10: Log-Linear Models
10: Log-Linear ModelsOverview
Thus far in the course, we have alluded to log-linear models several times but have never got down to the specifics of them. When we dealt with inter-relationships among several categorical variables, our focus was mostly on describing their associations via
- single summary statistics and
- significance testing.
Log-linear models go beyond single summary statistics and specify how the cell counts depend on the levels of categorical variables. They model the association and interaction patterns among categorical variables. The log-linear model is natural for Poisson, Multinomial and Product-Multinomial sampling. They are appropriate when there is no clear distinction between response and explanatory variables or when there are more than two responses. This is a fundamental difference between logistic models and log-linear models. In the former, a response is identified, but no such special status is assigned to any variable in log-linear modeling. By default, log-linear models assume discrete variables to be nominal, but these models can be adjusted to deal with ordinal and matched data. Log-linear models are more general than logit models, but some log-linear models have direct correspondence to logit models.
Consider the Berkeley admission example. We may consider all possible relationships among A = Admission, D = Department and S = Sex. Alternatively, we may consider A as response and D and S as covariates, in which case the possible logit models are
- logit model for A with only an intercept;
- logit model for A with a main effect for D;
- logit model for A with a main effect for S;
- logit model for A with a main effects for D and S; and
- logit model for A with main effects for D and S and the D \(\times\) S interaction.
Corresponding to each of the above, a log-linear model may be defined. The notations below follow those of Lesson 5.
- Model of joint independence (DS, A), which indicates neither D nor S has an effect on A is equivalent to a logit model for response A with only an intercept;
- Model of conditional independence (DS, DA), which indicates that S has no effect on A after the effect of D is included, is equivalent to a logit model for response A with the single predictor D; another conditional independence model (DS, SA) is equivalent to a logit model for response A with the single predictor S;
- Model of homogeneous association (DS, DA, SA) indicates that the effect of S on A is the same at each level of department, which is equivalent to a logit model for response A with predictors D and S but no interaction; and
- Saturated or unrestricted model (DSA) indicates that the effect of S on A varies across D and is equivalent to a logit model for response A with predictors D and S as well as the D by S interaction.
"Equivalent" means that two models give equivalent goodness-of-fit statistics relative to a saturated model, and equivalent expected counts for each cell. Log-linear models are not the same as logit models, because the log-linear models describe the joint distribution of all three variables, whereas the logit models describe only the conditional distribution of A given D and S. Log-linear models have more parameters than the logit models, but the parameters corresponding to the joint distribution of D and S are not of interest.
In general, to construct a log-linear model that is equivalent to a logit model, we need to include all possible associations among the predictors. In the Berkeley example, we need to include DS in every model. This lesson will walk through examples of how this is done in both SAS and R.
In subsequent sections, we look at the log-linear models in more detail. The two great advantages of log-linear models are that they are flexible and they are interpretable. Log-linear models have all the flexibility associated with ANOVA and regression. We have mentioned before that log-linear models are also another form of GLM. They also have natural interpretations in terms of odds and frequently have interpretations in terms of independence, as we have shown above.
Lesson 10 Code Files
10.1 - Log-Linear Models for Two-way Tables
10.1 - Log-Linear Models for Two-way TablesOverview
Recall, that a two-way ANOVA models the expected value of a continuous variable (e.g., plant length) depending on the levels of two categorical variables (e.g., low/high sunlight and low/high water amount). In contrast, the log-linear model expresses the cell counts (e.g., the number of plants in a cell) depending on the levels of two categorical variables.
Let \(\mu_{ij}\) be the expected counts, \(E(n_{ij})\), in an \(I \times J\) table, created by two random variables \(A\) and \(B\).
Objective
Model the cell counts: \(\mu_{ij} = n\pi_{ij}\)
Model structure
An analogous saturated log-linear model to two-way ANOVA with interaction is
\(\log(\mu_{ij})=\lambda+\lambda_i^A+\lambda_j^B+\lambda_{ij}^{AB}\)
where \(i = 1,\ldots, I, j = 1, \ldots, J\), are levels of categorical random variables \(A\) and \(B\), with constraints: \(\sum_i \lambda_i = \sum_j \lambda_j = \sum_i \sum_j \lambda_{ij} = 0\), to deal with overparametrization. Overparametrization means that the number of parameters is more than what can be uniquely estimated. This model is over-parametrized because term \(\lambda_{ij}\) already has \(I \times J\) parameters corresponding to the cell means \(\mu_{ij}\). The constant, \(\lambda\), and the "main effects", \(\lambda_i\) and \(\lambda_j\) give us additional \(1 + I + J\) parameters. Superscripts denote variables \(A\) and \(B\). We will see more on this in the next sections.
Model Assumptions
The \(N = I \times J\) counts in the cells are assumed to be independent observations from a Poisson random variable, \(n_{ij}\sim \text{Poisson}(\mu_{ij})\). The log-linear modeling is natural for Poisson, Multinomial and Product-Multinomial sampling like we have discussed in earlier lectures.
Recall the Vitamin C study, a \(2 \times 2\) example from Lesson 3. Are the type of treatment and contracting cold independent? If there are associated, in which way are they associated?
We already know how to answer the above questions via the chi-square test of independence, but now we want to model the cell counts with the log-linear model of independence and ask if this model fits well.
Log-linear Models for Two-Way Tables
Given two categorical random variables, \(A\) and \(B\), there are two main types of models we will consider:
- Independence model (A, B)
- Saturated model (AB)
Let us start with the model of independence.
10.1.1 - Model of Independence
10.1.1 - Model of IndependenceRecall, that the independence can be stated in terms of cell probabilities as a product of marginal probabilities,
\(\pi_{ij}=\pi_{i+}\pi_{+j}\quad i = 1, \ldots, I, j = 1, \ldots, J\)
and in terms of cell frequencies,
\(\mu_{ij}=n\pi_{ij}=n\pi_{i+}\pi_{+j}\quad i = 1, \ldots, I, j = 1, \ldots, J\)
By taking natural logarithms on both sides of "=" sign, we obtain the loglinear model of independence:
\(\log(\mu_{ij}) = \lambda+\lambda_i^A+\lambda_j^B\)
where superscripts A and B are just used to denote the two categorical variables.
This is an ANOVA type-representation where:
- \(\lambda\) represents the "overall" effect, or the grand mean of the logarithms of the expected counts, and it ensures that \(\sum_i \sum_j \mu_{ij} = n\), that is, the expected cell counts under the fitted model add up to the total sample size \(n\).
- \(\lambda^A_I\) represents the "main" effect of variable A, or a deviation from the grand mean, and it ensures that \(\sum_j \mu_{ij} = n_{i+}\), that is, the marginal totals under the fitted model add up to the observed marginal counts. It represents the effect of classification in row \(i\).
- \(\lambda^B_J\) represents the "main" effect of variable B, or a deviation from the grand mean, and it ensures that \(\sum_i \mu_{ij} = n+j\). This is the effect of classification in column j.
- \(\lambda^A_I=\lambda^B_J=0\), or alternatively, \(\sum_i \lambda^{A}_{i} = \sum_j \lambda^{B}_{j} = 0\), to deal with over-parametrization (see below).
The maximum likelihood (ML) fitted values for the cell counts are the same as the expected (fitted) values under the test of independence in two-way tables, i.e., \(E(\mu_{ij}) = n_{i+}n_{+j}/n\). Thus, the \(X^2\) and \(G^2\) for the test of independence are goodness-of-fit statistics for the log-linear model of independence testing that the independence model holds versus that it does not, or more specifically testing that the independence model is true vs. saturated model is true. This model also implies that ALL odds ratios should be equal to 1.
Parameter Constraints & Uniqueness
For an \(I\times J\) table, and the model is
\(\log(\mu_{ij})=\lambda+\lambda_i^A+\lambda_j^B\)
There are \(I\) terms in the set {\(\lambda^A_I\)}, but one of them is redundant, so there are \(I − 1\) unknown parameters, e.g., {\(\lambda_{1}^{A}, \ldots , \lambda_{I-1}^{A}\)}, and there are \(J\) terms in the set {\(\lambda^B_J\)}, and one is redundant, so there are \(J − 1\) unknown parameters, e.g., {\(\lambda_1^B , \ldots, \lambda_{J-1}^B\)}. (Why is one of them redundant?) There can be many different parameterizations, but regardless of which set we use, we need to set the constraints to account for redundant parameters. Nonexistence of a unique set of parameters does not mean that the expected cell counts will change depending on which set of parameters is being used. It simply means that the estimates of the effects may be obtained under different sets of constraints, which will lead to different interpretations. But expected cell counts will remain the same.
DUMMY CODING: To avoid over-parametrization, one member in the set of \(\lambda\)s is fixed to have a constant value, typically 0. This corresponds to using dummy coding for the categorical variables (e.g. A = 1, 0). By default, in SAS PROC GENMOD, the last level is set to 0. So, we have
\(\log(\mu_{11})=\lambda+\lambda_1^A+\lambda_1^B\)
\(\log(\mu_{22})=\lambda+0+0=\lambda\)
By default, in R glm() the first level of the categorical variable is set to 0. So, we have
\(\log(\mu_{11})=\lambda+0+0=\lambda\)
\(\log(\mu_{22})=\lambda+\lambda_2^A+\lambda_2^B\)
ANOVA-type CODING: Another way to avoid over-parametrization is to fix the sum of the terms equal to a constant, typically 0. That is the ANOVA-type constraint. This corresponds to using the so-called "effect” coding for categorical variables (e.g. A = 1, 0, −1). By default, SAS PROC CATMOD and R loglin(), use the zero-sum constraint, e.g., the expected cell count in the first cell and the last cell,
\(\log(\mu_{11})=\lambda+\lambda_1^A+\lambda_1^B\)
\(\log(\mu_{22})=\lambda-\lambda_1^A-\lambda_1^B\)
We will see more on these with a specific example in the next section.
Link to odds and odds ratio
We can have different parameter estimates (i.e, different values of \(\lambda\)s) depending on the type of constraints we set. So, what is unique about these parameters that lead to the same inference, regardless of parametrization? The differences, that is the log odds, are unique:
\(\lambda_i^A-\lambda_{i'}^A\)
\(\lambda_j^B-\lambda_{j'}^B\)
where the subscript \(i\) denotes one level of categorical variable \(A\) and "\(i\)" denotes another level of the same variable; similarly for \(B\).
Thus the odds is also unique!
\begin{align} \log(odds) &= \log\left(\dfrac{\mu_{i1}}{\mu_{i2}}\right)=\log(\mu_{i1})-\log(\mu_{i2})\\ &= (\lambda+\lambda_i^A+\lambda_1^B)-(\lambda+\lambda_i^A+\lambda_2^B)=\lambda_1^B-\lambda_2^B\\ \end{align}
\(\Rightarrow \mbox{odds} = \exp(\lambda_{1}^{B} − \lambda_{2}^{B})\)
If we have the model of independence, we expect that the log(odds ratio) is 0, that is, that the odds ratio is 1. Can you finish the calculation below and show that you get zero?
\begin{align}\log(oddsratio) &= \log\left(\dfrac{\mu_{11}\mu_{22}}{\mu_{12}\mu_{21}}\right)\\&= \log(\mu_{11})+\log(\mu_{22})-\log(\mu_{12})-\log(\mu_{21})\\&= \cdots\\\end{align}
\(\begin{align*}\log(oddsratio)& = \log\left(\dfrac{\mu_{11}\mu_{22}}{\mu_{12}\mu_{21}}\right)\\ &= \log(\mu_{11})+\log(\mu_{22})-\log(\mu_{12})+\log(\mu_{21})\\ &= \lambda+\lambda_1^A+\lambda_1^B+\lambda+\lambda_2^A+\lambda_2^B-\lambda-\lambda_1^A-\lambda_2^B-\lambda-\lambda_2^A-\lambda_1^B\\ &= 0\\ \end{align*}\)
The odds ratio measures the strength of the association and depends only on the interaction terms {\(\lambda_{ij}^{AB}\) }, which clearly does not appear in this model, but we will discuss it when we see the saturated log-linear model.
Do you recall, how many odds ratios do we need to completely characterize associations in \(I\times J\) tables?
10.1.2 - Example: Therapeutic Value of Vitamin C
10.1.2 - Example: Therapeutic Value of Vitamin CExample: Vitamin C Study - Revisited
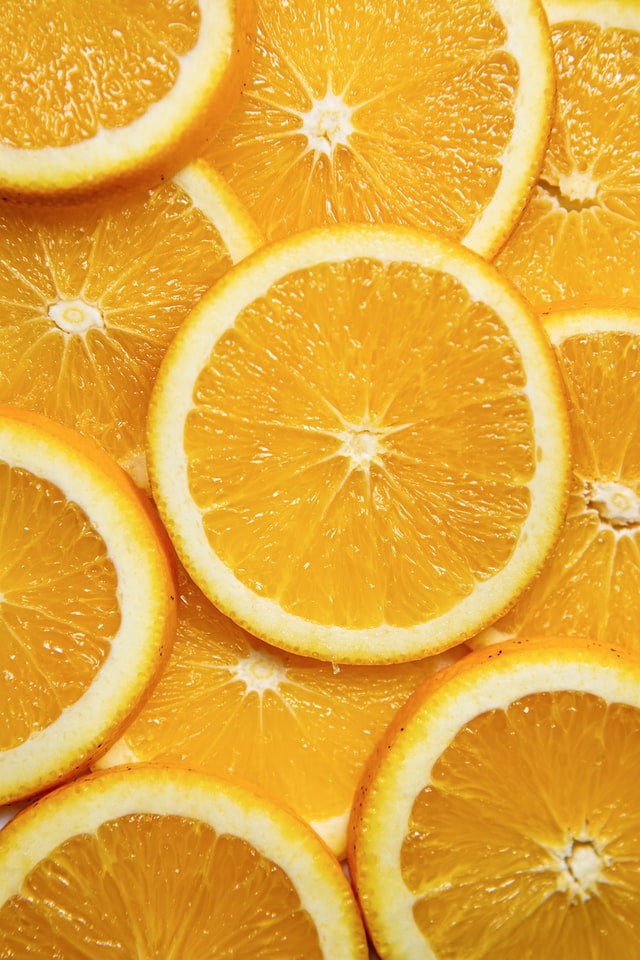
Recall the Vitamin C study, a \(2 \times 2\) example from Lesson 3. From the earlier analysis, we learned that the treatment and response for this data are not independent:
\(G^2 = 4.87\), df = 1, p−value = 0.023,
And from the estimated odds ratio of 0.49, we noted that the odds of getting a cold were about twice as high for those who took the placebo, compared with those who took vitamin C. Next, we will consider the same question by fitting the log-linear model of independence.
There are (at least) two ways of fitting these models in SAS and R. First, we will see the specification for two procedures in SAS (PROC CATMOD and PROC GENMOD); see VitaminCLoglin.sas and VitaminCLoglin.R.
The PROC CATMOD procedure
INPUT:
proc catmod data=ski order=data;
weight count;
model treatment*response=_response_;
loglin treatment response;
run;
The MODEL statement (line) specifies that we are fitting the model on two variables treatment and response specified on the left-hand side of the MODEL statement. The MODEL statement only takes independent variables on its right-hand side. In the case of fitting a log-linear model, we need to use a keyword _response_ on the right-hand side.
The LOGLIN statement specifies that it's a model of independence because we are stating that the model has only main effects, one for the "treatment" variable and the other for the "response" variable, in this case.
OUTPUT:
Population Profiles and Response profiles
The responses we're modeling are four cell counts:
Population Profiles | |
---|---|
Sample | Sample Size |
1 | 279 |
Response Profiles | ||
---|---|---|
Response | treatment | response |
1 | placebo | cold |
2 | placebo | nocold |
3 | absorbic | cold |
4 | absorbic | nocold |
Maximum Likelihood Analysis of Variance
As with ANOVA, we are breaking down the variability across factors. More specifically, the main effect TREATMENT tests if the subjects are evenly distributed over the two levels of this variable. The null hypothesis assumes that they are, and the alternative assumes that they are not. Based on the output below, are the subjects evenly distributed across the placebo and the vitamin C conditions?
Maximum Likelihood Analysis of Variance | |||
---|---|---|---|
Source | DF | Chi-Square | Pr > ChiSq |
treatment | 1 | 0.00 | 0.9523 |
response | 1 | 98.12 | <.0001 |
Likelihood Ratio | 1 | 4.87 | 0.0273 |
The main effect RESPONSE tests if the subjects are evenly distributed over the two levels of this variable. The Wald statistic \(X^2=98.12\) with df = 1 indicates highly uneven distribution.
The LIKELIHOOD RATIO (or the deviance statistic) tells us about the overall fit of the model. Does this value look familiar? Does the model of independence fit well?
Analysis of Maximum Likelihood Estimates
Gives the estimates of the parameters. We focus on the estimation of odds.
Analysis of Maximum Likelihood Estimates | |||||
---|---|---|---|---|---|
Parameter | Estimate | Standard | Chi- | Pr > ChiSq | |
treatment | placebo | 0.00358 | 0.0599 | 0.00 | 0.9523 |
response | cold | -0.7856 | 0.0793 | 98.12 | <.0001 |
For example,
\(\lambda_1^A=\lambda_{placebo}^{TREATMENT}=0.00358=-\lambda_{ascobic}^{TREATMENT}=-\lambda_2^A\)
What are the odds of getting cold? Recall that PROC CATMOD, by default, uses zero-sum constraints. Based on this output the log odds are
\begin{align}
\text{log}(\mu_{i1}/\mu_{i2}) &=\text{log}(\mu_{i1})-\text{log}(\mu_{i2})\\
&=[\lambda+\lambda_{placebo}^{TREATMENT}+\lambda_{cold}^{RESPONSE}]-[\lambda+\lambda_{placebo}^{TREATMENT}+\lambda_{nocold}^{RESPONSE}]\\
&=\lambda_{cold}^{RESPONSE}-\lambda_{nocold}^{RESPONSE}\\
&=\lambda_{cold}^{RESPONSE}+\lambda_{cold}^{RESPONSE}\\
&=-0.7856-0.7856=-1.5712\\
\end{align}So the odds are \(\exp(-1.5712) = 0.208\). Notice that the odds are the same for all rows.
Think about it!
What are the odds of being in the placebo group? Note that this question is not really relevant here, but it's a good exercise to figure out the model parameterization and how we come up with estimates of the odds. Hint: log(odds)=0.00716.
The PROC GENMOD procedure
Fits a log-linear model as a Generalized Linear Model (GLM) and by default uses indicator variable coding. We will mostly use PROC GENMOD in this course.
INPUT:
proc genmod data=ski order=data;
class treatment response;
model count = response*treatment /link=log dist=poisson lrci type3 obstats;
run;
The CLASS statement (line) specifies that we are dealing with two categorical variables: treatment and response.
The MODEL statement (line) specifies that our responses are the cell counts, and link=log specifies that we are modeling the log of counts. The random distribution is Poisson, and the remaining specifications ask for different parts of the output to be printed.
OUTPUT:
Model Information
Gives assumptions about the model. In our case, tells us that we are modeling the log, i.e., the LINK FUNCTION, of the responses, i.e. DEPENDENT VARIABLE, that is counts which have a Poisson distribution.
Model Information | |
---|---|
Data Set | WORK.SKI |
Distribution | Poisson |
Link Function | Log |
Dependent Variable | count |
Number of Observations Read | 4 |
---|---|
Number of Observations Used | 4 |
Class Level Information
Information on the categorical variables in our model
Class Level Information | ||
---|---|---|
Class | Levels | Values |
treatment | 2 | placebo absorbic |
response | 2 | cold nocold |
Criteria For Assessing Goodness of Fit
Gives the information on the convergence of the algorithm for MLE for the parameters, and how well the model fits. What is the value of the deviance statistic \(G^2\)? What about the Pearson \(X^2\)? Note that they are the same as what we got when we did the chi-square test of independence; we will discuss "scaled" options later with the concept of overdispersion. Note also the value of the log-likelihood for the fitted model, which in this case is independence.
Criteria For Assessing Goodness Of Fit | |||
---|---|---|---|
Criterion | DF | Value | Value/DF |
Deviance | 1 | 4.8717 | 4.8717 |
Scaled Deviance | 1 | 4.8717 | 4.8717 |
Pearson Chi-Square | 1 | 4.8114 | 4.8114 |
Scaled Pearson X2 | 1 | 4.8114 | 4.8114 |
Log Likelihood | 970.6299 |
Analysis of Parameter Estimates
Gives individual parameter estimates. Recall that PROC GENMOD does not use zero-sum constraints but fixes typically the last level of each variable to be equal to zero:
\(\hat{\lambda}=4.75,\ \hat{\lambda}^A_1=0.0072,\ \hat{\lambda}^A_2=0,\ \hat{\lambda}^B_1=-1.5712,\ \hat{\lambda}^B_2=0\)
Analysis Of Maximum Likelihood Parameter Estimates | ||||||||
---|---|---|---|---|---|---|---|---|
Parameter | DF | Estimate | Standard | Likelihood Ratio 95% Confidence Limits | Wald Chi-Square | Pr > ChiSq | ||
Intercept | 1 | 4.7457 | 0.0891 | 4.5663 | 4.9158 | 2836.81 | <.0001 | |
treatment | placebo | 1 | 0.0072 | 0.1197 | -0.2277 | 0.2422 | 0.00 | 0.9523 |
treatment | absorbic | 0 | 0.0000 | 0.0000 | 0.0000 | 0.0000 | . | . |
response | cold | 1 | -1.5712 | 0.1586 | -1.8934 | -1.2702 | 98.11 | <.0001 |
response | nocold | 0 | 0.0000 | 0.0000 | 0.0000 | 0.0000 | . | . |
Scale | 0 | 1.0000 | 0.0000 | 1.0000 | 1.0000 |
The scale parameter was held fixed.
So, we can compute the estimated cell counts for having cold given vitamin C based on this model:
\(\mu_{21}=\exp(\lambda+\lambda_2^A+\lambda_1^B)=\exp(4.745+0-1.5712)=23.91 \)
What about the other cells: \(\mu_{11}\), \(\mu_{12}\), and \(\mu_{22}\)?
What are the estimated odds of getting a cold?
\(\exp(\lambda_1^B-\lambda_2^B)=\exp(-1.571-0)=0.208\)
What about the odds ratio?
LR Statistics for Type 3 Analysis
Testing the main effects is similar to the Maximum Likelihood Analysis of Variance in the CATMOD, except this is the likelihood ratio (LR) statistic and not the Wald statistic. When the sample size is moderate or small, the likelihood ratio statistic is the better choice. From the CATMOD output, the chi-square Wald statistic was 98.12, with df=1, and we reject the null hypothesis. Here, we reach the same conclusion, except, that LR statistic is 130.59 with df=1.
LR Statistics For Type 3 Analysis | |||
---|---|---|---|
Source | DF | Chi-Square | Pr > ChiSq |
treatment | 1 | 0.00 | 0.9523 |
response | 1 | 130.59 | <.0001 |
Observation Statistics
Gives information on expected cell counts and five different residuals for further assessment of the model fit (i.e., "Resraw"=observed count - predicted (expected) count, "Reschi"=pearson residual, "Resdev"= deviance residuals, "StReschi"=standardized pearson residuals, etc.)
Observation Statistics | |||||||||||||||||||||||
---|---|---|---|---|---|---|---|---|---|---|---|---|---|---|---|---|---|---|---|---|---|---|---|
Observation | count | treatment | response | Predicted Value | Linear Predictor | Standard Error of the Linear Predictor | HessWgt | Lower | Upper | Raw Residual | Pearson Residual | Deviance Residual | Std Deviance Residual | Std Pearson Residual | Likelihood Residual | Leverage | CookD | DFBETA_Intercept | DFBETA_treatmentplacebo | DFBETA_responsecold | DFBETAS_Intercept | DFBETAS_treatmentplacebo | DFBETAS_responsecold |
1 | 31 | placebo | cold | 24.086031 | 3.1816321 | 0.1561792 | 24.086031 | 17.734757 | 32.711861 | 6.9139686 | 1.4087852 | 1.3483626 | 2.0994112 | 2.1934897 | 2.1551805 | 0.5875053 | 2.2842489 | -0.060077 | 0.1197239 | 0.3491947 | -0.674251 | 0.9998856 | 2.2013658 |
2 | 109 | placebo | nocold | 115.91398 | 4.7528483 | 0.0888123 | 115.91398 | 97.395437 | 137.95359 | -6.913978 | -0.642185 | -0.648733 | -2.215859 | -2.193493 | -2.195419 | 0.9142868 | 17.107471 | -0.060077 | -0.576172 | 0.3491952 | -0.674252 | -4.811954 | 2.2013689 |
3 | 17 | absorbic | cold | 23.913989 | 3.1744636 | 0.1563437 | 23.913989 | 17.602407 | 32.488674 | -6.913989 | -1.413848 | -1.491801 | -2.314435 | -2.193496 | -2.244533 | 0.5845377 | 2.2564902 | -0.060077 | 0.1197243 | -0.346701 | -0.674253 | 0.9998885 | -2.185648 |
4 | 122 | absorbic | nocold | 115.08602 | 4.7456799 | 0.0891012 | 115.08602 | 96.645029 | 137.04577 | 6.913978 | 0.6444908 | 0.638194 | 2.172062 | 2.1934927 | 2.1916509 | 0.9136701 | 16.973819 | 0.6358195 | -0.576172 | -0.346701 | 7.1359271 | -4.811954 | -2.185645 |
The LOGLIN and GLM functions in R
To do the same analysis in R, the loglin()
function corresponds roughly to PROC CATMOD, and glm()
function corresponds roughly to PROC GENMOD. See VitaminCLoglin.R for the commands and to generate the output.
Here is a brief comparison to loglin() and glm() output with respect to model estimates.
The following command fits the log-linear model of independence, and from the part of the output that gives parameter estimates, we see that they correspond to the output from CATMOD. For example, the odds of getting a cold are estimated to be
\(\exp(\lambda_{cold}^{response} - \lambda_{no cold}^{response})=\exp(-0.7856-0.7856)=\exp(-1.5712)=0.208\)
loglin(ski, list(1, 2), fit=TRUE, param=TRUE)
$param$"(Intercept)"
[1] 3.963656
$param$Treatment
Placebo VitaminC
0.003584245 -0.003584245
$param$Cold
Cold NoCold
-0.7856083 0.7856083
The following command fits the log-linear model of independence using glm()
, and from the part of the output that gives parameter estimates, we see that they correspond to the output from GENMOD but with the signs reversed, i.e., odds of \(nocold=\exp(\lambda_{nocold}^{response})=\exp(1.5712)\). Thus, the odds of cold are \(\exp(-1.5712)\).
glm(ski.data$Freq~ski.data$Treatment+ski.data$Cold, family=poisson())
Coefficients:
Estimate Std. Error z value Pr(>|z|)
(Intercept) 3.181632 0.156179 20.372 <2e-16 ***
ski.data$TreatmentVitaminC -0.007168 0.119738 -0.060 0.952
ski.data$ColdNoCold 1.571217 0.158626 9.905 <2e-16 ***
Assessing Goodness of Fit
The ANOVA function gives information on how well the model fits. The value of the deviance statistic is \(G^2=4.872\) with 1 degree of freedom, which is significant evidence to reject this model. Note that this is the same as what we got when we did the chi-square test of independence; we will discuss options for dealing with overdispersion later as well.
> ski.ind
Call: glm(formula = ski.data$Freq ~ ski.data$Treatment + ski.data$Cold, family = poisson())
Coefficients:
(Intercept) ski.data$TreatmentVitaminC ski.data$ColdNoCold
3.181632 -0.007168 1.571217
Degrees of Freedom: 3 Total (i.e. Null); 1 Residual
Null Deviance: 135.5
Residual Deviance: 4.872 AIC: 34
10.1.3 - Saturated Model
10.1.3 - Saturated ModelWith the saturated model, the \(N = IJ\) counts in the cells are still assumed to be independent observations of a Poisson random variable, but no independence is assumed between the variables \(A\) and \(B\). The model is expressed as
\(\log(\mu_{ij})=\lambda+\lambda_i^A+\lambda_j^B+\lambda_{ij}^{AB}\)
Note the additional \(\lambda_{ij}^{AB}\) interaction term, compared with the independence model. We still have the same ANOVA-like constraints to avoid over-parameterization, and again, this changes with the software used. Ultimately, the inference, in the end, will not depend on the choice of constraints.
Parameter estimates and interpretation
The odds ratio is directly related to the interaction terms. For example, for a \(2 \times 2\) table,
\begin{align}
\log(\theta) &= \log\left(\dfrac{\mu_{11}\mu_{22}}{\mu_{12}\mu_{21}}\right)\\
&= \\
&= \lambda_{11}^{AB}+\lambda_{22}^{AB}-\lambda_{12}^{AB}-\lambda_{21}^{AB}
\end{align}
\(\begin{align*}\log(\theta)& = \log\left(\dfrac{\mu_{11}\mu_{22}}{\mu_{12}\mu_{21}}\right)\\ &= \lambda+\lambda_1^A+\lambda_1^B+\lambda_{11}^{AB}+\lambda+\lambda_2^A+\lambda_2^B+\lambda_{22}^{AB}-\lambda-\lambda_1^A-\lambda_2^B-\lambda_{12}^{AB}-\lambda-\lambda_2^A-\lambda_1^B-\lambda_{21}^{AB}\\ &= \lambda_{11}^{AB}+\lambda_{22}^{AB}-\lambda_{12}^{AB}-\lambda_{21}^{AB}\\ \end{align*}\)
How many odds ratios are there? There should be \((I-1) (J-1)\) which should be equal to the unique number of \(\lambda_{ij}\)s in the model.
How many unique parameters are there in this model?
Term | # of terms | #of constraints | # of unique parameters |
---|---|---|---|
\(\lambda\) | \(1\) | \(0\) | \(1\) |
\(\lambda_i^A\) | \(I\) | \(1\) | \(I - 1\) |
\(\lambda_i^B\) | \(J\) | \(1\) | \(J - 1\) |
\(\lambda_{ij}^{AB}\) | \(I J\) | \(I + J - 1\) | \((I - 1) (J - 1)\) |
With \(I J = N\) terms, the model is a perfect fit! Moreover, the saturated model includes the independence model as a special case, where the interaction terms are 0.
Example: Vitamin C
Let's evaluate the CATMOD and GENMOD output for VitaminCLoglin.sas and R code for VitaminCLoglin.R.
To fit the saturated models, we need to specify or add an interaction term in our code.
In CATMOD:
proc catmod data=ski order=data;
weight count;
model treatment*response=_response_;
loglin treatment|response;
run;
The LOGLIN statement (line) now specifies the saturated model. treatment|response specification adds an interaction term to the main effects model.
In GENMOD:
proc genmod data=ski order=data;
class treatment response;
model count = response*treatment /link=log dist=poisson lrci type3 obstats;
run;
The "*" sign in the response*treatment, indicates that we want the interaction term and ALL lower order terms; that is, the saturated model.
Notice, that we can also specify the saturated model by explicitly entering the main effects in the model statement:
model count = response treatment response*treatment/link=log dist=poisson lrci type3 obstats;
In these two model specifications under GENMOD, we are fitting different numbers of parameters, and the output sections on LR Statistics For Type 3 Analysis will be different, but the estimates of odds and odds-ratios will be the same. For the output, we have the same parts as we did for the independence model, except now we have more parameter estimates. We will consider the latter GENMOD parametrization and compare it to that for CATMOD.
So, what are the odds of getting cold given that a person took a placebo pill? Let's first start with log-odds:
\(\log(\mu_{11}/\mu_{12})=\log(\mu_{11})-\log(\mu_{12})=[\lambda+\lambda_1^A+\lambda_1^B+\lambda_{11}^{AB}]-[\lambda+\lambda_1^A+\lambda_2^B+\lambda_{12}^{AB}]\)
Based on GENMOD parameterization: \(\lambda_1^B + \lambda_{11}^{AB} - \lambda_2^B -\lambda_{12}^{AB} = -1.9708 + 0.7134 - 0 - 0\), and the odds are \(\exp(-1.2574) = 0.2844\).
Based on CATMOD parameterization: \([\lambda+\lambda_1^A + \lambda_1^B+\lambda_{11}^{AB} ]- [\lambda+\lambda_1^A- \lambda_1^B - \lambda_{11}^{AB}] = 2\lambda_1^B+2\lambda_{11}^{AB} = 2(-0.807 + 0.1784)\), and the odds are \(\exp(\log \mbox{odds}) = 0.2844\).
In R, the syntax for loglin()
would be
loglin(ski, list(c(1, 2)), fit=TRUE, param=TRUE)
And the syntax for glm()
would be
glm(Freq~Treatment*Cold, family=poisson(), data=ski.data)
loglin()
? What about GENMOD or glm()
?
For example, from the second way of fitting the model with GENMOD, the odds ratio is \(\exp(0.7134)=2.041\), with 95% CI \((\exp(0.079), \exp(1.3770)\). This corresponds to \(1/2.041=0.49\), which we saw before.
treatment*response placebo cold 1 0.7134 0.3293 0.0790 1.3770
From R, we get the same:
> summary(ski.sat)
...
TreatmentVitaminC:ColdNoCold 0.7134 0.3293 2.166 0.0303 *
Q2: Is the interaction of treatment and response significant (hint: look at LR Statistics for Type 3 Analysis). Where does df = 3 come from?
Q3: Model fits perfectly. Let's do some model selection. Compare the independence and saturated model, by calculating the LR statistics (e.g. difference in deviances?
\(2\times (973.0657-970.629)\approx 4.8717\)
Q4: How many df does this statistic have?
Hierarchical Models
These models include all lower-order terms that comprise higher-order terms in the model.
(A, B) is a simpler model than (AB)
Interpretation does not depend on how the variables are coded.
\(\log(\mu_{ij})=\lambda+\lambda_i^A+ \lambda_{ij}^{AB}\)
No, it is not a hierarchical model. It is missing \(\lambda^{B}_{j}\).
We want hierarchical models so that interaction terms represent associations. If there is a significant interaction term, we do NOT look at the lower-order terms, but only interpret the higher-order terms because the value of lower-order terms is coding dependent and can be misleading.
Next, we'll see how these models extend to three-way tables.
10.2 - Log-linear Models for Three-way Tables
10.2 - Log-linear Models for Three-way TablesIn this section we will extend the concepts we learned about log-linear models for two-way tables to three-way tables. We will learn how to fit various models of independence discussed in Lesson 5 (e.g., conditional independence, joint independence, etc.) and will see additional statistics, besides the usual \(X^2\) and \(G^2\), to assess the model fit and to choose the "best" model.
The notation for log-linear models extends to three-way tables as follows. If \(\mu_{ijk}\) represents the mean for the \(i\)th, \(j\)th, and \(k\)th levels of variables \(A\), \(B\), and \(C\), respectively, then we write
\(\log(\mu_{ijk})=\lambda+\lambda_i^A+\lambda_j^B+\lambda_k^C+\lambda_{ij}^{AB}+\lambda_{ik}^{AC}+\lambda_{jk}^{BC}+\lambda_{ijk}^{ABC}\)
The main questions of interest are:
- What do the \(\lambda\) terms mean in this model?
- What hypotheses about them correspond to the models of independence we already know?
- What are some efficient ways to specify and interpret these models and tables?
- What are some efficient ways to fit and select among many possible models in three and higher dimensions?
As before for three-way tables, there are multiple models we can test and now fit. The log-linear models we will fit and evaluate are
- Saturated
- Complete Independence
- Joint Independence
- Conditional Independence
- Homogeneous Association
Example: Graduate Admissions
Let us go back to our familiar dataset on graduate admissions at Berkeley:
Dept. | Males admitted | Males rejected | Females admitted | Females rejected |
---|---|---|---|---|
A | 512 | 313 | 89 | 19 |
B | 353 | 207 | 17 | 8 |
C | 120 | 205 | 202 | 391 |
D | 139 | 279 | 131 | 244 |
E | 53 | 138 | 94 | 299 |
F | 22 | 351 | 24 | 317 |
Let D = department, S = sex, and A = admission status (rejected or accepted). We analyzed this as a three-way table before, and specifically, we looked at partial and marginal tables. Now we will look at it from a log-linear model point of view. Let \(Y\) be the observed frequency or count in a particular cell of the three-way table.
10.2.1 - Saturated Log-Linear Model
10.2.1 - Saturated Log-Linear ModelThis model is the default model in a way that serves for testing of goodness-of-fit of the other models. Recall that the saturated model has the maximum number of parameters and fitting a saturated model is the same as estimating ML parameters of distributions appropriate for each cell of the contingency table.
Main assumption
The \(N = IJK\) counts in the cells are assumed to be independent observations of a Poisson random variable.
Model structure
\(\log(\mu_{ijk})=\lambda+\lambda_i^A+\lambda_j^B+\lambda_k^C+\lambda_{ij}^{AB}+\lambda_{ik}^{AC}+\lambda_{jk}^{BC}+\lambda_{ijk}^{ABC}\)
Parameter constraints can be different, but the (typical) set-to-zero constraints imply that any \(\lambda\) for which a subscript corresponds to the last category (i.e., includes \(I\), \(J\), or \(K\)) is set to 0. For example,
\(\lambda_I^A=\lambda_J^B=\lambda_K^C=\lambda_{Ij}^{AB}=\cdots=\lambda_{ijK}^{ABC}=0\)
Parameter estimation and interpretation
- \(\lambda\) represents an "overall" effect or a grand mean (on the log scale) of the expected counts, and it ensures that \(\sum_i\sum_j\sum_k\mu_{ijk}=n\).
- \(\lambda_i^A\), \(\lambda_j^B\), and \(\lambda_k^C\) represent the "main" effects of variables \(A\), \(B\), and \(C\), or deviations from the grand mean.
- \(\lambda_{ij}^{AB}\), \(\lambda_{ik}^{AC}\), \(\lambda_{jk}^{BC}\) represent the interaction/association between two variables while controlling the third (e.g., conditional odds ratios, test for partial associations) and reflect the departure from independence
- \(\lambda_{ijk}^{ABC}\) represents the interaction/association between three variables and reflect the departure from independence
If there is a significant interaction term, we typically do not look at the lower-order terms but only interpret the higher-order terms because the values of lower-order terms are coding dependent and can be misleading.
Model Fit
The saturated model has a perfect fit with \(G^2=0\) and df = 0 since the number of cells is equal to the number of unique parameters in the model.
Model Selection
Relevant when comparing to simpler models. The saturated model is the most complex model possible.
Fitting in SAS and R
Using PROC GENMOD, let us fit the saturated log-linear model.
proc genmod data=berkeley order=data;
class D S A;
model count= D S A D*S D*A S*A D*S*A/dist=poisson link=log;
run;
When we use the order=data option, GENMOD orders the levels of class variables in the same order as they appear in the dataset. For each class variable, GENMOD creates a set of dummy using the last category as a reference group (recall the CATMOD and GENMOD coding from the previous lesson). Therefore, we can interpret a two-way association as the log-odds ratio for the two variables in question, with the other variable held constant at its last category (i.e., conditional odds-ratios).
Here's a portion of the SAS output that includes ML estimates.
Analysis Of Maximum Likelihood Parameter Estimates | ||||||
---|---|---|---|---|---|---|
Parameter | DF | Estimate | Standard Error |
|||
Intercept | 1 | 3.1781 | 0.2041 | |||
D | DeptA | 1 | 1.3106 | 0.2300 | ||
D | DeptB | 1 | -0.3448 | 0.3170 | ||
D | DeptC | 1 | 2.1302 | 0.2159 | ||
D | DeptD | 1 | 1.6971 | 0.2220 | ||
D | DeptE | 1 | 1.3652 | 0.2287 | ||
D | DeptF | 0 | 0.0000 | 0.0000 | ||
S | Male | 1 | -0.0870 | 0.2952 | ||
S | Female | 0 | 0.0000 | 0.0000 | ||
A | Reject | 1 | 2.5808 | 0.2117 | ||
A | Accept | 0 | 0.0000 | 0.0000 | ||
D*S | DeptA | Male | 1 | 1.8367 | 0.3167 | |
D*S | DeptA | Female | 0 | 0.0000 | 0.0000 | |
D*S | DeptB | Male | 1 | 3.1203 | 0.3857 | |
D*S | DeptB | Female | 0 | 0.0000 | 0.0000 | |
D*S | DeptC | Male | 1 | -0.4338 | 0.3169 | |
D*S | DeptC | Female | 0 | 0.0000 | 0.0000 | |
D*S | DeptD | Male | 1 | 0.1391 | 0.3194 | |
D*S | DeptD | Female | 0 | 0.0000 | 0.0000 | |
D*S | DeptE | Male | 1 | -0.4860 | 0.3415 | |
D*S | DeptE | Female | 0 | 0.0000 | 0.0000 | |
D*S | DeptF | Male | 0 | 0.0000 | 0.0000 | |
D*S | DeptF | Female | 0 | 0.0000 | 0.0000 | |
D*A | DeptA | Reject | 1 | -4.1250 | 0.3297 | |
D*A | DeptA | Accept | 0 | 0.0000 | 0.0000 | |
D*A | DeptB | Reject | 1 | -3.3346 | 0.4782 | |
D*A | DeptB | Accept | 0 | 0.0000 | 0.0000 | |
D*A | DeptC | Reject | 1 | -1.9204 | 0.2288 | |
D*A | DeptC | Accept | 0 | 0.0000 | 0.0000 | |
D*A | DeptD | Reject | 1 | -1.9589 | 0.2378 | |
D*A | DeptD | Accept | 0 | 0.0000 | 0.0000 | |
D*A | DeptE | Reject | 1 | -1.4237 | 0.2425 | |
D*A | DeptE | Accept | 0 | 0.0000 | 0.0000 | |
D*A | DeptF | Reject | 0 | 0.0000 | 0.0000 | |
D*A | DeptF | Accept | 0 | 0.0000 | 0.0000 | |
S*A | Male | Reject | 1 | 0.1889 | 0.3052 | |
S*A | Male | Accept | 0 | 0.0000 | 0.0000 | |
S*A | Female | Reject | 0 | 0.0000 | 0.0000 | |
S*A | Female | Accept | 0 | 0.0000 | 0.0000 | |
D*S*A | DeptA | Male | Reject | 1 | 0.8632 | 0.4027 |
D*S*A | DeptA | Male | Accept | 0 | 0.0000 | 0.0000 |
D*S*A | DeptA | Female | Reject | 0 | 0.0000 | 0.0000 |
D*S*A | DeptA | Female | Accept | 0 | 0.0000 | 0.0000 |
D*S*A | DeptB | Male | Reject | 1 | 0.0311 | 0.5335 |
... | ... | ... | ... | ... | ... | ... |
The scale parameter was held fixed.
By default, R will set the first category (first alphabetically) as the reference level with the set-to-zero constraint, which we can change manually if we wish. The results will be equivalent, but the interpretations of the estimates reported will be different. With this choice of reference, we can interpret a two-way association as the log-odds ratio for the two variables in question, with the other variable held constant at its last category (i.e., conditional odds-ratios). Also, note that the built-in data set UCBAdmissions uses "Gender" instead of "Sex".
UCBAdmissions
berk.data = as.data.frame(UCBAdmissions)
berk.data$Gender = relevel(berk.data$Gender, ref='Female')
berk.data$Dept = relevel(berk.data$Dept, ref='F')
berk.sat = glm(Freq~Admit*Gender*Dept, family=poisson(), data=berk.data)
Here's a portion of the summary output that includes ML estimates.
> summary(berk.sat)
Call:
glm(formula = Freq ~ Admit * Gender * Dept, family = poisson(),
data = berk.data)
Deviance Residuals:
[1] 0 0 0 0 0 0 0 0 0 0 0 0 0 0 0 0 0 0 0 0 0 0 0 0
Coefficients:
Estimate Std. Error z value Pr(>|z|)
(Intercept) 3.17805 0.20412 15.569 < 2e-16 ***
AdmitRejected 2.58085 0.21171 12.190 < 2e-16 ***
GenderMale -0.08701 0.29516 -0.295 0.7682
DeptA 1.31058 0.23001 5.698 1.21e-08 ***
DeptB -0.34484 0.31700 -1.088 0.2767
DeptC 2.13021 0.21591 9.866 < 2e-16 ***
DeptD 1.69714 0.22204 7.644 2.11e-14 ***
DeptE 1.36524 0.22870 5.969 2.38e-09 ***
AdmitRejected:GenderMale 0.18890 0.30516 0.619 0.5359
AdmitRejected:DeptA -4.12505 0.32968 -12.512 < 2e-16 ***
AdmitRejected:DeptB -3.33462 0.47817 -6.974 3.09e-12 ***
AdmitRejected:DeptC -1.92041 0.22876 -8.395 < 2e-16 ***
AdmitRejected:DeptD -1.95888 0.23781 -8.237 < 2e-16 ***
AdmitRejected:DeptE -1.42370 0.24250 -5.871 4.33e-09 ***
GenderMale:DeptA 1.83670 0.31672 5.799 6.66e-09 ***
GenderMale:DeptB 3.12027 0.38572 8.090 5.99e-16 ***
GenderMale:DeptC -0.43376 0.31687 -1.369 0.1710
GenderMale:DeptD 0.13907 0.31938 0.435 0.6632
GenderMale:DeptE -0.48599 0.34151 -1.423 0.1547
AdmitRejected:GenderMale:DeptA 0.86318 0.40267 2.144 0.0321 *
AdmitRejected:GenderMale:DeptB 0.03113 0.53349 0.058 0.9535
AdmitRejected:GenderMale:DeptC -0.31382 0.33741 -0.930 0.3523
AdmitRejected:GenderMale:DeptD -0.10691 0.34013 -0.314 0.7533
AdmitRejected:GenderMale:DeptE -0.38908 0.36500 -1.066 0.2864
---
Signif. codes: 0 ‘***’ 0.001 ‘**’ 0.01 ‘*’ 0.05 ‘.’ 0.1 ‘ ’ 1
(Dispersion parameter for poisson family taken to be 1)
Null deviance: 2.6501e+03 on 23 degrees of freedom
Residual deviance: 1.4788e-13 on 0 degrees of freedom
AIC: 207.06
The intercept is a normalizing constant and should be ignored. The main effects for D, S, and A are all difficult to interpret and not very meaningful since we have significant two-way and three-way associations; the two- and three-way associations are highly meaningful. For example, the estimated coefficient for the SA association is 0.1889.
Exponentiating this coefficient gives
\(\exp(0.1889)=1.208\),
which is the estimated SA odds ratio for Department F since that is the reference department in this analysis. The reference group for S is "female," and the reference group for A is "accept." If we write the \(2 \times 2\) table for \(S \times A\) in Department F, i.e., the "partial" table, with the reference groups in the last row and column, we get
Dept F | Reject | Accept |
---|---|---|
Men | 351 | 22 |
Women | 317 | 24 |
for which the estimated odds ratio is:
\(351 \times 24/317 \times 22=1.208\).
The Wald z-statistic for this coefficient,
\(z=0.1889/0.3052\),
which corresponds to Chi-square statistic \(0.62^2 = 0.38\) with p-value 0.5359 and indicates that the SA odds ratio for Department F is not significantly different from 1.00 or that the log odds ratio is not significantly different from 0.
The 95% confidence interval for the parameter estimate, that is, for the log odds ratio, is \((−0.4092, 0.7870)\). Thus the 95% confidence interval for the odds ratio is
\((\exp(-0.4092),\exp(0.7870))=(0.67,2.20)\).
To get the SA odds ratio for any other department, we have to combine the SA coefficient with one of the DSA coefficients. For example, the SA odds ratio for Department A is
\(\exp(0.1889+0.8632)=2.864\).
Based on:
\begin{align} \theta_{SA(i="A")} &= \dfrac{\mu_{ijk} \mu_{ij'k'}}{\mu_{ij'k} \mu_{ijk'}}\\ &= \\ &= (\lambda_{jk}+\lambda_{j'k'}-\lambda_{j'k}-\lambda_{jk'})+(\lambda_{ijk}+\lambda_{ij'k'}-\lambda_{ij'k}-\lambda_{ijk'})\\ &= (0.1889+0-0-0)+(0.8632+0-0-0) \end{align}
The Wald z-statistic for the first DSA coefficient,
\(z=0.8632/0.4027\),
indicates that the SA odds ratio for Department A is significantly different from the SA odds ratio in Department F. To see if the SA odds ratio in Department A is significantly different from 1.00, we would have to compute the standard error for the sum of the two coefficients using the estimated covariance matrix, or refit the model by fixing the level of interest equal to 0.
In many situations, however, we take recourse to the saturated model only as a last resort. As the number of variables grow, saturated models become more and more difficult to interpret. In the following sections, we look at simpler models which are useful in explaining the associations among the discrete variables of interest.
10.2.2 - Complete Independence
10.2.2 - Complete IndependenceThis is the most restrictive model in that all variables are assumed to be jointly independent, regardless of any conditioning. Equivalently, this requires that each two and three-way distribution factors into the product of the marginal distributions involved.
Main assumptions
- The \(N = IJK\) counts in the cells are assumed to be independent observations of a Poisson random variable, and
- there are no partial interactions: \(\lambda_{ij}^{AB} =\lambda_{ik}^{AC} =\lambda_{jk}^{BC}=0\), for all \(i, j, k\), and
- there is no three-way interaction: \(\lambda_{ijk}^{ABC}=0\) for all \(i, j, k\).
Note the constraints above are in addition to the usual set-to-zero or sum-to-zero constraints (present even in the saturated model) imposed to avoid overparameterization.
Model Structure
\(\log(\mu_{ijk})=\lambda+\lambda_i^A+\lambda_j^B+\lambda_k^C\)
In SAS, the model of complete independence (D, S, A) can be fitted with the following commands:
proc genmod data=berkeley order=data;
class D S A;
model count = D S A / dist=poisson link=log;
run;
What are the estimated odds of male vs. female in this example? From the output, the ML estimate of the parameter S-Male, thus, the odds of being male are higher than being female applicant:
\(\exp(0.382) = 1.467 = 2691/1835\)
with p-value < .0001 indicating that the odds are significantly different. Note these are odds, not odds ratios! (When we are dealing with main effects we do not look at odds ratios.)
What about the odds of being rejected? What can we conclude from the part of the output below?
Analysis Of Maximum Likelihood Parameter Estimates | ||||||||
---|---|---|---|---|---|---|---|---|
Parameter | DF | Estimate | Standard Error |
Wald 95% Confidence Limits | Wald Chi-Square | Pr > ChiSq | ||
Intercept | 1 | 4.7207 | 0.0455 | 4.6315 | 4.8100 | 10748.0 | <.0001 | |
D | DeptA | 1 | 0.2675 | 0.0497 | 0.1701 | 0.3650 | 28.95 | <.0001 |
D | DeptB | 1 | -0.1993 | 0.0558 | -0.3086 | -0.0900 | 12.77 | 0.0004 |
D | DeptC | 1 | 0.2513 | 0.0499 | 0.1535 | 0.3491 | 25.37 | <.0001 |
D | DeptD | 1 | 0.1037 | 0.0516 | 0.0025 | 0.2048 | 4.04 | 0.0445 |
D | DeptE | 1 | -0.2010 | 0.0558 | -0.3103 | -0.0916 | 12.98 | 0.0003 |
D | DeptF | 0 | 0.0000 | 0.0000 | 0.0000 | 0.0000 | . | . |
S | Male | 1 | 0.3829 | 0.0303 | 0.3235 | 0.4422 | 159.93 | <.0001 |
S | Female | 0 | 0.0000 | 0.0000 | 0.0000 | 0.0000 | . | . |
A | Reject | 1 | 0.4567 | 0.0305 | 0.3969 | 0.5165 | 224.15 | <.0001 |
A | Accept | 0 | 0.0000 | 0.0000 | 0.0000 | 0.0000 | . | . |
Scale | 0 | 1.0000 | 0.0000 | 1.0000 | 1.0000 |
The scale parameter was held fixed.
But, we should really check the overall fit of the model first, to determine if these estimates are meaningful.
Model Fit
The goodness-of-fit statistics indicate that the model does not fit.
Criteria For Assessing Goodness Of Fit | |||
---|---|---|---|
Criterion | DF | Value | Value/DF |
Deviance | 16 | 2097.6712 | 131.1045 |
Scaled Deviance | 16 | 2097.6712 | 131.1045 |
Pearson Chi-Square | 16 | 2000.3281 | 125.0205 |
Scaled Pearson X2 | 16 | 2000.3281 | 125.0205 |
Log Likelihood | 19464.3700 | ||
Full Log Likelihood | -1128.3655 | ||
AIC (smaller is better) | 2272.7309 | ||
AICC (smaller is better) | 2282.3309 | ||
BIC (smaller is better) | 2282.1553 |
If the model fits well, the "Value/DF" would be close to 1. Recall how we get the degrees of freedom:
df = number of cells - number of fitted parameters in the model.
df = number of fitted parameters in the saturated model - number of fitted parameters in our model.
Recall that these goodness-of-fit statistics compare the fitted model to the saturated model. Thus, the model of complete independence does not fit well in comparison to the saturated model.
In R, the model of complete independence can be fit with the following commands:
berk.ind = glm(Freq~Admit+Gender+Dept, family=poisson(), data=berk.data)
What are the estimated odds of male vs. female in this example? From the output, the ML estimate of the parameter GenderMale, thus, the odds of being male are higher than being female applicant:
\(\exp(0.382) = 1.467 = 2691/1835\)
with p-value < 2e-16, indicating that the odds are significantly different. Note these are odds, not odds ratios! (When we are dealing with main effects we do not look at odds ratios.)
> summary(berk.ind)
Call:
glm(formula = Freq ~ Admit + Gender + Dept, family = poisson(),
data = berk.data)
Deviance Residuals:
Min 1Q Median 3Q Max
-18.170 -7.719 -1.008 4.734 17.153
Coefficients:
Estimate Std. Error z value Pr(>|z|)
(Intercept) 4.72072 0.04553 103.673 < 2e-16 ***
AdmitRejected 0.45674 0.03051 14.972 < 2e-16 ***
GenderMale 0.38287 0.03027 12.647 < 2e-16 ***
DeptA 0.26752 0.04972 5.380 7.44e-08 ***
DeptB -0.19927 0.05577 -3.573 0.000352 ***
DeptC 0.25131 0.04990 5.036 4.74e-07 ***
DeptD 0.10368 0.05161 2.009 0.044533 *
DeptE -0.20098 0.05579 -3.602 0.000315 ***
---
Signif. codes: 0 ‘***’ 0.001 ‘**’ 0.01 ‘*’ 0.05 ‘.’ 0.1 ‘ ’ 1
(Dispersion parameter for poisson family taken to be 1)
Null deviance: 2650.1 on 23 degrees of freedom
Residual deviance: 2097.7 on 16 degrees of freedom
AIC: 2272.7
What about the odds of being rejected? What can we conclude from the part of the output above?
But, we should really check the overall fit of the model first, to determine if these estimates are meaningful.
Model Fit
The reported "Residual deviance" of 2097.7 on 16 degrees of freedom indicates that the model does not fit. If the model fits well, the "Value/DF" would be close to 1. Recall how we get the degrees of freedom:
df = number of cells - number of fitted parameters in the model.
df = number of fitted parameters in the saturated model - number of fitted parameters in our model.
Recall that this goodness-of-fit statistic compares the fitted model to the saturated model. Thus, the model of complete independence does not fit well in comparison to the saturated model.
Next, let us see an example of the joint independence model.
10.2.3 - Joint Independence
10.2.3 - Joint IndependenceWith three variables, there are three ways to satisfy joint independence. The assumption is that one variable is independent of the other two, but the latter two can have an arbitrary association. For example, if \(A\) is jointly independent of \(B\) and \(C\), which we denote by \((A, BC)\), then \(A\) is independent of each of the others, and we can factor the three-way joint distribution into the product of the marginal distribution for \(A\) and the two-way joint distribution of \((B,C)\).
Main assumptions
(these are stated for the case \((A,BC)\) but hold in a similar way for \((B, AC)\) and \((C, AB)\) as well)
- The \(N = IJK\) counts in the cells are assumed to be independent observations of a Poisson random variable, and
- there are no partial interactions involving \(A\): \(\lambda_{ij}^{AB} =\lambda_{ik}^{AC} =0\), for all \(i, j, k\), and
- there is no three-way interaction: \(\lambda_{ijk}^{ABC}=0\) for all \(i, j, k\).
Note the constraints above are in addition to the usual set-to-zero or sum-to-zero constraints (present even in the saturated model) imposed to avoid overparameterization.
Model Structure
\(\log(\mu_{ijk})=\lambda+\lambda_i^A+\lambda_j^B+\lambda_k^C +\lambda_{jk}^{BC}\)
In the example below, we consider the model where admission status is jointly independent of department and sex, which we denote by \((A, DS)\).
In SAS, the model can be fitted like this:
proc genmod data=berkeley order=data;
class D S A;
model count = D S A D*S / dist=poisson link=log lrci type3 obstats;
run;
This model implies that the association between D and S does NOT depend on the level of the variable A. That is, the association between department and sex is independent of the rejection/acceptance decision.
\(\theta_{DS(A=reject)}=\theta_{DS(A=accept)}=\dfrac{\text{exp}(\lambda_{ij}^{DS})\text{exp}(\lambda_{i'j'}^{DS})}{\text{exp}(\lambda_{i'j}^{DS})\text{exp}(\lambda_{ij'}^{DS})}\)
Since we are assuming that the (DS) distribution is independent of A, then we are assuming that the conditional distribution of DS, given A, is the same as the unconditional distribution of DS. And equivalently, the conditional distribution of A, given DS, is the same as the unconditional distribution of A. In other words, if this model fits well, neither department nor sex tells us anything significant about admission status.
The first estimated coefficient 1.9436 for the DS associations...
D*S DeptA Male 1 1.9436 0.1268 1.6950 2.1921 234.84
implies that the estimated odds ratio between sex and department (specifically, A versus F) is \(\exp(1.9436) = 6.98\) with 95% CI
\((\exp(1.695), \exp(2.192))= (5.45, 8.96)\)
But, we should really check the overall fit of the model first, to determine if these estimates are meaningful.
Model Fit
The goodness-of-fit statistics indicate that the model does not fit since the "Value/df" is much larger than 1.
Criteria For Assessing Goodness Of Fit | |||
---|---|---|---|
Criterion | DF | Value | Value/DF |
Deviance | 11 | 877.0564 | 79.7324 |
Scaled Deviance | 11 | 877.0564 | 79.7324 |
Pearson Chi-Square | 11 | 797.7041 | 72.5186 |
Scaled Pearson X2 | 11 | 797.7041 | 72.5186 |
Log Likelihood | 20074.6774 |
How did we get these degrees of freedom? As usual, we're comparing this model to the saturated model, and the df is the difference in the numbers of parameters involved:
\(DF = (IJK-1) - [(I-1)+(J-1)+(K-1)+(J-1)(K-1)] = (I-1)(JK-1) \)
With \(I=2\), \(J=2\), and \(K=6\) corresponding to the levels of A, S, and D, respectively, this works out to be 11.
As before, we can look at the residuals for more information about why this model fits poorly. Recall that adjusted residuals have approximately a N(0, 1) distribution (i.e., "Std Pearson Residual"). In general, we have a lack of fit if (1) we have a large number of cells and adjusted residuals are greater than 3, or (2) we have a small number of cells and adjusted residuals are greater than 2. Here is only part of the output. Notice that the absolute value of the standardized residual for the first six cells are all large (e.g., in cell 1, the value is \(-15.1793\)). Many other such residuals are rather large as well, indicating that this model fits poorly.
Evaluate the residuals
Observation Statistics | ||||||||||||||||||||||||||||||||||||||||||||
---|---|---|---|---|---|---|---|---|---|---|---|---|---|---|---|---|---|---|---|---|---|---|---|---|---|---|---|---|---|---|---|---|---|---|---|---|---|---|---|---|---|---|---|---|
Observation | count | D | S | A | Predicted Value | Linear Predictor | Standard Error of the Linear Predictor | HessWgt | Lower | Upper | Raw Residual | Pearson Residual | Deviance Residual | Std Deviance Residual | Std Pearson Residual | Likelihood Residual | Leverage | CookD | DFBETA_Intercept | DFBETA_DDeptA | DFBETA_DDeptB | DFBETA_DDeptC | DFBETA_DDeptD | DFBETA_DDeptE | DFBETA_SMale | DFBETA_AReject | DFBETA_DDeptASMale | DFBETA_DDeptBSMale | DFBETA_DDeptCSMale | DFBETA_DDeptDSMale | DFBETA_DDeptESMale | DFBETAS_Intercept | DFBETAS_DDeptA | DFBETAS_DDeptB | DFBETAS_DDeptC | DFBETAS_DDeptD | DFBETAS_DDeptE | DFBETAS_SMale | DFBETAS_AReject | DFBETAS_DDeptASMale | DFBETAS_DDeptBSMale | DFBETAS_DDeptCSMale | DFBETAS_DDeptDSMale | DFBETAS_DDeptESMale |
1 | 313 | DeptA | Male | Reject | 505.09831 | 6.2247531 | 0.0367703 | 505.09831 | 469.97739 | 542.84378 | -192.0983 | -8.547431 | -9.199152 | -16.3367 | -15.17931 | -15.55562 | 0.6829213 | 38.173694 | 0.1338572 | 1.051E-15 | 0 | 0 | -2.63E-16 | 0 | 0 | -0.218635 | -0.734349 | 5.255E-16 | 0 | 0 | 0 | 2.3367482 | 9.518E-15 | 0 | 0 | -3.51E-15 | 0 | 0 | -7.166703 | -5.790199 | 2.414E-15 | 0 | 0 | 0 |
2 | 512 | DeptA | Male | Accept | 319.90169 | 5.7680137 | 0.0395092 | 319.90169 | 296.06444 | 345.65816 | 192.09831 | 10.740272 | 9.8692317 | 13.948262 | 15.179309 | 14.575998 | 0.4993589 | 17.678562 | 0.1338572 | -1.33E-15 | -4.99E-16 | -4.99E-16 | -4.99E-16 | -4.99E-16 | -6.66E-16 | -0.218635 | 0.4650965 | 3.328E-16 | 6.656E-16 | 6.656E-16 | 6.656E-16 | 2.3367481 | -1.21E-14 | -2.41E-15 | -7.35E-15 | -6.67E-15 | -6.75E-15 | -8.88E-15 | -7.166703 | 3.6671961 | 1.529E-15 | 6.534E-15 | 6.441E-15 | 5.751E-15 |
3 | 19 | DeptA | Female | Reject | 66.122038 | 4.1915021 | 0.0969494 | 66.122038 | 54.679277 | 79.959431 | -47.12204 | -5.794967 | -6.845121 | -11.12613 | -9.419201 | -10.09928 | 0.6214932 | 11.205917 | 0.0275065 | -1.152726 | -7.77E-16 | -7.77E-16 | -7.86E-16 | -7.77E-16 | -1.13E-15 | -0.044928 | 1.1527259 | 1.062E-15 | 1.074E-15 | 1.075E-15 | 1.128E-15 | 0.4801819 | -10.4398 | -3.75E-15 | -1.14E-14 | -1.05E-14 | -1.05E-14 | -1.51E-14 | -1.472697 | 9.0890214 | 4.878E-15 | 1.054E-14 | 1.04E-14 | 9.745E-15 |
4 | 89 | DeptA | Female | Accept | 41.878088 | 3.7347627 | 0.0980209 | 41.878088 | 34.558213 | 50.748407 | 47.121912 | 7.2816446 | 6.3202597 | 8.1755761 | 9.4191761 | 8.6973682 | 0.402369 | 4.5948769 | 0.0275065 | 0.7300717 | 3.761E-16 | 3.761E-16 | 3.761E-16 | 3.761E-16 | 5.813E-16 | -0.044928 | -0.730072 | -5.47E-16 | -5.47E-16 | -5.47E-16 | -5.81E-16 | 0.4801807 | 6.6119817 | 1.815E-15 | 5.535E-15 | 5.027E-15 | 5.083E-15 | 7.759E-15 | -1.472693 | -5.756474 | -2.51E-15 | -5.37E-15 | -5.29E-15 | -5.02E-15 |
5 | 207 | DeptB | Male | Reject | 342.85461 | 5.8373065 | 0.0438822 | 342.85461 | 314.59903 | 373.64795 | -135.8546 | -7.337015 | -7.925271 | -13.59608 | -12.58691 | -12.93864 | 0.6602177 | 23.679966 | 0.0883403 | -6.94E-16 | 0 | -6.94E-16 | -6.94E-16 | -3.47E-16 | -6.94E-16 | -0.14429 | 1.04E-15 | -0.713979 | 6.936E-16 | 1.04E-15 | 6.936E-16 | 1.5421589 | -6.28E-15 | 0 | -1.02E-14 | -9.27E-15 | -4.69E-15 | -9.26E-15 | -4.729733 | 8.203E-15 | -3.279442 | 6.809E-15 | 1.007E-14 | 5.993E-15 |
6 | 353 | DeptB | Male | Accept | 217.14539 | 5.3805671 | 0.0462014 | 217.14539 | 198.34621 | 237.72635 | 135.85461 | 9.2193238 | 8.4461135 | 11.531262 | 12.586906 | 12.032087 | 0.4635118 | 10.529199 | 0.0883403 | -1.1E-16 | 0 | 1.098E-16 | 0 | -1.1E-16 | 0 | -0.14429 | -2.2E-16 | 0.4521955 | 0 | -2.2E-16 | 0 | 1.5421589 | -9.95E-16 | 0 | 1.616E-15 | 0 | -1.48E-15 | 0 | -4.729733 | -1.73E-15 | 2.0770196 | 0 | -2.13E-15 | 0 |
7 | 8 | DeptB | Female | Reject | 15.30601 | 2.7282455 | 0.2003495 | 15.30601 | 10.335325 | 22.667301 | -7.30601 | -1.867451 | -2.056977 | -3.312462 | -3.007258 | -3.128479 | 0.6143822 | 1.108357 | 0.0041861 | 1.682E-16 | -0.75785 | 1.939E-16 | 1.926E-16 | 1.939E-16 | 1.981E-16 | -0.006837 | -1.95E-16 | 0.7578499 | -2.47E-16 | -2.31E-16 | -2.39E-16 | 0.0730767 | 1.523E-15 | -3.657546 | 2.853E-15 | 2.574E-15 | 2.62E-15 | 2.644E-15 | -0.224123 | -1.54E-15 | 3.4809481 | -2.43E-15 | -2.23E-15 | -2.07E-15 |
8 | 17 | DeptB | Female | Accept | 9.6939907 | 2.2715062 | 0.2008702 | 9.6939907 | 6.5391536 | 14.37089 | 7.3060093 | 2.3465452 | 2.1180239 | 2.7143924 | 3.0072581 | 2.8325522 | 0.3911414 | 0.4469052 | 0.0041861 | -1.3E-16 | 0.4799807 | -1.41E-16 | -1.41E-16 | -1.41E-16 | -1.46E-16 | -0.006837 | 1.457E-16 | -0.479981 | 1.769E-16 | 1.665E-16 | 1.717E-16 | 0.0730767 | -1.18E-15 | 2.3164901 | -2.07E-15 | -1.88E-15 | -1.9E-15 | -1.94E-15 | -0.224123 | 1.149E-15 | -2.204642 | 1.737E-15 | 1.612E-15 | 1.484E-15 |
9 | 205 | DeptC | Male | Reject | 198.97812 | 5.2931949 | 0.0567174 | 198.97812 | 178.04404 | 222.3736 | 6.0218769 | 0.426903 | 0.4247764 | 0.7080436 | 0.7115884 | 0.7103146 | 0.6400844 | 0.0692709 | -0.003697 | 2.177E-17 | 2.902E-17 | 0 | 2.177E-17 | 1.451E-17 | 2.902E-17 | 0.006038 | -2.9E-17 | -4.35E-17 | 0.0514811 | -2.9E-17 | -2.9E-17 | -0.064534 | 1.971E-16 | 1.401E-16 | 0 | 2.909E-16 | 1.961E-16 | 3.874E-16 | 0.197922 | -2.29E-16 | -2E-16 | 0.5053783 | -2.81E-16 | -2.51E-16 |
10 | 120 | DeptC | Male | Accept | 126.02188 | 4.8364555 | 0.0585301 | 126.02188 | 112.36343 | 141.34059 | -6.021879 | -0.536425 | -0.540785 | -0.717372 | -0.711589 | -0.714881 | 0.431723 | 0.029591 | -0.003697 | 9.191E-18 | -4.6E-18 | 1.838E-17 | 4.596E-18 | 4.596E-18 | 0 | 0.006038 | 0 | 9.191E-18 | -0.032605 | 0 | 0 | -0.064534 | 8.324E-17 | -2.22E-17 | 2.705E-16 | 6.142E-17 | 6.21E-17 | 0 | 0.1979221 | 0 | 4.222E-17 | -0.320079 | 0 | 0 |
11 | 391 | DeptC | Female | Reject | 363.05854 | 5.8945641 | 0.0427349 | 363.05854 | 333.88785 | 394.77779 | 27.941455 | 1.4664278 | 1.4481968 | 2.4948336 | 2.5262405 | 2.5157016 | 0.6630449 | 0.9659997 | -0.018322 | 1.899E-17 | -9.36E-17 | 0.1398371 | -1.58E-17 | 1.433E-17 | -3.99E-18 | 0.0299254 | -1E-17 | 1.916E-16 | -0.139837 | 3.936E-17 | 3.992E-18 | -0.31984 | 1.719E-16 | -4.52E-16 | 2.0575648 | -2.11E-16 | 1.936E-16 | -5.33E-17 | 0.9809346 | -7.92E-17 | 8.802E-16 | -1.372749 | 3.809E-16 | 3.449E-17 |
12 | 202 | DeptC | Female | Accept | 229.94146 | 5.4378247 | 0.0451131 | 229.94146 | 210.48294 | 251.19886 | -27.94146 | -1.84264 | -1.881985 | -2.580183 | -2.526241 | -2.555081 | 0.4679758 | 0.4318154 | -0.018322 | 9.111E-17 | 1.367E-16 | -0.088565 | 9.111E-17 | 6.833E-17 | 9.111E-17 | 0.0299254 | -9.11E-17 | -2.05E-16 | 0.0885652 | -1.14E-16 | -9.11E-17 | -0.31984 | 8.251E-16 | 6.595E-16 | -1.303149 | 1.218E-15 | 9.233E-16 | 1.216E-15 | 0.9809347 | -7.18E-16 | -9.42E-16 | 0.8694243 | -1.1E-15 | -7.87E-16 |
13 | 279 | DeptD | Male | Reject | 255.30424 | 5.5424559 | 0.0503787 | 255.30424 | 231.29997 | 281.79967 | 23.695762 | 1.4830018 | 1.4609054 | 2.4622378 | 2.4994795 | 2.4864328 | 0.6479663 | 0.8845534 | -0.014872 | 2.919E-17 | 0 | -2.92E-17 | 0 | -2.92E-17 | 0 | 0.0242913 | 0 | -5.84E-17 | 0 | 0.1614174 | 0 | -0.259622 | 2.644E-16 | 0 | -4.3E-16 | 0 | -3.94E-16 | 0 | 0.7962501 | 0 | -2.68E-16 | 0 | 1.5620689 | 0 |
14 | 138 | DeptD | Male | Accept | 161.69576 | 5.0857166 | 0.0524112 | 161.69576 | 145.91036 | 179.18892 | -23.69576 | -1.863466 | -1.912007 | -2.564589 | -2.49948 | -2.535876 | 0.444168 | 0.3840251 | -0.014872 | 7.395E-17 | 5.546E-17 | 7.395E-17 | 7.395E-17 | 7.395E-17 | 7.395E-17 | 0.0242913 | -7.4E-17 | -3.7E-17 | -7.4E-17 | -0.102233 | -7.4E-17 | -0.259622 | 6.698E-16 | 2.677E-16 | 1.088E-15 | 9.883E-16 | 9.993E-16 | 9.871E-16 | 0.7962502 | -5.83E-16 | -1.7E-16 | -7.26E-16 | -0.989329 | -6.39E-16 |
15 | 244 | DeptD | Female | Reject | 229.59014 | 5.4362957 | 0.0529774 | 229.59014 | 206.94685 | 254.71097 | 14.409858 | 0.9510056 | 0.941309 | 1.5784537 | 1.5947136 | 1.5889501 | 0.644368 | 0.3544502 | -0.008952 | 9.714E-17 | 4.215E-17 | 7.729E-17 | 0.1080507 | 9.486E-17 | 1.562E-16 | 0.0146225 | -1.63E-16 | -8.21E-17 | -1.21E-16 | -0.108051 | -1.39E-16 | -0.156284 | 8.797E-16 | 2.034E-16 | 1.137E-15 | 1.4439895 | 1.282E-15 | 2.085E-15 | 0.479316 | -1.29E-15 | -3.77E-16 | -1.19E-15 | -1.045628 | -1.2E-15 |
16 | 131 | DeptD | Female | Accept | 145.40986 | 4.9795564 | 0.0549138 | 145.40986 | 130.57234 | 161.93344 | -14.40986 | -1.194986 | -1.215586 | -1.622204 | -1.594714 | -1.610208 | 0.4384866 | 0.152763 | -0.008953 | -1.11E-17 | 1.113E-17 | -1.11E-17 | -0.068433 | -2.23E-17 | -5.56E-17 | 0.0146225 | 5.565E-17 | 1.113E-17 | 3.339E-17 | 0.0684334 | 4.452E-17 | -0.156284 | -1.01E-16 | 5.371E-17 | -1.64E-16 | -0.914544 | -3.01E-16 | -7.43E-16 | 0.4793161 | 4.388E-16 | 5.112E-17 | 3.278E-16 | 0.6622441 | 3.847E-16 |
17 | 138 | DeptE | Male | Reject | 116.93791 | 4.7616431 | 0.0733181 | 116.93791 | 101.28541 | 135.00933 | 21.062088 | 1.9477076 | 1.8932348 | 3.106604 | 3.1959883 | 3.1630862 | 0.6286041 | 1.3298634 | -0.01253 | -2.46E-17 | 0 | -4.92E-17 | 0 | -9.84E-17 | -4.92E-17 | 0.0204658 | 4.919E-17 | 0 | 9.838E-17 | 4.919E-17 | 0.2969142 | -0.218736 | -2.23E-16 | 0 | -7.24E-16 | 0 | -1.33E-15 | -6.57E-16 | 0.6708529 | 3.878E-16 | 0 | 9.657E-16 | 4.76E-16 | 2.5655562 |
18 | 53 | DeptE | Male | Accept | 74.062089 | 4.3049038 | 0.0747292 | 74.062089 | 63.971469 | 85.744365 | -21.06209 | -2.447392 | -2.579791 | -3.368885 | -3.195988 | -3.298475 | 0.4135965 | 0.5541756 | -0.01253 | 9.346E-17 | 4.673E-17 | 7.788E-17 | 6.231E-17 | 9.346E-17 | 9.346E-17 | 0.0204658 | -9.35E-17 | -6.23E-17 | -1.25E-16 | -9.35E-17 | -0.188049 | -0.218736 | 8.464E-16 | 2.255E-16 | 1.146E-15 | 8.327E-16 | 1.263E-15 | 1.247E-15 | 0.6708529 | -7.37E-16 | -2.86E-16 | -1.22E-15 | -9.04E-16 | -1.624883 |
19 | 299 | DeptE | Female | Reject | 240.61047 | 5.4831793 | 0.0518118 | 240.61047 | 217.37632 | 266.32799 | 58.389531 | 3.7642437 | 3.6255985 | 6.0928851 | 6.3258808 | 6.2443737 | 0.6459102 | 5.6150979 | -0.036434 | 3.776E-17 | -1.86E-16 | 2.849E-17 | 4.013E-17 | 0.4195937 | 1.351E-16 | 0.0595093 | -1.63E-16 | 9.503E-17 | -6.36E-17 | -1.36E-16 | -0.419594 | -0.636029 | 3.419E-16 | -8.98E-16 | 4.193E-16 | 5.362E-16 | 5.669627 | 1.803E-15 | 1.9506733 | -1.29E-15 | 4.365E-16 | -6.24E-16 | -1.32E-15 | -3.625598 |
20 | 94 | DeptE | Female | Accept | 152.38953 | 5.02644 | 0.0537902 | 152.38953 | 137.14149 | 169.33293 | -58.38953 | -4.72996 | -5.093896 | -6.812612 | -6.325881 | -6.602426 | 0.4409215 | 2.4276557 | -0.036434 | 1.812E-16 | 2.718E-16 | 1.359E-16 | 1.359E-16 | -0.265748 | 9.059E-17 | 0.0595093 | -9.06E-17 | -2.26E-16 | -1.36E-16 | -9.06E-17 | 0.2657478 | -0.636029 | 1.641E-15 | 1.312E-15 | 1.999E-15 | 1.816E-15 | -3.590832 | 1.209E-15 | 1.9506734 | -7.14E-16 | -1.04E-15 | -1.33E-15 | -8.77E-16 | 2.2962557 |
21 | 351 | DeptF | Male | Reject | 228.36589 | 5.4309491 | 0.0531121 | 228.36589 | 205.78898 | 253.41969 | 122.63411 | 8.1151336 | 7.5151464 | 12.598896 | 13.604754 | 13.255617 | 0.6441967 | 25.777847 | -0.076153 | 1.196E-15 | -4.48E-16 | 0 | 7.474E-16 | 2.99E-16 | 0.9240428 | 0.1243841 | -0.924043 | -0.924043 | -0.924043 | -0.924043 | -0.924043 | -1.329404 | 1.083E-14 | -2.16E-15 | 0 | 9.988E-15 | 4.039E-15 | 12.333171 | 4.077222 | -7.285899 | -4.244304 | -9.071119 | -8.942148 | -7.984408 |
22 | 22 | DeptF | Male | Accept | 144.63448 | 4.9742098 | 0.0550438 | 144.63448 | 129.84299 | 161.111 | -122.6345 | -10.1971 | -12.744 | -17.00283 | -13.6048 | -15.6051 | 0.4382161 | 11.106051 | -0.076153 | -2.84E-16 | 5.68E-16 | 2.84E-16 | -9.47E-17 | 9.467E-17 | -0.58524 | 0.1243845 | 0.58524 | 0.58524 | 0.58524 | 0.58524 | 0.58524 | -1.329408 | -2.57E-15 | 2.741E-15 | 4.179E-15 | -1.27E-15 | 1.279E-15 | -7.811181 | 4.0772344 | 4.6145047 | 2.6881185 | 5.745169 | 5.6634853 | 5.0569034 |
23 | 317 | DeptF | Female | Reject | 208.77408 | 5.3412527 | 0.05543 | 208.77408 | 187.28133 | 232.73339 | 108.22592 | 7.4901924 | 6.9525291 | 11.611039 | 12.508961 | 12.194621 | 0.6414552 | 21.533862 | 0.8184913 | -0.885183 | -0.885183 | -0.885183 | -0.885183 | -0.885183 | -0.885183 | 0.1089309 | 0.8851832 | 0.8851832 | 0.8851832 | 0.8851832 | 0.8851832 | 14.288419 | -8.016767 | -4.272084 | -13.0246 | -11.82959 | -11.96076 | -11.81451 | 3.5706788 | 6.9794986 | 4.0658143 | 8.6896432 | 8.5660954 | 7.6486325 |
24 | 24 | DeptF | Female | Accept | 132.22611 | 4.8845134 | 0.0572835 | 132.22611 | 118.18364 | 147.93708 | -108.2261 | -9.411816 | -11.59923 | -15.41621 | -12.50898 | -14.22795 | 0.4338873 | 9.225178 | -0.62732 | 0.5606277 | 0.5606277 | 0.5606277 | 0.5606277 | 0.5606277 | 0.5606277 | 0.1089311 | -0.560628 | -0.560628 | -0.560628 | -0.560628 | -0.560628 | -10.95113 | 5.0773916 | 2.7057097 | 8.2490839 | 7.4922269 | 7.5753036 | 7.4826807 | 3.5706851 | -4.420441 | -2.575069 | -5.503555 | -5.425307 | -4.844235 |
In R, one way to fit the (A, DS) model is with the following command:
berk.jnt = glm(Freq~Admit+Gender+Dept+Gender*Dept, family=poisson(), data=berk.data)
This model implies that association between D and S does NOT depend on level of the variable A. That is, the association between department and sex is independent of the rejection/acceptance decision.
\(\theta_{DS(A=reject)}=\theta_{DS(A=accept)}=\dfrac{\text{exp}(\lambda_{ij}^{DS})\text{exp}(\lambda_{i'j'}^{DS})}{\text{exp}(\lambda_{i'j}^{DS})\text{exp}(\lambda_{ij'}^{DS})}\)
Since we are assuming that the (DS) distribution is independent of A, then we are assuming that the conditional distribution of DS, given A, is the same as the unconditional distribution of DS. And equivalently, the conditional distribution of A, given DS, is the same as the unconditional distribution of A. In other words, if this model fits well, neither department nor sex tells us anything significant about admission status.
The first estimated coefficient 1.9436 for the DS associations implies that the estimated odds ratio between sex and department (specifically, A versus F) is \(\exp(1.9436) = 6.98\) with 95% CI
\((\exp(1.695), \exp(2.192))= (5.45, 8.96)\)
> summary(berk.jnt)
Call:
glm(formula = Freq ~ Admit + Gender + Dept + Gender * Dept, family = poisson(),
data = berk.data)
Deviance Residuals:
Min 1Q Median 3Q Max
-12.744 -3.208 -0.058 2.495 9.869
Coefficients:
Estimate Std. Error z value Pr(>|z|)
(Intercept) 4.88451 0.05728 85.269 < 2e-16 ***
AdmitRejected 0.45674 0.03051 14.972 < 2e-16 ***
GenderMale 0.08970 0.07492 1.197 0.2312
DeptA -1.14975 0.11042 -10.413 < 2e-16 ***
DeptB -2.61301 0.20720 -12.611 < 2e-16 ***
DeptC 0.55331 0.06796 8.141 3.91e-16 ***
DeptD 0.09504 0.07483 1.270 0.2040
DeptE 0.14193 0.07401 1.918 0.0551 .
GenderMale:DeptA 1.94356 0.12683 15.325 < 2e-16 ***
GenderMale:DeptB 3.01937 0.21771 13.869 < 2e-16 ***
GenderMale:DeptC -0.69107 0.10187 -6.784 1.17e-11 ***
GenderMale:DeptD 0.01646 0.10334 0.159 0.8734
GenderMale:DeptE -0.81123 0.11573 -7.010 2.39e-12 ***
---
Signif. codes: 0 ‘***’ 0.001 ‘**’ 0.01 ‘*’ 0.05 ‘.’ 0.1 ‘ ’ 1
(Dispersion parameter for poisson family taken to be 1)
Null deviance: 2650.10 on 23 degrees of freedom
Residual deviance: 877.06 on 11 degrees of freedom
AIC: 1062.1
But, we should really check the overall fit of the model first, to determine if these estimates are meaningful.
Model Fit
The goodness-of-fit statistic (in the output as "Residual deviance") 877.06 indicates that the model does not fit since the "Value/df" is much larger than 1.
How did we get these degrees of freedom? As usual, we're comparing this model to the saturated model, and the df is the difference in the numbers of parameters involved:
\(DF = (IJK-1) - [(I-1)+(J-1)+(K-1)+(J-1)(K-1)] = (I-1)(JK-1) \)
With \(I=2\), \(J=2\), and \(K=6\) corresponding to the levels of A, S, and D, respectively, this works out to be 11.
As before, we can look at the residuals for more information about why this model fits poorly. Recall that adjusted residuals have approximately a N(0, 1) distribution. In general, we have a lack of fit if (1) we have a large number of cells and adjusted residuals are greater than 3, or (2) we have a small number of cells and adjusted residuals are greater than 2. Here is only part of the output. Notice that the absolute value of the standardized residual for the first six cells are all large (e.g., in cell 1, the value is \(-15.18\)). Many other such residuals are rather large as well, indicating that this model fits poorly.
Evaluate the residuals
> fits = fitted(berk.jnt)
> resids = residuals(berk.jnt,type="pearson")
> h = lm.influence(berk.jnt)$hat
> adjresids = resids/sqrt(1-h)
> round(cbind(berk.data$Freq,fits,adjresids),2)
fits adjresids
1 512 319.90 15.18
2 313 505.10 -15.18
3 89 41.88 9.42
4 19 66.12 -9.42
5 353 217.15 12.59
6 207 342.85 -12.59
...
Next, let us look at what is often the most interesting model of conditional independence.
10.2.4 - Conditional Independence
10.2.4 - Conditional IndependenceWith three variables, there are three ways to satisfy conditional independence. The assumption is that two variables are independent, given the third. For example, if \(A\) and \(B\) are conditionally independent, given \(C\), which we denote by \((AC, BC)\), then the conditional distribution of \((AB)\), given \(C\), can be factored into the product of the two conditional marginals, given \(C\).
Main assumptions
(these are stated for the case \((AC,BC)\) but hold in a similar way for \((AB, BC)\) and \((AB, AC)\) as well):
- The \(N = IJK\) counts in the cells are assumed to be independent observations of a Poisson random variable, and
- there is no partial interaction between \(A\) and \(B\): \(\lambda_{ij}^{AB} =0\), for all \(i, j\), and
- there is no three-way interaction: \(\lambda_{ijk}^{ABC}=0\) for all \(i, j, k\).
Note the constraints above are in addition to the usual set-to-zero or sum-to-zero constraints (present even in the saturated model) imposed to avoid overparameterization.
Model Structure
\(\log(\mu_{ijk})=\lambda+\lambda_i^A+\lambda_j^B+\lambda_k^C +\lambda_{ik}^{AC}+\lambda_{jk}^{BC}\)
In the example below, we consider the model where admission status and department are conditionally independent, given sex, which we denote by \((AS, DS)\).
In SAS, this model can be fitted as:
proc genmod data=berkeley order=data;
class D S A;
model count = D S A A*S D*S / dist=poisson link=log lrci type3 obstats;
run;
Model Fit:
The goodness-of-fit statistics indicate that the model does not fit.
Criteria For Assessing Goodness Of Fit | |||
---|---|---|---|
Criterion | DF | Value | Value/DF |
Deviance | 10 | 783.6070 | 78.3607 |
Scaled Deviance | 10 | 783.6070 | 78.3607 |
Pearson Chi-Square | 10 | 715.2958 | 71.5296 |
Scaled Pearson X2 | 10 | 715.2958 | 71.5296 |
Log Likelihood | 20121.4021 |
How did we get these DF?
\(DF = (IJK-1) - [(I-1)+(J-1)+(K-1)+(I-1)(J-1)+(J-1)(K-1)]=J(I-1)(K-1) \)
With \(I=2\), \(J=2\), and \(K=6\) corresponding to the levels of A, S, and D, respectively, this works out to be 10.
So where is the lack of fit? As before, we look at residuals. For example, the adjusted residual for the first cell is \(-12.1747\), a great deviation from zero.
We can also evaluate overall the individual parameters and their significance:
LR Statistics For Type 3 Analysis | |||
---|---|---|---|
Source | DF | Chi-Square | Pr > ChiSq |
D | 5 | 313.04 | <.0001 |
S | 1 | 333.24 | <.0001 |
A | 1 | 283.32 | <.0001 |
S*A | 1 | 93.45 | <.0001 |
D*S | 5 | 1220.61 | <.0001 |
This is like an ANOVA table in ANOVA and regression models. All parameters are significantly different from zero. That is they contribute significantly in describing the relationships between our variables, but the overall lack of fit of the model suggests that they are not sufficient.
In R, the (AS, DS) model can be fitted with
berk.cnd = glm(Freq~Admit+Gender+Dept+Admit*Gender+Dept*Gender, family=poisson(), data=berk.data)
Model Fit:
The goodness-of-fit statistics indicate that the model does not fit, e.g., Residual deviance: 783.6 on 10 degrees of freedom
How did we get these DF?
\(DF = (IJK-1) - [(I-1)+(J-1)+(K-1)+(I-1)(J-1)+(J-1)(K-1)]=J(I-1)(K-1) \)
With \(I=2\), \(J=2\), and \(K=6\) corresponding to the levels of A, S, and D, respectively, this works out to be 10.
So where is the lack of fit? As before, we look at residuals. For example, the adjusted residual for the first cell is \(-12.17\), a great deviation from zero.
> fits = fitted(berk.cnd)
> resids = residuals(berk.cnd,type="pearson")
> h = lm.influence(berk.cnd)$hat
> adjresids = resids/sqrt(1-h)
> round(cbind(berk.data$Freq,fits,adjresids),2)
fits adjresids
1 512 367.28 12.17
2 313 457.72 -12.17
3 89 32.78 12.13
4 19 75.22 -12.13
5 353 249.31 9.91
6 207 310.69 -9.91
...
We can also evaluate overall the individual parameters and their significance. However, the ANOVA table R reports for glm-fitted models uses the sequential sum of squares, which depend on the order terms are added to the model.
> anova(berk.cnd, test="LR")
Analysis of Deviance Table
Model: poisson, link: log
Response: Freq
Terms added sequentially (first to last)
Df Deviance Resid. Df Resid. Dev Pr(>Chi)
NULL 23 2650.10
Admit 1 230.03 22 2420.07 < 2.2e-16 ***
Gender 1 162.87 21 2257.19 < 2.2e-16 ***
Dept 5 159.52 16 2097.67 < 2.2e-16 ***
Admit:Gender 1 93.45 15 2004.22 < 2.2e-16 ***
Gender:Dept 5 1220.61 10 783.61 < 2.2e-16 ***
---
Signif. codes: 0 ‘***’ 0.001 ‘**’ 0.01 ‘*’ 0.05 ‘.’ 0.1 ‘ ’ 1
This is like an ANOVA table in ANOVA and regression models. All parameters are significantly different from zero. That is they contribute significantly in describing the relationships between our variables, but the overall lack of fit of the model suggests that they are not sufficient.
10.2.5 - Homogeneous Association
10.2.5 - Homogeneous AssociationThe homogeneous associations model is also known as a model of no-3-way interactions. Denoted by (AB, AC, BC), the only restriction this model imposes over the saturated model is that each pairwise conditional association doesn't depend on the value the third variable is fixed at. For example, the conditional odds ratio between \(A\) and \(B\), given \(C\) is fixed at its first level, must be the same as the conditional odds ratio between \(A\) and \(B\), given \(C\) is fixed at its second level, and so on.
Main assumptions
- The N = IJK counts in the cells are assumed to be independent observations of a Poisson random variable, and
- there is no three-way interaction among the variables: \(\lambda_{ijk}^{ABC}=0\) for all \(i, j, k\).
Model Structure
\(\log(\mu_{ijk})=\lambda+\lambda_i^A+\lambda_j^B+\lambda_k^C+\lambda_{ij}^{AB}+\lambda_{jk}^{BC}+\lambda_{ik}^{AC}\)
In terms of the Berkeley example, this model implies that the conditional association between department and sex does not depend on the fixed value of admission status, that the conditional association between sex and admission status does not depend on the fixed value of department, and the conditional association between department and admission status does not depend on the fixed value of sex.
Does this model fit? Even this model doesn't fit well, but it seems to fit better than the previous models. The deviance statistic is \(G^2= 20.2251\) with df= 5, and the Value/df is 4.0450.
Since the only terms that separate this model from the saturated one are those for the three-way interactions, the degrees of freedom must be \((I-1)(J-1)(K-1)\), which is \((2-1)(2-1)(6-1)=5\) in this example.
In SAS, this model can be fitted as:
proc genmod data=berkeley order=data;
class D S A;
model count = D S A D*S D*A S*A / dist=poisson link=log lrci type3 obstats;
run;
Are all terms in the model significant (e.g. look at "Type 3 Analysis output"); recall we need to use option "type3". For example, here is the ANOVA-like table that shows that SA association does not seem to be significant,
LR Statistics For Type 3 Analysis | |||
---|---|---|---|
Source | DF | Chi-Square | Pr > ChiSq |
D | 5 | 360.23 | <.0001 |
S | 1 | 252.58 | <.0001 |
A | 1 | 303.43 | <.0001 |
D*S | 5 | 1128.70 | <.0001 |
D*A | 5 | 763.40 | <.0001 |
S*A | 1 | 1.53 | 0.2159 |
Here is part of the output from the "Analysis of Parameter Estimates" given the values for all the parameters,
Analysis Of Maximum Likelihood Parameter Estimates | |||||||||
---|---|---|---|---|---|---|---|---|---|
Parameter | DF | Estimate | Standard Error |
Likelihood Ratio 95% Confidence Limits | Wald Chi-Square | Pr > ChiSq | |||
Intercept | 1 | 3.1374 | 0.1567 | 2.8166 | 3.4322 | 400.74 | <.0001 | ||
D | DeptA | 1 | 1.1356 | 0.1820 | 0.7858 | 1.5007 | 38.94 | <.0001 | |
D | DeptB | 1 | -0.3425 | 0.2533 | -0.8525 | 0.1450 | 1.83 | 0.1763 | |
D | DeptC | 1 | 2.2228 | 0.1649 | 1.9102 | 2.5579 | 181.77 | <.0001 | |
D | DeptD | 1 | 1.7439 | 0.1682 | 1.4241 | 2.0848 | 107.52 | <.0001 | |
D | DeptE | 1 | 1.4809 | 0.1762 | 1.1439 | 1.8361 | 70.64 | <.0001 | |
D | DeptF | 0 | 0.0000 | 0.0000 | 0.0000 | 0.0000 | . | . | |
S | Male | 1 | -0.0037 | 0.1065 | -0.2126 | 0.2048 | 0.00 | 0.9720 | |
S | Female | 0 | 0.0000 | 0.0000 | 0.0000 | 0.0000 | . | . | |
A | Reject | 1 | 2.6246 | 0.1577 | 2.3270 | 2.9467 | 276.88 | <.0001 | |
A | Accept | 0 | 0.0000 | 0.0000 | 0.0000 | 0.0000 | . | . | |
D*S | DeptA | Male | 1 | 2.0023 | 0.1357 | 1.7394 | 2.2717 | 217.68 | <.0001 |
D*S | DeptA | Female | 0 | 0.0000 | 0.0000 | 0.0000 | 0.0000 | . | . |
D*S | DeptB | Male | 1 | 3.0771 | 0.2229 | 2.6595 | 3.5364 | 190.63 | <.0001 |
D*S | DeptB | Female | 0 | 0.0000 | 0.0000 | 0.0000 | 0.0000 | . | . |
D*S | DeptC | Male | 1 | -0.6628 | 0.1044 | -0.8679 | -0.4587 | 40.34 | <.0001 |
D*S | DeptC | Female | 0 | 0.0000 | 0.0000 | 0.0000 | 0.0000 | . | . |
D*S | DeptD | Male | 1 | 0.0440 | 0.1057 | -0.1633 | 0.2513 | 0.17 | 0.6774 |
D*S | DeptD | Female | 0 | 0.0000 | 0.0000 | 0.0000 | 0.0000 | . | . |
D*S | DeptE | Male | 1 | -0.7929 | 0.1167 | -1.0226 | -0.5652 | 46.19 | <.0001 |
D*S | DeptE | Female | 0 | 0.0000 | 0.0000 | 0.0000 | 0.0000 | . | . |
D*S | DeptF | Male | 0 | 0.0000 | 0.0000 | 0.0000 | 0.0000 | . | . |
D*S | DeptF | Female | 0 | 0.0000 | 0.0000 | 0.0000 | 0.0000 | . | . |
D*A | DeptA | Reject | 1 | -3.3065 | 0.1700 | -3.6510 | -2.9834 | 378.38 | <.0001 |
D*A | DeptA | Accept | 0 | 0.0000 | 0.0000 | 0.0000 | 0.0000 | . | . |
D*A | DeptB | Reject | 1 | -3.2631 | 0.1788 | -3.6240 | -2.9220 | 333.12 | <.0001 |
D*A | DeptB | Accept | 0 | 0.0000 | 0.0000 | 0.0000 | 0.0000 | . | . |
D*A | DeptC | Reject | 1 | -2.0439 | 0.1679 | -2.3842 | -1.7247 | 148.24 | <.0001 |
D*A | DeptC | Accept | 0 | 0.0000 | 0.0000 | 0.0000 | 0.0000 | . | . |
D*A | DeptD | Reject | 1 | -2.0119 | 0.1699 | -2.3559 | -1.6884 | 140.18 | <.0001 |
D*A | DeptD | Accept | 0 | 0.0000 | 0.0000 | 0.0000 | 0.0000 | . | . |
D*A | DeptE | Reject | 1 | -1.5672 | 0.1804 | -1.9300 | -1.2213 | 75.44 | <.0001 |
D*A | DeptE | Accept | 0 | 0.0000 | 0.0000 | 0.0000 | 0.0000 | . | . |
D*A | DeptF | Reject | 0 | 0.0000 | 0.0000 | 0.0000 | 0.0000 | . | . |
D*A | DeptF | Accept | 0 | 0.0000 | 0.0000 | 0.0000 | 0.0000 | . | . |
S*A | Male | Reject | 1 | 0.0999 | 0.0808 | -0.0582 | 0.2588 | 1.53 | 0.2167 |
S*A | Male | Accept | 0 | 0.0000 | 0.0000 | 0.0000 | 0.0000 | . | . |
S*A | Female | Reject | 0 | 0.0000 | 0.0000 | 0.0000 | 0.0000 | . | . |
S*A | Female | Accept | 0 | 0.0000 | 0.0000 | 0.0000 | 0.0000 | . | . |
Scale | 0 | 1.0000 | 0.0000 | 1.0000 | 1.0000 |
The scale parameter was held fixed.
Recall, we are interested in the highest-order terms, thus two-way associations here, and they correspond to log-odds ratios. For example, the first coefficient 0.0999, reported in the row beginning with "S*A, male, reject", is the conditional log-odds ratio between sex and admission status. The interpretation is as follows: for a fixed department, the odds a male is rejected is \(\exp(0.0999)=1.10506\) times the odds that a female is rejected.
Although the department is fixed for this interpretation (so the comparison is among individuals applying to the same department), it doesn't matter which department we're focusing on; they all lead to the same result under this model. However, this model does not fit well, so we can't really rely on the inferences based on this model.
In R, here is one way of fitting this model (note that this syntax will also include all first-order terms automatically):
berk.ha = glm(Freq~(Admit+Gender+Dept)^2, family=poisson(), data=berk.data)
Here is part of the summary output that gives values for all the parameter estimates:
> summary(berk.ha)
...
Coefficients:
Estimate Std. Error z value Pr(>|z|)
(Intercept) 3.137358 0.156723 20.019 < 2e-16 ***
AdmitRejected 2.624559 0.157728 16.640 < 2e-16 ***
GenderMale -0.003731 0.106460 -0.035 0.972
DeptA 1.135552 0.181963 6.241 4.36e-10 ***
DeptB -0.342489 0.253251 -1.352 0.176
DeptC 2.222782 0.164869 13.482 < 2e-16 ***
DeptD 1.743872 0.168181 10.369 < 2e-16 ***
DeptE 1.480918 0.176194 8.405 < 2e-16 ***
AdmitRejected:GenderMale 0.099870 0.080846 1.235 0.217
AdmitRejected:DeptA -3.306480 0.169982 -19.452 < 2e-16 ***
AdmitRejected:DeptB -3.263082 0.178784 -18.252 < 2e-16 ***
AdmitRejected:DeptC -2.043882 0.167868 -12.176 < 2e-16 ***
AdmitRejected:DeptD -2.011874 0.169925 -11.840 < 2e-16 ***
AdmitRejected:DeptE -1.567174 0.180436 -8.685 < 2e-16 ***
GenderMale:DeptA 2.002319 0.135713 14.754 < 2e-16 ***
GenderMale:DeptB 3.077140 0.222869 13.807 < 2e-16 ***
GenderMale:DeptC -0.662814 0.104357 -6.351 2.13e-10 ***
GenderMale:DeptD 0.043995 0.105736 0.416 0.677
GenderMale:DeptE -0.792867 0.116664 -6.796 1.07e-11 ***
---
Signif. codes: 0 ‘***’ 0.001 ‘**’ 0.01 ‘*’ 0.05 ‘.’ 0.1 ‘ ’ 1
(Dispersion parameter for poisson family taken to be 1)
Null deviance: 2650.095 on 23 degrees of freedom
Residual deviance: 20.204 on 5 degrees of freedom
AIC: 217.26
Recall, we are interested in the highest-order terms, thus two-way associations here, and they correspond to log-odds ratios. For example, the first coefficient 0.0999, reported in the row beginning with "AdmitRejected:GenderMale", is the conditional log-odds ratio between sex and admission status. The interpretation is as follows: for a fixed department, the odds a male is rejected is \(\exp(0.0999)=1.10506\) times the odds that a female is rejected.
Although the department is fixed for this interpretation (so the comparison is among individuals applying to the same department), it doesn't matter which department we're focusing on; they all lead to the same result under this model. However, this model does not fit well (deviance statistic of 20.204 with 5 df), so we can't really rely on the inferences based on this model.
10.2.6 - Model Selection
10.2.6 - Model SelectionAccording to our usual approach for hierarchical models, where one is a special case of the other, we can use a likelihood ratio test to measure the reduction in fit of the smaller model (null hypothesis), relative to the larger model (alternative hypothesis). The degrees of freedom for these tests are the difference between the numbers of parameters involved between the two models.
Below is a summary of all possible models for the Berkeley admissions example data, with complete independence (most restrictive) at the top the saturated model at the bottom. The df, \(G^2\), and p-value columns correspond to the deviance (goodness-of-fit) test for each model compared with the saturated model.
Model | df | \(G^2\) | p-value |
---|---|---|---|
(D, S, A) | 16 | 2097.671 | < .001 |
(DS, A) | 11 | 877.056 | < .001 |
(D, SA) | 15 | 2004.222 | < .001 |
(DA, S) | 11 | 1242.350 | < .001 |
(DS, SA) | 10 | 783.607 | < .001 |
(DS, DA) | 6 | 21.736 | < .001 |
(DA, SA) | 10 | 1148.901 | < .001 |
(DS, DA, SA) | 5 | 20.204 | < .001 |
(DSA) | 0 | 0.00 |
Based on these results, the saturated model would be preferred because any reduced model has a significantly worse fit (all p-values are significant). However, if a reduced model would have been acceptable, relative to the saturated one, we could continue to test further reductions with likelihood ratio tests.
Likelihood Ratio Tests
Suppose the model of homogeneous association (HA) had been insignificantly different from the saturated model. We would then have preferred the HA model because it has fewer parameters and is easier to work with overall. And to test additional reductions, we could use the likelihood ratio test with the HA model as the alternative hypothesis (instead of the saturated one).
For example, to test the (DS, DA) model, which assumes sex and admission status are conditionally independent, given department, the hypotheses would be \(H_0\): (DS, DA) versus \(H_a\): (DS, DA, SA). The test statistic would be twice the difference between their log-likelihood values but could be computed directly from the deviance statistics above:
\(G^2=783.607-20.204 =763.4\)
Relative to a chi-square distribution with \(10-5=5\) degrees of freedom, this is would highly significant (p-value less than .001). If, however, this conditional independence model hadn't been rejected, we could place into the role of the alternative hypothesis to test the further reduced joint independence model (DS, A), and so on. As we've seen with our earlier models, this approach works for any full versus reduced model comparison.
10.3 - Lesson 10 Summary
10.3 - Lesson 10 SummaryIn this lesson, we introduced the log-linear model and showed how it can be used to explain the associations among a set of two or more categorical variables. Although many of the same results as those from a logistic model can be obtained, the main advantage of the log-linear model is that by not treating anyone variable as the response, it can estimate relationships among any of the variables, whereas the logistic model focuses only on relationships involving the response. The advantage of the logistic model, however, is that it can accommodate quantitative variables.
In the next lesson, we continue the discussion on log-linear models to include ordinal data and "dependent" data, which is effectively the categorical version of matched pairs.