, B, C, or D for president. In this case, we have a general categorical response, namely, either the person prefers candidate A, B, C or D. As soon as we determine that we are studying two or more groups with a categorical response, we should be thinking chi-square test. In Minitab, we use the commands Stat >> Tables >> Cross Tabulation and Chi-Square... to conduct the test.
Example 28-4
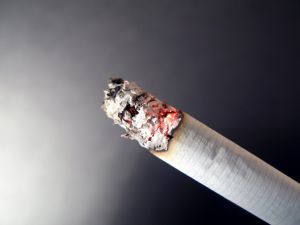
Is the rate of smoking independent of semester standing? One-hundred randomly selected students from each of the four classes (freshmen, sophomores, juniors, and seniors) are asked about their smoking behavior (never, a few times, regularly, addicted).
Answer
The research question involves the study of four groups, namely freshmen, sophomores, juniors, and seniors. The research question involves a categorical response... either a person classifies him- or herself as having never smoked, as having smoked a few times, as a regular smoker, or as being completely addicted. The research question involves assessing the independence of the two variables, smoking and semester standing. In summarizing the data, we determine the proportion of freshmen falling into each category of smokers, the proportion of sophomores falling into each category of smokers, the proportion of juniors falling into each category of smokers, and the proportion of seniors falling into each category of smokers. We can enter the resulting data into Minitab and then ask Minitab to conduct a chi-square test for us.
28.2 - One Continuous Response
28.2 - One Continuous ResponseNow, let's turn our attention towards those methods that are appropriate for the case in which we have a continuous response. You know... that means a response that falls in an interval of values... such as, weight (in pounds), temperature (in degrees Fahreneit), or grade on a statistics final exam. First, let's consider only those methods that are appropriate for the case in which we are studying just one group... such as, high school freshmen, six-year-old girls, or moray eels.
One Group with a Continuous Response
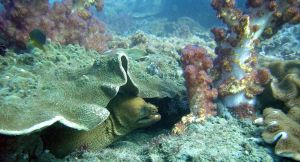
Suppose we are interested in learning about the length of the population of moray eels. In that case, we are studying just one group, namely, the population of moray eels. Then, we take a random sample of n moray eels from that population and determine the length of each specimen selected. In that situation, we have a continuous response, namely, the length of the eel. As soon as we determine that we are studying one group with a continuous response, we should be thinking means, means, means, or .... errr.... medians, medians, medians. Which is the more appropriate summary statistic, of course, depends on the distribution of the data, that is, whether it is symmetric or skewed. At any rate, the mean or the median is a natural way of summarizing the observed data, so therefore the statistical methods we should potentially use must necessarily concern either means of medians. Specifically, our options are:
- performing a t-test for one mean
- performing a sign test or signed rank test for one median
- calculating a t-interval for one mean
- calculating a distribution-free confidence interval for a median or a general percentile
Again, what we choose depends on our specific research question. If the research question is an "is it this?" question, then we'd want to conduct a hypothesis test, whereas if it's a "what is it?" question, we'd want to calculate a confidence interval. Once we determine the appropriate statistical method, Minitab can do the dirty work for us using these commands:
- Stat >> Basic Stat >> 1-Sample t... to conduct a t-test for one mean or to calculate a t-interval for one mean
- Stat >> Nonparametrics >> 1-Sample Sign... to conduct a sign test
- Stat >> Nonparametrics >> 1-Sample Wilcoxon... to conduct a signed rank test for one median or to calculate a distribution-free confidence interval for a median
The details about how to perform the analyses in Minitab, as well as about the assumptions that must be made in each case, can be found in the relevant lessons.
Example 28-5
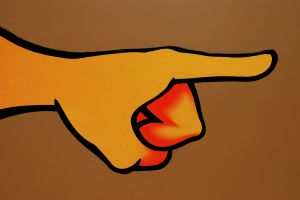
What is the mean length of the pointer finger of the population of college students?
Answer
The research question involves the study of one group, namely college students. The research question involves a continuous response... the length of the pointer finger of a randomly selected college student. The research question is a "what is it?" question, and therefore involves calculating a confidence interval. If \(\mu\) is the (unknown) mean length of the pointer finger of college students, then we are specifically interested in estimating \(\mu\). We can enter the resulting data into Minitab and then ask Minitab to calculate a t-interval for one mean for us.
Example 28-6
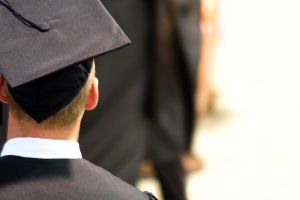
Is the mean IQ, as measured by the Stanford-Binet IQ test, of the population of graduating college seniors greater than 115?
Answer
The research question involves the study of one group, namely graduating college seniors. The research question involves a continuous response... the score on the Stanford-Binet IQ test. The research question is a "is it this?" question, and therefore involves conducting a hypothesis test. If \(\mu\) is the (unknown) mean IQ score of graduating college seniors, then we are specifically interested in testing the null hypothesis \(H_0: \mu = 115\) against the alternative hypothesis \(H_A: \mu > 115\). We can enter the resulting data into Minitab and then ask Minitab to conduct a t-test for one mean for us.
Example 28-7
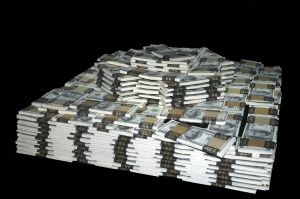
Is the median annual income of American households greater than \$40,000?
Answer
The research question involves the study of one group, namely American households. The research question involves a continuous response... annual income (in dollars). The research question is a "is it this?" question, and therefore involves conducting a hypothesis test. At this point, because the response is continuous we could conduct a hypothesis test about the mean or the median. However, because it is well known that the distribution of American incomes is highly skewed, the median is a better measure of the "center" of the income distribution. Therefore, our analysis should probably concern the median. That said, if m is the (unknown) median annual income of American households, then we are specifically interested in testing the null hypothesis \(H_0: m = 40,000\) against the alternative hypothesis \(H_A: m > 40,000\). We can enter the resulting data into Minitab and then ask Minitab to conduct either a sign test or a signed rank test for one median for us.
Two Paired Groups with a Continuous Response
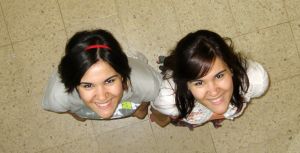
Suppose we are interested in comparing the heights of first-born and second-born twins. Then, we have two groups, namely that of the first-born twins and that of the second-born twins. The groups have a special characteristic, however, in that they are not independent. As you know, we say they are paired. Therefore, any analysis we perform would have to take this dependence into account. The response, height, is of course, continuous. Therefore, our analysis involves two paired groups with a continuous response, and hence our options are:
- performing a paired t-test for a mean difference
- performing a sign test or signed rank test for a median difference
- calculating a paired t-interval for a mean difference
- calculating a distribution-free confidence interval for a median (or general percentile) difference
Again, what we choose depends on our specific research question. If the research question is an "is it this?" question, then we'd want to conduct a hypothesis test, whereas if it's a "what is it?" question, we'd want to calculate a confidence interval. Once we determine the appropriate statistical method, Minitab can do the dirty work for us using these commands:
- Stat >> Basic Stat >> Paired t... to conduct a paired t-test for a mean difference or to calculate a paired t-interval for a mean difference
- Stat >> Nonparametrics >> 1-Sample Sign... to conduct a sign test for a median difference
- Stat >> Nonparametrics >> 1-Sample Wilcoxon... to conduct a signed rank test for a median difference or to calculate a distribution-free confidence interval for a median difference
The details about how to perform the analyses in Minitab, as well as about the assumptions that must be made in each case, can be found in the relevant lessons.
Example 28-8
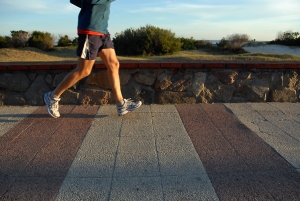
Do people's pulse rates increase after exercise?
Answer
The research question involves the study of one group, namely people. Oops, actually if you think about it, the question involves the study of two groups, people, before exercise and people after exercise. Although the research question doesn't specifically suggest this, we should all know by now that it would be a good idea to collect the data in a paired way, that is, to measure the same people before and after exercise. Doing otherwise would introduce needless variability into the data. By measuring the same people twice, of course, removes the independence of the groups, and hence we should be thinking paired, paired, paired.
Because the research question involves a continuous response... the pulse rate, we should be thinking mean, mean, mean or median, median, median. So, we have a paired, paired, paired, mean, mean, mean or a paired, paired, paired, median, median, median. (I've been clearly writing too long today.) At any rate, the research question is clearly a "is it this?" question. It is? Clearly? Well, if \(\mu_D = \mu_{After} − \mu_{Before}\), is the (unknown) mean difference in the pulse rates, then we are specifically interested in testing the null hypothesis \(H_0: \mu_D = 0\) against the alternative hypothesis \(H_A: \mu_D > 0\). We can enter the resulting data into Minitab and then ask Minitab to conduct either a paired t-test for one mean or, alternatively, a sign test or signed-rank test for the median difference. Of course, if we went a step further, we could also ask Minitab to calculate a confidence interval for us, so that we can quantify how different the pulse rates are before and after exercise.
Two Independent Groups with a Continuous Response
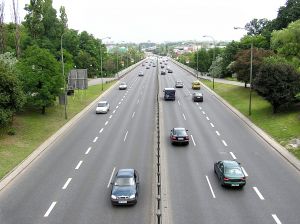
Suppose we are interested in comparing the gas mileage of two different vehicles, Toyota Camry and Volkswagen Passat, say. In this case, we have two independent groups, namely that of Toyota Camry vehicles and that of Volkswagen Passat vehicles. The response, gas mileage, is a continuous measurement. Therefore, our analysis would involve two independent groups with a continuous response, and hence our options are:
- performing a two-sample t-test for the difference in two means
- performing a two-sample Wilcoxon test for a difference in two medians
- calculating a two-sample t-interval for the difference in two means
Again, what we choose depends on our specific research question. If the research question is an "is it this?" question, then we'd want to conduct a hypothesis test, whereas if it's a "what is it?" question, we'd want to calculate a confidence interval. Once we determine the appropriate statistical method, Minitab can do the dirty work for us using these commands:
- Stat >> Basic Stat >> 2-Sample t... to conduct a t-test for the difference in two means or to calculate a t-interval for the difference in two means
- Stat >> Nonparametrics >> Mann-Whitney... to conduct a variation of the two-sample Wilcoxon test for a difference in two medians
The details about how to perform the analyses in Minitab, as well as about the assumptions that must be made in each case, can be found in the relevant lessons.
Example 28-9
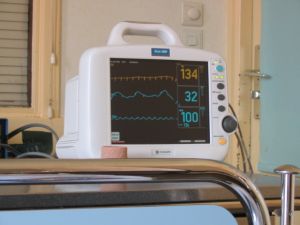
Do the resting pulse rates of adult males and females differ?
Answer
The research question involves the study of two independent groups, namely that of adult males and females. The research question involves a continuous response... resting pulse rates. The research question is a "is it this?" question, and therefore involves conducting a hypothesis test. If \(\mu_M\) is the (unknown) mean pulse rate of adult males, and \(\mu_F\) is the (unknown) mean pulse rate of adult females, then we are specifically interested in testing the null hypothesis:
\(H_0: \mu_M − \mu_F = 0\)
against the alternative hypothesis:
\(H_A: \mu_M − \mu_F ≠ 0\)
We can enter the resulting data into Minitab and then ask Minitab to conduct a two-sample t-test for one mean for us. Of course, we should check, as always, for the normality of the data and the equality of the population variances.
More than Two Independent Groups with a Continuous Response??
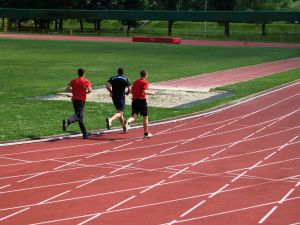
Suppose we are interested in comparing the average 5-kilometer race times of four different age groups. Because we have four independent groups and one continuous response, namely the race times, we would want to conduct a one-factor analysis of variance. If we were interested in testing whether a second factor, such as gender, had an effect on race times, then we would want to conduct a two-factor analysis of variance. We'd, of course, have to check the necessary assumptions, but once we did that, we could let Minitab do the analysis for us using these commands:
- Stat >> ANOVA >> One-way... to conduct a one-factor analysis of variance with the grouping variable in one column and the response in a second column
- Stat >> ANOVA >> One-way (Unstacked)... to conduct a one-factor analysis of variance with each group's responses being recorded in a different column
- Stat >> ANOVA >> Two-way... to conduct a two-factor analysis of variance
The details about how to perform the analyses in Minitab, as well as about the assumptions that must be made in each case, can be found in the relevant lessons.
28.3 - Two Continuous Measurements
28.3 - Two Continuous MeasurementsOne Group with Two Continuous Measurements
If we have two continuous measurements, we could consider either of two possible analyses, namely:
- Correlation
- Linear regression
Correlation helps to answer the research question "does a linear relationship exist between two continuous random variables?" Linear regression, on the other hand, helps to answer the research question "what is the linear relationship between a fixed predictor and a random variable?" In Minitab, we use the following commands:
- Stat >> Basic Statistics >> Correlation... to conduct a correlation analysis
- Stat >> Regression >> Regression... to conduct a linear regression analysis
Example 28-10
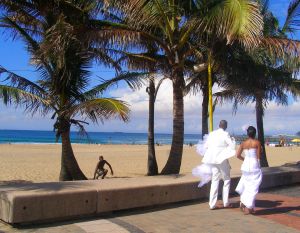
Does a (linear) relationship exist between a husband's and wife's height?
Answer
Because we are only interested in learning whether a linear relationship exists between husbands' and wives' heights, and not the nature of the relationship, we would want to conduct a correlation analysis. We can use Minitab's Stat >> Basic Statistics >> Correlation... command to test the null hypothesis:
\(H_0 : \rho = 0\)
against the alternative hypothesis:
\(H_A : \rho \ne 0\)
Example 28-11
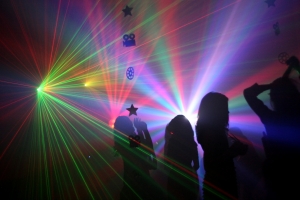
If a randomly selected college student goes out to party ten times each month, what kind of grade point average (GPA) can he or she expect?
Answer
If x denotes the number of times a randomly selected college student goes out to party in one month, and y = the student's grade point average, then we'd be interested in estimating the slope and intercept parameters in the linear regression equation:
\(\mu_y=\alpha + \beta x\)
Of course, that's assuming that the relationship is indeed a linear relationship, but that could be verified when doing the analysis. We could use Minitab's Stat >> Regression >> Regression... command to help complete the analysis.
28.4 - Practice
28.4 - PracticeWe've pretty much reviewed now all of the analysis methods we've learned in this course, as well as when it would be appropriate to use each analysis method. In summary:
- First, ask what type of response has been measured. Do we summarize it by a proportion or a mean (median)?
- Then, ask how many groups are being studied and/or compared.
- Then, decide whether we should conduct a hypothesis test or calculate an interval estimate.
- And, of course, always check that the method's assumptions and/or conditions are met.
Try it!
For each of the following research questions, identify at least one analysis that would be appropriate for the situation.
-
Do seniors earn higher semester grade point averages than freshmen?
Answer
We have two groups, seniors and freshmen. The response is continuous. We are only interested in determining whether or not a difference between the two groups exists. Therefore, conduct a two-sample t-test for testing the difference in the mean GPA for seniors and the mean GPA for freshmen.
-
What is the relationship between the amount of alcohol consumed (in ounces) and the level of coordination (on a scale from 1 to 10)?
Answer
We have two continuous measurements, for which we are interested in quantifying the nature of the relationship. Therefore, conduct a linear regression analysis so that we can estimate:
\(\mu_y=\alpha + \beta x\)
where x denotes the amount of alcohol consumed and the level of coordination is the response y.
-
Are SAT scores and grade point averages linearly related?
Answer
We have two continuous measurements, for which we are interested in determining whether or not a linear relationship exists. Therefore, conduct a correlation analysis to test the null hypothesis:
\(H_0 : \rho = 0\)
against the alternative hypothesis:
\(H_A : \rho \ne 0\)
-
Is there a difference in the percentage of NCAA basketball players who graduate and NCAA football players who graduate?
Answer
The response is binary (graduate or not), and there are two groups being compared (NCAA basketball players and NCAA football players). Therefore, conduct a Z-test for comparing two proportions.
-
How many hours per week do PSU students study outside of class?
Answer
We have a continuous response variable (number of hours per week studied) and one group (PSU students). Therefore, calculate a one-sample t-interval for the mean \(\mu\).
-
How much more prevalent is lupus in women than in men?
Answer
We have a binary response (lupus or not), and two groups (men and women). Therefore, calculate a Z-interval for the difference in the two proportions.
-
Do PSU students drink, on average, more than one cup of coffee per day during finals week? (During finals week, a sample of students will record how many cups of coffee they drink each day.)
Answer
We have a continuous response variable (number of cups of coffee consumed per day during finals week) and one group (PSU students). Therefore, conduct a one-sample t-test for testing \(H_0: \mu = 1\) against \(H_A: \mu > 1\).
-
Is the recovery time from a migraine headache related to the treatment (A, B, C)?
Answer
We have a continuous response variable (recovery time) and three groups (A, B, C). Therefore, conduct a one-factor analysis of variance for testing \(H_0: \mu_A = \mu_B = \mu_C\) against \(H_A:\) not all \(\mu_i\) are equal.
-
Is there a relationship between political affilition (Democrat, Republican, Independent) and income level (Poor, Middle Class, Wealthy)?
Answer
We have two categorical variables for which we are interested in determining whether or not a relationship exists. Therefore, conduct a chi-square test.
-
How much heavier (in pounds) are 15-year-old boys than 13-year-old boys?
Answer
We have a continuous response (weight in pounds) and two independent groups (13-year-old and 15-year-old boys). Therefore, calculate a two-sample t-interval for the difference in the two means.
-
A random sample of 64 students were asked "do you study regularly at Pattee Library?"
Answer
We have a binary response (yes or no) and one group (students). Therefore, calculate a confidence interval for the proportion of students who study regularly at Pattee.
-
Do Goodyear tires have better tread wear than Firestone tires? Tread wear is measured in millimeters of tread remaining after 30,000 miles. Thirty cars are selected for an experiment. On each car, one Goodyear tire and one Firestone tire is placed randomly in one of two front positions.
Answer
We have a continuous response (tread wear) and two paired groups (Firestone and Goodyear tires). Therefore, conduct a paired t-test for testing whether the mean difference is 0.