Lesson 10 - Power and Sample Size Considerations
Lesson 10 - Power and Sample Size ConsiderationsLesson 10 Objectives
- Describe the rationale for sample size calculations
- Describe the relationships between sample size, power, variability, effect size, and significance level
- Distinguish between type I & II error
- Calculate the sample size needed for the following scenarios given the necessary preliminary data
- Single proportion
- Comparison of two proportions
- Unmatched case-control study
- Matched case-control study
- Single mean
- Comparison of two means
10.1 - Rationale and Type I & II Error
10.1 - Rationale and Type I & II ErrorOne reason for performing sample size calculations in the planning phase of a study is to assure confidence in the study results and conclusions. We certainly wish to propose a study that has a chance to be scientifically meaningful.
Are there other implications, beyond a lack of confidence in the results, to an inadequately-powered study? Suppose you are reviewing grants for a funding agency. If insufficient numbers of subjects are to be enrolled for the study to have a reasonable chance of finding a statistically significant difference, should the investigator receive funds from the granting agency? Of course not. The FDA, NIH, NCI, and most other funding agencies are concerned about sample size and power in the studies they support and do not consider funding studies that would waste limited resources.
Money is not the only limited resource. What about potential study subjects? Is it ethical to enroll subjects in a study with a small probability of producing clinically meaningful results, precluding their participation in a more adequately-powered study? What about the horizon of patients not yet treated? Are there ethical implications to conducting a study in which treatment and care actually help prolong life, yet due to inadequate power, the results are unable to alter clinical practice?
Too many subjects are also problematic. If more subjects are recruited than needed, the study is prolonged. Wouldn't it be preferable to quickly disseminate the results if the treatment is worthwhile instead of continuing a study beyond the point where a significant effect is clear? Or, if the treatment proves detrimental to some, how many subjects will it take for the investigator to conclude there is a clear safety issue?
Recognizing that careful consideration of statistical power and the sample size is critical to assuring scientifically meaningful results, protection of human subjects, and good stewardship of fiscal, tissue, physical, and staff resources, let's review how power and sample size are determined.
Type I and II Error
When we are planning the sample size to use for a new study, we want to balance the use of resources while minimizing the chance of making an incorrect conclusion. Suppose our study is comparing an outcome between groups.
When we simply do hypothesis testing, without a priori sample size calculations, we use alpha (\(\boldsymbol{\alpha})\)=0.05 as our typical cutoff for significance. In this situation, the \(\boldsymbol{\alpha}\) of 0.05 means that we are comfortable with a 5% chance that we incorrectly rejected the null hypothesis when it was in fact true. In other words, a 5% chance that we concluded a significant difference between groups when there was not actually a difference. It makes sense that we really want to minimize the chance of making this error. This type of error is referred to as a Type I error (\(\boldsymbol{\alpha}\)).
Power comes in when we ALSO want to ensure that our sample size is large enough to detect a difference when one truly exists. We want our study to have large power (usually at least 80%) to correctly reject the null hypothesis when it is false. In other words, we want a high chance that if there truly is a difference between groups, we detect it with our statistical test. The type of error that comes in when we fail to reject the null hypothesis when it is in fact false is type II error (\(\boldsymbol{\beta}\)). Power is defined as \(1 - \boldsymbol{\beta}\).
Possible outcomes for hypothesis testing
Decision | Reality | |
---|---|---|
Null is true | Null is NOT true | |
Don't reject null | Correct decision | Type II error (\(\boldsymbol{\beta}\)) - Miss detecting a difference when there is one |
Reject null | Type I error (\(\boldsymbol{\alpha}\)) - Conclude difference when there isn't one |
Correct decision |
Using the analogy of a trial, we want to make correct decisions: declare the guilty, 'guilty' and the innocent, 'innocent'. We do not wish to declare the innocent 'guilty' (\(\sim \boldsymbol{\alpha}\)) or the guilty 'innocent' (\(\sim \boldsymbol{\beta}\)).
Factors that affect the sample size needed for a study
- Alpha - is the level of significance, the probability of a Type I error (\(\boldsymbol{\alpha}\)). This is usually 5% or 1%, meaning the investigator is willing to accept this level of risk of declaring the null hypothesis false when it is actually true (i.e. concluding a difference when there is not one).
- Beta - is the probability of making a Type II error, and Power = (\(1 - \boldsymbol{\beta}\)). Beta is usually 20% or lower, resulting in a power of 80% or greater, meaning the investigator is willing to accept this level of risk of not rejecting the null hypothesis when in fact it should be rejected (i.e. missing a difference when one exists)
- Effect size - is the deviation from the null (or difference between groups) that the investigator wishes to be able to detect. The effect size should be clinically meaningful. It may be based on the results of prior or pilot studies. For example, a study might be powered to be able to detect a relative risk of 2 or greater.
- Variability - may be expressed in terms of a standard deviation, or an appropriate measure of variability for the statistic. The investigator will need an estimate of the variability in order to calculate the sample size. Reasonable estimates may be obtained from historical data, pilot study data, or a literature search.
Change in factor | Change in sample size |
---|---|
Alpha \(\downarrow\) | Sample size \(\uparrow\) |
Beta \(\downarrow\) (power \(\uparrow\)) | Sample size \(\uparrow\) |
Effect size \(\downarrow\) | Sample size \(\uparrow\) |
Variability \(\downarrow\) | Sample size \(\downarrow\) |
Sample Size Considerations
- There are nice closed-form formulas for many of the standard comparisons we are interested in. For other scenarios, formulas do not exist, and simulations must be used.
- When calculating necessary sample sizes, there are various options (formulas, tables, online calculators, proprietary software). It is worthwhile to use more than one to check yourself while you are still getting comfortable doing these calculations and until you find a method that works best for you. They will likely make slightly different assumptions or use slightly different formulas, but the results should be similar.
- It is often good to make a table to see how sample size estimates will change based on different assumptions.
- One-sided versus two-sided alpha (depends on hypothesis)
- Alpha (level of type I error you’re comfortable with)
- Power (level of type II error you’re comfortable with)
- Preliminary estimates (depends if you have good preliminary data, or you’re just hypothesizing the null values)
- Estimated differences (see how sample size changes based on difference you’re trying to detect)
10.2 - Test a Single Proportion
10.2 - Test a Single ProportionExample
The baseline prevalence of smoking in a particular community is 30%. A clean indoor air policy goes into effect. What is the sample size required to detect a decrease in smoking prevalence of at least 2 percentage points, with a one-sided alpha of 0.05 and a power of 90%?
Formula
We are interested in testing the following hypothesis:
\(\begin{array}{l}
\mathrm{H}_{0}\colon \pi=\pi_{0} \\
\mathrm{H}_{1}\colon \pi=\pi_{1}=\pi_{0}+d
\end{array}\)
Where \(\pi\) is the true proportion, \(\pi_0\) is some specified value for the proportion we wish to test (30% in our example), and \(\pi_1\) (which differs from \(\pi_0\) by an amount d (d= 2% in our example)) is the alternative value.
The formula needed to calculate the sample size is:
\(\displaystyle{n=\frac{1}{d^{2}}\left[z_{\alpha} \sqrt{\pi_{0}\left(1-\pi_{0}\right)}+z_{\beta} \sqrt{\pi_{1}\left(1-\pi_{1}\right)}\right]^{2}}\)
Where
- \(\pi_0\) = null hypothesized proportion
- d = estimated change in proportion
Note that we can replace \(z_a\) by \(z_{\alpha / 2}\) for a two-sided test.
The z terms can be found from a standard normal distribution table, and common values are shown below:
Significance level | |||||||
---|---|---|---|---|---|---|---|
One-sided | Two-sided | Power | |||||
5% |
1% 2.3263 |
0.1% 3.0902 |
5% 1.9600 |
1% 2.5758 |
0.1% 3.2905 |
90% 1.2816 |
95% 1.6449 |
(Chapter 8.5, p 305, Woodward book)
From the formula, we can calculate that n= 4,417.
The table below can also be used to estimate the necessary sample size. Note that the formula and the Woodward table define d as a positive change. Since we are testing a decrease (30% down to 28%), we need to assume \(pi_{0}\) is 70%, and that it will go up to 72%. We can think of it as testing the “non-smoking” prevalence.
Note that Woodward offers additional tables in his textbook which can be used for different power, and for a two-sided versus a one-sided test.
Sample Size Statement: A total sample size of n=4,417 is needed to detect a change in smoking prevalence of 2% (30% down to 28%) using a one-group chi-squared test with one-sided alpha of 0.05 and 90% power.
Table B.8. Sample size requirements for testing the value of a single proportion.
These tables give requirements for a one-sided test directly. For two-sided tests, use the table corresponding to half the required significance level. Note that \(\pi_{0}\) is the hypothesized proportion (under \(H_{0}\)) and \(d\) is the difference to be tested. | |||||||||||
---|---|---|---|---|---|---|---|---|---|---|---|
(a) 5% significance, 90% power \(\pi_{0}\) |
|||||||||||
\(d\) | 0.01 | 0.10 | 0.20 | 0.30 | 0.40 | 0.50 | 0.60 | 0.70 | 0.80 | 0.90 | 0.95 |
0.01 | 1 178 | 8 001 | 13 923 | 18 130 | 20 625 | 21 406 | 20 475 | 17 830 | 13 473 | 7 400 | 3 717 |
0.02 | 366 | 2 070 | 3 534 | 4 567 | 5 172 | 5 349 | 5 097 | 4 417 | 3 308 | 1 769 | 833 |
0.03 | 192 | 950 | 1 593 | 2 045 | 2 305 | 2 376 | 2 255 | 1 944 | 1 443 | 748 | 322 |
0.04 | 123 | 551 | 908 | 1 158 | 1 300 | 1 335 | 1 262 | 1 083 | 795 | 398 | 148 |
0.05 | 88 | 362 | 589 | 746 | 834 | 853 | 804 | 686 | 498 | 239 | |
0.06 | 67 | 258 | 414 | 521 | 580 | 591 | 555 | 471 | 338 | 155 | |
0.07 | 54 | 194 | 308 | 385 | 427 | 434 | 405 | 342 | 242 | 104 | |
0.08 | 44 | 152 | 238 | 296 | 327 | 331 | 308 | 258 | 181 | 71 | |
0.09 | 38 | 123 | 190 | 235 | 259 | 261 | 242 | 201 | 139 | 48 | |
0.10 | 32 | 102 | 156 | 191 | 210 | 211 | 195 | 161 | 109 | ||
0.15 | 18 | 49 | 72 | 87 | 93 | 92 | 83 | 66 | 40 | ||
0.20 | 12 | 30 | 42 | 49 | 52 | 50 | 44 | 33 | |||
0.25 | 9 | 20 | 27 | 31 | 33 | 31 | 26 | 18 | |||
0.30 | 7 | 14 | 19 | 22 | 22 | 20 | 16 | ||||
0.35 | 5 | 11 | 14 | 16 | 16 | 14 | 10 | ||||
0.40 | 4 | 9 | 11 | 12 | 11 | 10 | |||||
0.45 | 4 | 7 | 8 | 9 | 8 | 6 | |||||
0.50 | 3 | 6 | 7 | 7 | 6 |
(Tables from Woodward, M. Epidemiology Study Design and Analysis. Boca Raton: Chapman and Hall:, 2013)
Stop and Think!
Looking at the table values, what happens to the necessary sample size as:
- Prevalence increases (\(B_0\))? Does the sample size increase or decrease?
- What happens to the sample size as effect size decreases?
- What is the minimal detectable difference if you had funds for 1,500 subjects?
- The largest sample sizes occur with baseline prevalence at 0.5
- The smaller the effect size, the larger the sample size
- About 3.6% decrease in prevalence
10.3 - Compare Two Proportions
10.3 - Compare Two ProportionsExample
Suppose the rate of disease in an unexposed population is 10/100 person-years. You hypothesize an exposure has a relative risk of 2.0. How many persons must you enroll assuming half are exposed and half are unexposed to detect this increased risk, with a one-sided alpha of 0.05 and power of 90%?
Formula
We are interested in testing the following hypothesis:
\(\begin{array}{l}
\mathrm{H}_{0}\colon \pi_{1}=\pi_{2} \\
\mathrm{H}_{1}\colon \pi_{1}-\pi_{2}=\delta
\end{array}\)
But it is usually more convenient to consider the ratio (i.e. relative risk = λ), so we can consider this hypothesis:
\(\begin{array}{l}
\mathrm{H}_{0}: \pi_{1}=\pi_{2} \\
\mathrm{H}_{1}: \pi_{1} / \pi_{2}=\lambda
\end{array}\)
The formulas needed to calculate the total sample size are:
\(\displaystyle{n=\frac{r+1}{r(\lambda-1)^{2} \pi^{2}}\left[z_{\alpha} \sqrt{(r+1) p_{c}\left(1-p_{c}\right)}+z_{\beta} \sqrt{\lambda \pi(1-\lambda \pi)+r \pi(1-\pi)}\right]^{2}}\),
and
\(\displaystyle{p_{c}=\frac{\pi(r \lambda+1)}{r+1}}\)
where
\(\pi=\pi_{2}\) is the proportion in the reference group
\(\mathrm{r}=\mathrm{n}_{1} / \mathrm{n}_{2}\) (ratio of sample sizes in each group)
\(p_{0}=\) the common proportion over the two groups
When r = 1 (equal-sized groups), the formula above reduces to:
\(p_{c}=\dfrac{\pi(\lambda+1)}{2}=\dfrac{\pi_{1}+\pi_{2}}{2}\)
From the formula, we can calculate that n=433 total, thus n=217 per group.
The table below can also be used to estimate the necessary sample size. For the column with \(\pi\)=0.10, with \(\lambda\)=2.0, we see that n=448 total, with n=224 per group. Approximately the same as from the formula.
Sample Size statement: A sample size of n=217 per group (total of 434) is needed to detect an increased risk of disease (relative risk=2.0) when the proportions are 10% in the unexposed and 20% in the exposed groups, using a two group chi-squared test with one-sided alpha of 0.05 and 90% power.
Table B.9. Total sample size requirements (for the two groups combined) for testing the ratio
of two proportions (relative risk) with equal numbers in each group.
These tables give requirements for a one-sided test directly. For two-sided tests, use the table corresponding to half the required significance level. Note that \(\pi\) is the proportion for the reference group (the denominator) and \(\lambda\) is the relative risk to be tested. | |||||||||
---|---|---|---|---|---|---|---|---|---|
(a) 5% significance, 90% power \(\pi\) |
|||||||||
\(\lambda\) | 0.001 | 0.005 | 0.010 | 0.050 | 0.100 | 0.150 | 0.200 | 0.500 | 0.900 |
0.10 | 23 244 | 4 636 | 2 310 | 488 | 216 | 138 | 100 | 30 | 8 |
0.20 | 32 090 | 6 398 | 3 188 | 618 | 298 | 190 | 136 | 40 | 10 |
0.30 | 45 406 | 9 052 | 4 508 | 874 | 418 | 268 | 192 | 56 | 14 |
0.40 | 66 554 | 13 268 | 6 606 | 1 278 | 612 | 390 | 278 | 78 | 18 |
0.50 | 102 678 | 20 466 | 10 190 | 1 968 | 940 | 598 | 426 | 118 | 26 |
0.60 | 171 126 | 34 104 | 16 976 | 3 274 | 1 562 | 990 | 706 | 192 | 38 |
0.70 | 323 228 | 64 410 | 32 058 | 6 176 | 2 940 | 1 862 | 1 322 | 352 | 62 |
0.80 | 770 020 | 153 422 | 76 348 | 14 688 | 6 980 | 4 412 | 3 128 | 814 | 126 |
0.90 | 3 251 102 | 647 690 | 322 264 | 61 924 | 29 380 | 18 534 | 13 110 | 3 336 | 450 |
1.10 | 3 593 120 | 715 666 | 355 984 | 68 240 | 32 272 | 20 282 | 14 288 | 3 496 | 292 |
1.20 | 941 030 | 187 410 | 93 208 | 17 846 | 8 426 | 5 286 | 3 716 | 890 | |
1.30 | 437 234 | 87 068 | 43 298 | 8 280 | 3 904 | 2 444 | 1 714 | 402 | |
1.40 | 256 630 | 51 098 | 25 406 | 4 854 | 2 284 | 1 428 | 1 000 | 228 | |
1.50 | 171 082 | 34 062 | 16 934 | 3 232 | 1 518 | 948 | 662 | 148 | |
1.60 | 123 556 | 24 596 | 12 226 | 2 330 | 1 094 | 680 | 474 | 104 | |
1.80 | 74 842 | 14 896 | 7 402 | 1 408 | 658 | 408 | 284 | 58 | |
2.00 | 51 318 | 10 212 | 5 074 | 962 | 448 | 278 | 192 | ||
3.00 | 17 102 | 3 400 | 1 688 | 316 | 146 | 88 | 60 | ||
4.00 | 9 498 | 1 886 | 934 | 174 | 78 | 46 | 30 | ||
5.00 | 6 419 | 1 272 | 630 | 116 | 52 | 30 | |||
10.00 | 2 318 | 458 | 226 | 40 | |||||
20.00 | 992 | 194 | 94 |
(Tables from Woodward, M. Epidemiology Study Design and Analysis. Boca Raton: Chapman and Hall, 2013)
Stop and Think!
- Incidence rate increase \((\pi)\)?
- Relative risk decreases \((\lambda)\)?
- How would you use this table to determine sample size for 'protective' effects (i.e., nutritional components or medical procedures which prevent a negative outcome), as opposed to an increased risk?
- What is the minimal detectable relative risk if you had funds for 1000 subjects?
- n decreases
- Largest n is closest to l
- Protective effects would be those with \(\lambda \lt 1\)
- With a background rate of 10/100 and 1000 subjects, a relative risk of about 1.65 could be detected.
10.4 - Unmatched Case Control
10.4 - Unmatched Case ControlExample
An unmatched case-control study evaluating the association between smoking and CHD is planned.
If 30% of the population is estimated to be smokers, what is the number of study subjects (assuming an equal number of cases and controls in an unmatched study design) necessary to detect a hypothesized odds ratio of 2.0? Assume 90% power and a one-sided alpha of 0.05.
Formula
Due to the design of unmatched case-control studies, where unequal sampling rates are used for the exposed and unexposed, we cannot estimate relative risks for case-control studies, and must instead estimate odds ratios. The hypothesis we wish to test is slightly altered
\(\begin{array}{l}
\mathrm{H}_{0}^{*}\colon \pi_{1}^{*}=\pi_{2}^{*} \\
\mathrm{H}_{1}^{*}\colon \pi_{1}^{*} / \pi_{2}^{*}=\lambda^{*}
\end{array}\)
Where
\(\begin{array}{l}
\pi_{1}^{*}=p(\text { Exposed } \mid \text { Disease })=p(\text { Exposed } \mid \text { Case }) \\
\pi_{2}^{*}=p(\text { Exposed } \mid \text { No disease })=p(\text { Exposed } \mid \text { Control })
\end{array}\)
The formulas are similar to the formula for relative risk, but with additional parameters.
\(\begin{aligned}
n=\frac{(r+1)(1+(\lambda-1) P)^{2}}{r P^{2}(P-1)^{2}(\lambda-1)^{2}}[ & z_{\alpha} \sqrt{(r+1) p_{c}^{*}\left(1-p_{c}^{*}\right)} \\
& \left.+z_{\beta} \sqrt{\frac{\lambda P(1-P)}{[1+(\lambda-1) P]^{2}}+r P(1-P)}\right]^{2}
\end{aligned}\)
and
\(\displaystyle{p_{c}^{*}=\frac{P}{r+1}\left(\frac{r \lambda}{1+(\lambda-1) P}+1\right)}\)
Where
- \(\mathrm{P}=\) exposure prevalence
- \(\lambda=\) estimated relative risk
- r = ratio of cases to controls
From the formula, we can calculate that n=306 total, thus 153 cases and 153 controls.
The table below can also be used to estimate the necessary sample size. For the column with P=0.30, with \(\lambda\)=2.0, we see that n=306 total, with n=153 cases, and n=153 controls.
Sample Size statement: A total sample size of n=306 (153 cases and 153 controls) is needed to detect an OR of 2.0, assuming the prevalence of exposure is 30%, with one-sided alpha of 0.05 and 90% power.
Table B.10. Total sample size requirements (for the two groups combined) for unmatched case–control studies with equal numbers of cases and controls.
These tables give requirements for a one-sided test directly. For two-sided tests, use the table corresponding to half the required significance level. Note that \(P\) is the prevalence of the risk factor in the entire population and \(\lambda\) is the appropriate relative risk to be tested. | |||||||||
---|---|---|---|---|---|---|---|---|---|
(a) 5% significance, 90% power \(P\) |
|||||||||
\(\lambda\) | 0.010 | 0.050 | 0.100 | 0.200 | 0.300 | 0.400 | 0.500 | 0.700 | 0.900 |
0.10 | 2 318 | 456 | 224 | 108 | 70 | 50 | 40 | 30 | 38 |
0.20 | 3 206 | 638 | 316 | 158 | 104 | 80 | 66 | 56 | 88 |
0.30 | 4 546 | 912 | 458 | 232 | 160 | 124 | 106 | 98 | 176 |
0.40 | 6 676 | 1 348 | 684 | 356 | 248 | 200 | 176 | 172 | 330 |
0.50 | 10 318 | 2 098 | 1 074 | 566 | 404 | 332 | 296 | 306 | 616 |
0.60 | 17 220 | 3 522 | 1 816 | 974 | 706 | 588 | 536 | 576 | 1 206 |
0.70 | 32 570 | 6 698 | 3 476 | 1 890 | 1 390 | 1 174 | 1 088 | 1 206 | 2 612 |
0.80 | 77 686 | 16 052 | 8 382 | 4 614 | 3 438 | 2 944 | 2 764 | 3 146 | 7 012 |
0.90 | 328 374 | 68 156 | 35 786 | 19 922 | 15 020 | 13 006 | 12 354 | 14 400 | 32 892 |
1.10 | 363 666 | 76 090 | 40 352 | 22 918 | 17 630 | 15 574 | 15 096 | 18 316 | 43 550 |
1.20 | 95 332 | 20 020 | 10 664 | 6 112 | 4 744 | 4 228 | 4 134 | 5 102 | 12 340 |
1.30 | 44 334 | 9 342 | 4 998 | 2 888 | 2 260 | 2 032 | 2 002 | 2 510 | 6 166 |
1.40 | 26 044 | 5 506 | 2 958 | 1 722 | 1 358 | 1 230 | 1 222 | 1 554 | 3 870 |
1.50 | 17 376 | 3 684 | 1 986 | 1 166 | 926 | 846 | 846 | 1 090 | 2 748 |
1.60 | 12 558 | 2 672 | 1 446 | 854 | 684 | 628 | 632 | 826 | 2 106 |
1.80 | 7 618 | 1 630 | 888 | 532 | 432 | 400 | 408 | 546 | 1 420 |
2.00 | 5 230 | 1 124 | 616 | 374 | 306 | 288 | 296 | 404 | 1 074 |
3.00 | 1 754 | 386 | 218 | 138 | 120 | 118 | 126 | 184 | 522 |
4.00 | 978 | 220 | 126 | 84 | 74 | 76 | 84 | 130 | 380 |
5.00 | 664 | 150 | 88 | 60 | 56 | 58 | 66 | 104 | 316 |
10.00 | 244 | 60 | 38 | 30 | 30 | 34 | 40 | 70 | 224 |
20.00 | 108 | 30 | 20 | 18 | 20 | 24 | 30 | 56 | 190 |
(Tables from Woodward, M. Epidemiology Study Design and Analysis. Boca Raton: Chapman and Hall, 2013)
Stop and Think!
- Prevalence of the risk factor increases (P)?
- Odds ratio decreases (\(\lambda\))?
- For many \(\lambda\), 0.5 has the smallest sample size requirement
- largest sample sizes with OR closest to 1; 1.1 requires greater n than 0.9
10.5 - Matched Case Control
10.5 - Matched Case ControlExample
In contrast to the unmatched case-control study proposed in 10.4, here, assume we want to plan a matched case-control study evaluating the association between smoking and CHD.
A previous study suggested that the chance of a discordant pair is about 50%. What is the number of study subjects necessary to detect a hypothesized odds ratio of 2.0? Assume 90% power and a one-sided alpha of 0.05.
Formula
In matched case/control study designs, useful data come from only the discordant pairs of subjects. Useful information does not come from the concordant pairs of subjects. Matching of cases and controls on a confounding factor (e.g., age, sex) may increase the efficiency of a case-control study, especially when the moderator's minimal number of controls is rejected.
The sample size for matched study designs may be greater or less than the sample size required for similar unmatched designs because only the pairs discordant on exposure are included in the analysis. The proportion of discordant pairs must be estimated to derive sample size and power. The power of matched case/control study design for a given sample size may be larger or smaller than the power of an unmatched design.
The hypothesis to be tested is essentially that the number of discordant pairs that have an exposed case is 50% compared to the alternative that it is different from 50%.
The formulas for sample size calculation for matched case-control study are:
\(\displaystyle{d_{p}=\frac{\left[z_{\alpha}(\lambda+1)+2 z_{\beta} \sqrt{\lambda}\right]^{2}}{(\lambda-1)^{2}}}\) and \(\displaystyle{n=2 d_{p} / \pi_{d}}\)
Where
- \(\mathrm{Dp}=\) number of discordant pairs needed
- n = total number of matched pairs
- \(\lambda =\) estimated relative risk
- \(\boldsymbol{\pi}_{\mathrm{d}}=\) probability of a discordant pair
From the formula, we can calculate that \(d_{p}\) = 73.19, and then n=292.7, so rounding up to the next nearest even number, the study needs 294 individuals - that is, 147 pairs.
In this scenario, conducting a matched case-control study provides a saving of 12 compared with the unmatched version.
Sample Size statement: A total sample size of n=294 (147 matched case-control pairs) is needed to detect an OR of 2.0, assuming the prevalence of exposure is 30%, with one-sided alpha of 0.05 and 90% power.
10.6 - Compare a Single Mean
10.6 - Compare a Single MeanExample
Suppose the male population of an area in a developing country is known to have had a mean serum total cholesterol of 5.5 mmol/l 10 years ago, with an estimated standard deviation of 1.4 mmol/l. In recent years Western food has been imported into the country and is believed to have increased cholesterol levels. The investigators want to see if mean cholesterol levels have increased a clinically meaningful amount (up to about 6 mmol/l, a difference of 0.5 mmol/l) with a one-sided alpha of 0.05 and a power of 90%.
Formula
We are interested in testing the following hypothesis:
\(\mathrm{H}_{0}\colon \mu=\mu_{0}\)
\(\mathrm{H}_{1}\colon \mu=\mu_{1}\)
The formula needed to calculate the sample size is:
\(\displaystyle{n=\frac{\left(z_{\alpha}+z_{\beta}\right)^{2} \sigma^{2}}{\left(\mu_{1}-\mu_{0}\right)^{2}}}\)
Where
- \(\mu_{0}\)= null hypothesized value
- \(\mu_{1}\)= alternative hypothesized value
- \(\sigma\) = standard deviation
From the formula, we can calculate that n=67.1, so rounding to the next whole number would be n=68 .
To use the table below, we can calculate S= (6.0 – 5.5)/1.4 = 0.3571. This exact value does not appear in Table B.7. In these situations, we can get a rough idea of sample size by taking the nearest figure for S. In the example, the nearest tabulated figure is 0.35, which has n = 70 (for one-sided 5% significance and 90% power). This is only slightly above the true value of 67 for S = 0.3571. However, this process can lead to considerable error when S is small, so it is preferable to use the formula.
Sample Size Statement: A total sample size of n=68 is needed to detect a 0.5 mmol/l increase in mean cholesterol compared to an historical value of 5.5 mmol/l using a one-group t- test with one-sided alpha of 0.05 and 90% power, and assuming a standard deviation of 1.4.
Table B.7. Sample size requirements for testing the value of a single mean or the difference between two means.
The table gives requirements for testing a single mean with a one-sided test directly. For two-sided tests, use the column corresponding to half the required significance level. For tests of the difference between two means, the total sample size (for the two groups combined) is obtained by multiplying the requirement given below by 4 if the two samples sizes are equal or by \((r+1)^{2} / r\) if the ratio of the first to the second is \(r : 1\) (assuming equal variances). Note that \(S\) = difference/standard deviation. |
||||||||||||
---|---|---|---|---|---|---|---|---|---|---|---|---|
5% Significance |
2.5% Significance |
1% Significance |
0.5% Significance |
0.1% Significance |
0.05% Significance |
|||||||
\(S\) | 90% Power |
95% Power |
90% Power |
95% Power |
90% Power |
95% Power |
90% Power |
95% Power |
90% Power |
95% Power |
90% Power |
95% Power |
0.01 | 85 639 | 108 222 | 105 075 | 129 948 | 130 170 | 157 705 | 148 794 | 178 142 | 191 125 | 224 211 | 209 040 | 243 580 |
0.02 | 21 410 | 27 056 | 26 269 | 32 487 | 32 543 | 39 427 | 37 199 | 44 536 | 47 782 | 56 053 | 52 260 | 60 895 |
0.03 | 9 516 | 12 025 | 11 675 | 14 439 | 14 464 | 17 523 | 16 533 | 19 794 | 21 237 | 24 913 | 23 227 | 27 065 |
0.04 | 5 353 | 6 764 | 6 568 | 8 122 | 8 136 | 9 587 | 9 300 | 11 134 | 11 946 | 14 014 | 13 065 | 15 224 |
0.05 | 3 426 | 4 329 | 4 203 | 5 198 | 5 207 | 6 309 | 5 952 | 7 126 | 7 645 | 8 969 | 8 362 | 9 744 |
0.06 | 2 379 | 3 007 | 2 919 | 3 610 | 3 616 | 4 381 | 4 134 | 4 949 | 5 310 | 6 229 | 5 807 | 6 767 |
0.07 | 1 748 | 2 209 | 2 145 | 2 652 | 2 657 | 3 219 | 3 037 | 3 636 | 3 901 | 4 576 | 4 267 | 4 972 |
0.08 | 1 339 | 1 691 | 1 642 | 2 031 | 2 034 | 2 465 | 2 325 | 2 784 | 2 987 | 3 504 | 3 267 | 3 806 |
0.09 | 1 058 | 1 334 | 1 298 | 1 605 | 1 608 | 1 947 | 1 837 | 2 200 | 2 360 | 2 769 | 2 581 | 3 008 |
0.10 | 857 | 1 083 | 1 051 | 1 300 | 1 302 | 1 578 | 1 488 | 1 782 | 1 912 | 2 243 | 2 091 | 2 436 |
0.15 | 381 | 481 | 467 | 578 | 579 | 701 | 662 | 792 | 850 | 997 | 930 | 1 083 |
0.20 | 215 | 271 | 263 | 325 | 326 | 395 | 372 | 446 | 478 | 561 | 523 | 609 |
0.25 | 138 | 174 | 169 | 208 | 209 | 253 | 239 | 286 | 306 | 359 | 335 | 390 |
0.30 | 96 | 121 | 117 | 145 | 145 | 176 | 166 | 198 | 213 | 250 | 233 | 271 |
0.35 | 70 | 89 | 86 | 107 | 107 | 129 | 122 | 146 | 157 | 184 | 171 | 199 |
0.40 | 54 | 68 | 66 | 82 | 82 | 99 | 93 | 112 | 120 | 141 | 131 | 153 |
0.45 | 43 | 54 | 52 | 65 | 65 | 78 | 74 | 88 | 95 | 111 | 104 | 121 |
0.50 | 35 | 44 | 43 | 52 | 53 | 64 | 60 | 72 | 77 | 90 | 84 | 98 |
0.55 | 29 | 36 | 35 | 43 | 44 | 53 | 50 | 59 | 64 | 75 | 70 | 81 |
from Woodward, M. Epidemiology Study Design and Analysis. Boca Raton: Chapman and Hall, 2013, p.770
10.7 - Compare Two Means
10.7 - Compare Two MeansExample
Suppose investigators plan an intervention study to help individuals lower their cholesterol, and randomize patients to participate in their new intervention or a control group. They hypothesize at the end of their 6-month intervention the intervention group will have cholesterol levels down to about 5.3, while the control group's cholesterol levels will still be about 6. They assume the standard deviation will still be about 1.4. What sample size is needed to detect this difference with a one-sided alpha of 0.05 and a power of 90%?
Formula
We are interested in testing the following hypothesis:
\(\begin{array}{l}
\mathrm{H}_{0}\colon \mu_{1}=\mu_{2} \\
\mathrm{H}_{1}\colon \mu_{1}-\mu_{2}=\delta,
\end{array}\)
The formula needed to calculate the sample size is:
\(\displaystyle{n=\frac{(r+1)^{2}\left(z_{\alpha}+z_{\beta}\right)^{2} \sigma^{2}}{\delta^{2} r}}\)
Where...
- \(\mu_{1}\)= hypothesized mean in group 1
- \(\mu_{2}\)= hypothesized mean in group 2
- \(\delta\)= difference in means (null hypothesis \(\delta = 0\), alternative hypothesis \(\delta \ne 0\))
- \(\sigma\) = standard deviation
- \(r = \dfrac{n_1}{n_2}\)
From the formula, we can calculate that n=137, but after rounding to the next highest even number, n=138, with 69 per group.
To use the table below, we can calculate S= (6.0-5.3)/1.4 = 0.5. For a one-sided alpha of 0.05, we need to use the column for alpha =5%, and 90% power. Reading down to S=0.5, we see n=35. Back to the table header directions, we see that for a test of the difference between two means, we need to multiply the value by 4. Thus, 35*4 = 140 is the total sample size needed.
Sample Size Statement: A total sample size of n=138 (69 per group) is needed to detect a 0.7 mmol/l difference in mean cholesterol using a two-group t- test with one-sided alpha of 0.05 and 90% power, and assuming a common standard deviation of 1.4.
Table B.7. Sample size requirements for testing the value of a single mean or the difference between two means.
The table gives requirements for testing a single mean with a one-sided test directly. For two-sided tests, use the column corresponding to half the required significance level. For tests of the difference between two means, the total sample size (for the two groups combined) is obtained by multiplying the requirement given below by 4 if the two samples sizes are equal or by \((r+1)^{2} / r\) if the ratio of the first to the second is \(r : 1\) (assuming equal variances). Note that \(S\) = difference/standard deviation. |
||||||||||||
---|---|---|---|---|---|---|---|---|---|---|---|---|
5% Significance |
2.5% Significance |
1% Significance |
0.5% Significance |
0.1% Significance |
0.05% Significance |
|||||||
\(S\) | 90% Power |
95% Power |
90% Power |
95% Power |
90% Power |
95% Power |
90% Power |
95% Power |
90% Power |
95% Power |
90% Power |
95% Power |
0.01 | 85 639 | 108 222 | 105 075 | 129 948 | 130 170 | 157 705 | 148 794 | 178 142 | 191 125 | 224 211 | 209 040 | 243 580 |
0.02 | 21 410 | 27 056 | 26 269 | 32 487 | 32 543 | 39 427 | 37 199 | 44 536 | 47 782 | 56 053 | 52 260 | 60 895 |
0.03 | 9 516 | 12 025 | 11 675 | 14 439 | 14 464 | 17 523 | 16 533 | 19 794 | 21 237 | 24 913 | 23 227 | 27 065 |
0.04 | 5 353 | 6 764 | 6 568 | 8 122 | 8 136 | 9 587 | 9 300 | 11 134 | 11 946 | 14 014 | 13 065 | 15 224 |
0.05 | 3 426 | 4 329 | 4 203 | 5 198 | 5 207 | 6 309 | 5 952 | 7 126 | 7 645 | 8 969 | 8 362 | 9 744 |
0.06 | 2 379 | 3 007 | 2 919 | 3 610 | 3 616 | 4 381 | 4 134 | 4 949 | 5 310 | 6 229 | 5 807 | 6 767 |
0.07 | 1 748 | 2 209 | 2 145 | 2 652 | 2 657 | 3 219 | 3 037 | 3 636 | 3 901 | 4 576 | 4 267 | 4 972 |
0.08 | 1 339 | 1 691 | 1 642 | 2 031 | 2 034 | 2 465 | 2 325 | 2 784 | 2 987 | 3 504 | 3 267 | 3 806 |
0.09 | 1 058 | 1 334 | 1 298 | 1 605 | 1 608 | 1 947 | 1 837 | 2 200 | 2 360 | 2 769 | 2 581 | 3 008 |
0.10 | 857 | 1 083 | 1 051 | 1 300 | 1 302 | 1 578 | 1 488 | 1 782 | 1 912 | 2 243 | 2 091 | 2 436 |
0.15 | 381 | 481 | 467 | 578 | 579 | 701 | 662 | 792 | 850 | 997 | 930 | 1 083 |
0.20 | 215 | 271 | 263 | 325 | 326 | 395 | 372 | 446 | 478 | 561 | 523 | 609 |
0.25 | 138 | 174 | 169 | 208 | 209 | 253 | 239 | 286 | 306 | 359 | 335 | 390 |
0.30 | 96 | 121 | 117 | 145 | 145 | 176 | 166 | 198 | 213 | 250 | 233 | 271 |
0.35 | 70 | 89 | 86 | 107 | 107 | 129 | 122 | 146 | 157 | 184 | 171 | 199 |
0.40 | 54 | 68 | 66 | 82 | 82 | 99 | 93 | 112 | 120 | 141 | 131 | 153 |
0.45 | 43 | 54 | 52 | 65 | 65 | 78 | 74 | 88 | 95 | 111 | 104 | 121 |
0.50 | 35 | 44 | 43 | 52 | 53 | 64 | 60 | 72 | 77 | 90 | 84 | 98 |
0.55 | 29 | 36 | 35 | 43 | 44 | 53 | 50 | 59 | 64 | 75 | 70 | 81 |
(from Woodward, M. Epidemiology Study Design and Analysis. Boca Raton: Chapman and Hall, 2013, p.770)
10.8 - Additional Sample Size Topics
10.8 - Additional Sample Size TopicsRatio of Cases to Controls
Another consideration for sample size is if the same number of cases and controls should be used.
Power increases but at a decreasing rate as the ratio of controls/cases increases. Little additional power is gained at ratios higher than four controls/cases. There is little benefit to enrolling a greater ratio of controls to cases.
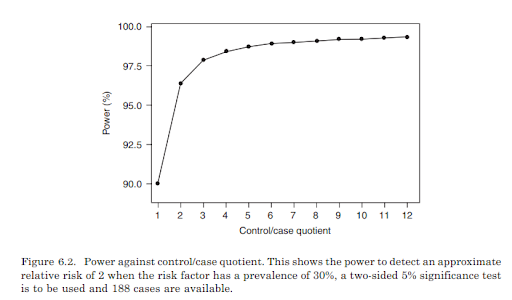
Under what circumstances would it be recommended to enroll a large number of controls compared to cases?
Perhaps the small gain in power is worthwhile if the cost of a Type II error is large and the expense of obtaining controls is minimal, such as selecting controls with covariate information from a computerized database. If you must physically locate and recruit the controls, set up clinic appointments, run diagnostic tests, and enter data, the effort of pursuing a large number of controls quickly offsets any gain. You would use a one-to-one or two-to-one range. The bottom line is there is little additional power beyond a four-to-one ratio.
Cohort v Case-control sample sizes
Sample sizes for cohort studies depend upon the rate of the outcome, not the prevalence of exposure. Sample size for case-control studies is dependent upon prevalence of exposure, not the rate of outcome. Because the rate of outcome is usually smaller than the prevalence of the exposure, cohort studies typically require larger sample sizes to have the same power as a case-control study.
The example below is from a study of smoking and coronary heart disease where the background incidence rate was 0.09 events per person-year among the non-exposed group and the prevalence of the risk factor was 0.3.
The sample size requirements to detect a given relative risk with the 90% power using two-sided 5% significance tests for cohort and case-control studies are listed below:
Relative Risk | Cohort study | Case-Control study |
---|---|---|
1.1 | 44,398 | 21,632 |
1.2 | 11,568 | 5,820 |
1.3 | 5,346 | 2,774 |
1.4 | 3,122 | 1,668 |
1.5 | 2,070 | 1,138 |
2 | 602 | 376 |
3 | 188 | 146 |
In such a situation, with a relative risk of 1.1, more than twice the number of subjects are required for a cohort study as for a case-control study. In every study in the table, the case-control design requires a smaller sample than does the cohort study to detect the same level of increased risk. This is generally true. There is also a dependence upon the rate of the outcome, but in general, case-control studies involve less sampling.
Furthermore, in designing a cohort study, loss-to-follow-up is important to consider. Based on your own experience or that of the literature, any sample size calculation should be inflated to account for the expected drop-outs. For example, if the drop-out rate is expected to be 5%, multiply n by 1/(1-0.05) and recruit the increased number of subjects.
10.9 - Summary
10.9 - SummaryCalculating the necessary sample size is important for the planning of a study, and sample size justification is often required when requesting funding. This is because we want to make sure we have enough participants to detect an effect if there is one and not too many that wastes resources (participant time/effort, cost, time), all while minimizing potential errors.
The two types of error occur when you 1) reject the null hypothesis when it is in fact true (type I) and 2) miss rejecting the null hypothesis when it is in fact false (type II error). In practice, you’ll never know if you made either of these errors, but using an appropriate sample size (based on prior knowledge) is a good way to do your best to minimize these possible errors. Most study’s primary objectives fall into the categories discussed in this section, and once you have the necessary estimates needed for the sample size calculations, these formulas can help you decide on the sample size for the study. Often when we are comparing two groups, equal sample sizes in each group are the best choice, but there are situations when unequal sample sizes are appropriate.
Sample size calculations are only as good as the preliminary data put into the formulas, so it is important to use the best information available, and if needed, to run pilot studies first to get good preliminary data.