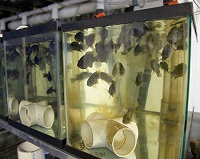
An experimental unit is an item (or physical entity) that receives the treatment. Identifying the experimental unit can be a trivial task in most experiments, but there can be exceptions.
For example...
Consider a situation where the effect of polluted stream water on fish lesions is to be studied. Two aquaria each with 50 fish are used for the study. The water treatment (polluted vs. control) is randomly assigned to each of the aquaria. After 30 days, the number of lesions was counted from randomly caught 10 fish from each aquarium. The treatment design is a single-factor design with 2 levels of water treatment, and a one-way ANOVA can be run on the data... but what is the experimental unit?Going back to our definition, the experimental unit is the entity that receives the treatment. In this case, we have applied a water treatment to each aquarium. The fish are not the experimental units. In order for individual fish to be experimental units, somehow the investigators would have to take one fish at a time and apply the treatment independently to each fish. This would be impractical from a logistics standpoint and was not done. Instead, the water treatment levels were applied to the entire aquarium, and so the experimental unit is an aquarium with 50 fish.
Now we can determine what constitutes a replication of the experiment. Each time the full set of treatment levels (2 levels in our example) is applied, we have a complete replication. Therefore in the experiment described there is only one replication; a situation often described as an un-replicated study.
The individual fish that were caught and counted for lesions are sampling units. Sampling units are the entities from which the observations are recorded. Traditionally, to obtain a correct ANOVA, mean values of the sampling units have to be computed for each experimental unit before the calculation of the treatment SS. Failure to recognize sampling units can result in a serious problem: pseudo-replication. Pseudo-replication results from treating each sampling unit as if it were an experimental unit and inflating the error degrees of freedom. By artificially increasing the error df, we reduce the MSE and produce a larger (incorrect) F-statistic.