Lesson 7 - Other Types of Study Designs: Cross-Sectional, Ecologic, Experimental
Lesson 7 - Other Types of Study Designs: Cross-Sectional, Ecologic, ExperimentalLesson 7 Objectives
- Compare advantages/ disadvantages of cross-sectional and ecological studies
- Describe ecological fallacy
- Describe the main difference between observational and experimental studies
- Identify design considerations unique to intervention studies including equipoise, randomization, and masking
7.1 - Cross sectional studies
7.1 - Cross sectional studiesRationale and Design
A cross-sectional study is a study with individual-level variables that measures exposure and disease at one point in time. In other words, cross-sectional studies take a snapshot of a population. These types of studies are often used for public health planning.
Advantages & Disadvantages of cross-sectional studies
Advantages
- Highly generalizable when based on a sample of the general population
- Low cost and short time period needed to conduct
Disadvantages
- Cannot infer temporal sequence between exposure and disease
- Identify a high proportion of prevalent cases of long duration
- Can suffer from the “healthy worker effect” - where those who remain employed tend to be healthier than those who leave employment.
Examples in Research
This includes the National Center for Health Statistics study on Products - Data Briefs - Number 443 - August 2022 (cdc.gov)
A key finding = The percentages of both men and women who met the guidelines for both aerobic and muscle-strengthening activities decreased with age.

This includes Talking With Children About Prognosis: The Decisions and Experiences of Mothers With Metastatic Cancer
A Key finding = Nearly 80% (n = 176) of mothers with metastatic cancer reported they had discussed their prognosis with at least one of their children; 79% identified at least one barrier to these discussions.
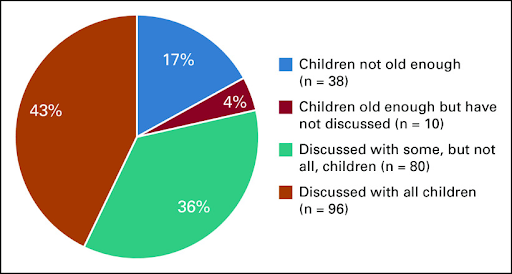
This includes Occupational health nurses’ personal attitudes toward smoking: A cross‐sectional study
A key finding =
Opinion | Training Experience a (n = 67) |
No Training Experience b (n = 41) |
Total |
P-value* |
|||
---|---|---|---|---|---|---|---|
n | % | n | % | n | % | ||
Smoking is harmful to health | |||||||
Strongly Agree | 62 | 92.5% | 31 | 75.6% | 93 | 86.1% | .024 |
Agree | 5 | 7.5% | 9 | 22.0% | 14 | 13.0% | |
Undecided | 0 | 0.0% | 1 | 2.4% | 1 | 0.9% | |
Disagree & Strongly Disagree |
0 | 0.0% | 0 | 0.0% | 0 | 0.0% | |
Healthcare professionals have a social responsibility to warn smokers of the harmful effects of smoking. | |||||||
Strongly Agree | 42 | 62.7% | 15 | 36.6% | 57 | 52.8% | .017 |
Agree | 22 | 32.8% | 20 | 48.8% | 42 | 38.9% | |
Undecided | 3 | 4.5% | 6 | 14.6% | 9 | 8.3% | |
Disagree & Strongly Disagree |
0 | 0.0% | 0 | 0.0% | 0 | 0.0% |
a Nurses with training experience in interventions to stop smoking.
b Nurses without training experience in interventions to stop smoking.
* Fischers exact test.
7.2 - Ecological studies
7.2 - Ecological studiesRationale and Ecological Variables
An ecological study is an observational study in which at least one variable is measured at the group level. An ecological study is especially appropriate for the initial investigation of causal hypotheses.
So...why conduct an ecological study? Several reasons support using an ecological study design.
- The hypothesis is relatively new
- Adequate measurement of individual-level variables is not possible
- Adequate design of an individual-level study is not possible (i.e., not ethical)
- We are interested in the effect of ecological variables, for which there is no correlation at the individual level
- We have limited funds or limited time to do the study
Three types of ecological variables:
- Aggregate Variables
- A summary or composite measure derived from values collected from individual members of a population. Aggregate variables can measure exposure (e.g., mean blood pressure) or outcome (e.g., rate of disease) variables. One limitation with aggregate measures is that there is variation within the population - not all the individuals in the population have the average blood pressure.
- Environmental Variables
- A measure of the physical characteristics of the environment in which people reside, work, recreate or attend school. For example, we might hypothesize that rainfall is a risk for a fungal disease or that the content of minerals in drinking water is protective against a certain disease. Therefore, environmental variables would be the mean rainfall in a geographic area or the mean level of minerals in drinking water. Environmental variables measure exposure, not outcomes. One limitation of an environmental variable is that there is variation in exposure levels for individuals in the population.
- Global Variables (Measure Exposure)
- A measure of the attributes of groups, organizations, or places for which there is no analog at the individual level. For example, the procedures or treatments that are covered in a health insurance plan might affect the rate of disease or adverse health outcomes. Additionally, population density would be another global variable because crowding might be an important exposure. There is no individual population density! Global variables are used to measure exposures, not outcomes.
Advantages & Disadvantages of Ecological Studies
Advantages
- Can be done quickly and inexpensively bc rely on pre-existing data
- Analysis and presentation are relatively simple
- Can achieve a wider range of exposure levels than can be expected from an individual-level study
- Help explain population-level associations
Disadvantages
- Ecological fallacy - the possibility of making incorrect conclusions about individual-level associations when only using group-level data
- Lack of information on important variables
Analysis of Ecologic Studies
Analytic models in ecologic studies are of different forms:
- Completely Ecologic
- All variables (outcome, exposure, and covariates) are ecological.
- Partially Ecologic
- Some, but not all, variables are ecological.
- Multilevel
- Analyses may simultaneously include individual and ecological variables on the same construct (e.g., income). This could be called multilevel modeling, hierarchical regression, or mixed-effects modeling.
7.2.1 - Sample Ecological Data and Analysis
7.2.1 - Sample Ecological Data and AnalysisThe following data illustrate a problem with the interpretation of ecological studies. The data include the numbers in an exposed and non-exposed group and the disease rate per 100,000 person-years within each of the three different groups.
With the data given, we can calculate the exposure rates per group as:
Exposure | Group 1 | Group 2 | Group 3 | ||||||
---|---|---|---|---|---|---|---|---|---|
Cases | PY |
Rate/ |
Cases | PY | Rate/ 100,000 PY |
Cases | PY | Rate/ 100,000 PY |
|
Exposed (x=1) | 20 | 7000 | 20 | 10000 | 20 | 13000 | |||
Unexposed (x=0) | 13 | 13000 | 10 | 10000 | 7 | 7000 | |||
Total | 33 | 20000 | 165 | 30 | 20000 | 150 | 27 | 20000 | 135 |
Exposure Rate | 35% | 50% | 65% |
What is the relationship between exposure level and disease rate per 100,000 person-years?
Once we can calculate the exposure rate in each group, we see that as exposure rates increase, disease rates decrease.
The natural conclusion would seem to be that exposure protects individuals from the disease by decreasing the rate of disease.
So...would you want to be exposed to this factor in order to cut your disease risk? Or would you like to ask further questions?
What about the fact that we have no data measured at the individual level? For example, do we know the exposure level and the disease outcome for each person in the study? NO! In fact, all the cases could have actually occurred among the exposed individuals. This would be a problem if our hypothesis was that a biological process was responsible for the increased risk.
Consider these tables:
Stratum 1 and Stratum 2 are similar to the groups, of which there were 3, in the previous example. We don't know the numbers for each cell within any stratum, nor do we know A, B, C, or D for the combined data. Only the marginal counts are known - the number exposed and unexposed, and the numbers of cases and non-cases within each stratum. So, if our hypothesis for the risk pathway is biological, then we run the risk of an ecological fallacy. An ecological fallacy is possible when we use group-level data as evidence for risk pathways that operate at the individual level because we are ascribing group observations to the individual! (Note: Group-level data are appropriate if our hypothesis is that the disease pathway is from a group-level exposure. Group-level exposures are recognized as important in disease causation models with both individual and group processes).
Individual-level Data and Analysis
To demonstrate the ecological fallacy, let's look at the individual-level data from the same example. We will fill in the number of cases within each cell for each group. For instance, in group 1, there were 20 cases in 7,000 person-years of being at-risk.
Then we can calculate the rates per 100,000PY for each exposure level in each group as:
Exposure | Group 1 | Group 2 | Group 3 | ||||||
---|---|---|---|---|---|---|---|---|---|
Cases | PY |
Rate/ |
Cases | PY | Rate/ 100,000 PY |
Cases | PY | Rate/ 100,000 PY |
|
Exposed (x=1) | 20 | 7000 | 286 | 20 | 10000 | 200 | 20 | 13000 | 154 |
Unexposed (x=0) | 13 | 13000 | 100 | 10 | 10000 | 100 | 7 | 7000 | 100 |
Total | 33 | 20000 | 165 | 30 | 20000 | 150 | 27 | 20000 | 135 |
Exposure Rate | 35% | 50% | 65% |
Next, we can calculate the Rate Difference and Rate Ratio within each group as
\(\text { Rate Difference }=\text { Rate }_{\text {Exposed }}-\text { Rate }_{\text {Unexposed }}\)
\(\text { Rate Ratio }=\dfrac{\text { Rate }_{\text {Exposed }}}{\text { Rate }_{\text {Unexposed }}}\)
Exposure | Group 1 | Group 2 | Group 3 | ||||||
---|---|---|---|---|---|---|---|---|---|
Cases | PY |
Rate/ |
Cases | PY | Rate/ 100,000 PY |
Cases | PY | Rate/ 100,000 PY |
|
Exposed (x=1) | 20 | 7000 | 286 | 20 | 10000 | 200 | 20 | 13000 | 154 |
Unexposed (x=0) | 13 | 13000 | 100 | 10 | 10000 | 100 | 7 | 7000 | 100 |
Total | 33 | 20000 | 165 | 30 | 20000 | 150 | 27 | 20000 | 135 |
Exposure Rate | 35% | 50% | 65% | ||||||
Rate Difference | 186 | 100 | 54 | ||||||
Rate Ratio | 2.86 | 2.00 | 1.54 |
When we look at each group separately, we see that exposure is related to a higher rate of disease!
So, we would conclude that exposure increases the risk of this outcome, which is the opposite of what we concluded previously! We also observe that the rate of disease among the non-exposed was the same for all groups. Across groups, the rate of disease among the exposed was higher than the unexposed, but the rate seems to vary among the exposed groups.
Recall, that when we used the group-level (ecological) data we saw that this exposure appeared to be protective. HOWEVER, given the individual-level data, exposure appears to increase the risk of disease! This is an example of an ecological fallacy (or ecological bias)... using group-level data to support an individual pathway.
Can an ecological study produce results without ecological bias? Yes, under certain conditions...
7.3 - Experimental Studies
7.3 - Experimental StudiesRationale and Design
Experimental studies are used to investigate the role of some agent or intervention in the causation, prevention, or treatment of a disease or outcome. The investigators assign patients to either receive (or not receive) the intervention, which is the most distinguishing differentiation between an experimental study and an observational study.
More can also be learned about experimental studies in both STAT 503 and STAT 509
Design considerations
Equipoise
Type of intervention (note: this is not an exhaustive list!)
- Drug
- Educational
- Behavioral
- Environmental
- Organizational (clinic level, hospital level)
Treatment assignment and randomization
- Methods
- Simple randomization - if the planned sample size for a study is n=100, a randomization scheme for assignment can be created. But it is possible that the computer-generated sample assignment could be that the first 50 patients are assigned control, and the last 50 are assigned to the treatment arm. This is not an ideal randomization scheme. To combat this, one can use blocking and/or stratification.
- Blocking - for the planned sample size of 100, one might choose to use block randomization with blocks of size 20. So, for patients 1-20, we’d know that there would be 10 patients assigned to the control arm and 10 to the treatment arm. This would be repeated for the next 4 sets of 20 enrolled patients. This method keeps randomization balanced over the course of the study enrollment.
- Stratification - There may be one variable that we believe is likely to be a strong confounder and we want to make sure it is balanced between arms. Thus, we perform randomization separately within each stratum.
- Assignment - the intervention can be assigned at either the individual level or at a cluster level
- Individual level - Each participant in the study is assigned to their experimental condition separately. When the treatment is a specific medication or educational/behavioral material given to the participant, this usually works fine. If, however, the study is being conducted in a medical center, and clinicians see patients in both the treatment and control arms of a study, there might be a spillover of the intervention into all patients.
- Cluster level - experimental conditions are assigned at a cluster/group level. In a hospital, this may be when clinicians are assigned to a certain condition, and thus all patients they see receive the same intervention. Or when many hospitals are selected for a trial, and the entire hospital does or does not receive the intervention. In a school, this could be at the classroom, grade, or even school level.
- Randomization
Random assignment is the ideal way to assign treatment conditions because it is less prone to bias. With a large enough sample size, researchers can feel confident that randomization controls for both known and unknown confounders- Methods
- Simple randomization - if the planned sample size for a study is n=100, a randomization scheme for assignment can be created. But it is possible that the computer-generated sample assignment could be that the first 50 patients are assigned control, and the last 50 are assigned to the treatment arm. This is not an ideal randomization scheme. To combat this, one can use blocking and/or stratification.
- Blocking - for the planned sample size of 100, one might choose to use block randomization with blocks of size 20. So, for patients 1-20, we’d know that there would be 10 patients assigned to the control arm and 10 to the treatment arm. This would be repeated for the next 4 sets of 20 enrolled patients. This method keeps randomization balanced over the course of the study enrollment.
- Stratification - There may be one variable that we believe is likely to be a strong confounder and we want to make sure it is balanced between arms. Thus, we perform randomization separately within each stratum.
- Methods
Masking
- Methods
- Drug trials often use placebo pills which look exactly the same, but do not contain the active ingredient of interest
- Sham procedures can be used that resemble the treatment, but this can be hard based on the nature of the procedure (ie. surgery)
- Trials that are evaluating educational interventions can provide the treatment group with all the official study material, but also provide the control group a basic pamphlet that may already be around the clinic so that both groups receive some reading material.
- Outcome Assessment - when an assessment of outcomes is subjective, it is very important to employ masking, and this can be done at different levels
- Single-masked: participants but not investigators are masked. If patients are the ones providing the assessment (via self-reports), this is usually sufficient.
- Double-masked: both participants and investigators are masked. If investigators are also tasked with providing some subjective report of efficacy it is best to keep investigators masked as well
- Triple-masked: participants, investigators, and monitoring committee members are all masked. This situation arises for larger trials when it is important to monitor serious adverse or beneficial events and possibly recommend an early ending for the study.
Advantages & Disadvantages
Advantages
- Can control study conditions to isolate effect of interest
- Random assignment to study conditions can eliminate baseline differences between groups
- Use of placebo controls can allow masking which prevents biased ascertainment of outcome measures
Disadvantages
- Can be very expensive
- Patients may be unwilling to be “guinea pigs” for science
- Ethical issues - only permissible when there is a state of equipoise in the medical community
- Placebo controls vs another effective treatment as control
- Patients may be non-compliant with treatment regimen
- Eligibility can be strict and not allow generalization back to all real-world scenarios
Examples in Research
Design elements include:
- Educational and organizational intervention
- Cluster randomized
- No masking
Design elements include:
- Medical device intervention (intervention arm= fully magnetically levitated centrifugal-flow, control arm = axial-flow LVAD
- Stratified randomization within each center, 1:1 randomization
- No masking
7.4 - Lesson 7 Summary
7.4 - Lesson 7 SummaryAdditional study designs included in this lesson were cross-sectional, ecological, and experimental. Along with case-control and cohort study designs, these give a good overview of typical study designs used for epidemiologic research. There are also other study designs as well that are not covered in this class, including specific types of experimental study designs. The most important thing to keep in mind is the research question. Only once the investigators are clear about the research question, can the team decide the best study design to use. The study design must fit and be able to answer the research question and not the other way around.
Cross-sectional studies take a snapshot of a population and are often used for public health planning. The National Health Interview Survey is an example of a cross-sectional survey that has been done over the years to monitor the health of the US population. Although it is done yearly, the same individuals are not included, so it is different from a cohort study. Ecological studies include at least one study variable measured at the group level. These can be very useful for the initial investigation of causal hypotheses, but it is important to be aware and cautious of the possibility of an ecological fallacy. Finally, experimental studies are in contrast to observational studies in that the investigators assign participants to certain study conditions. Both observational and experimental studies are important for the advancement of public health, and it is important to consider the pros and cons of each when deciding how best to study the research/policy/health question of interest.