The textbook we are using brings an engineering perspective to the design of experiments. We will bring in other contexts and examples from other fields of study including agriculture (where much of the early research was done) education and nutrition. Surprisingly the service industry has begun using design of experiments as well.
All experiments are designed experiments, it is just that some are poorly designed and some are well-designed.
Engineering Experiments Section
If we had infinite time and resource budgets there probably wouldn't be a big fuss made over designing experiments. In production and quality control we want to control the error and learn as much as we can about the process or the underlying theory with the resources at hand. From an engineering perspective we're trying to use experimentation for the following purposes:
- reduce time to design/develop new products & processes
- improve performance of existing processes
- improve reliability and performance of products
- achieve product & process robustness
- perform evaluation of materials, design alternatives, setting component & system tolerances, etc.
We always want to fine-tune or improve the process. In today's global world this drive for competitiveness affects all of us both as consumers and producers.
Robustness is a concept that enters into statistics at several points. At the analysis, stage robustness refers to a technique that isn't overly influenced by bad data. Even if there is an outlier or bad data you still want to get the right answer. Regardless of who or what is involved in the process - it is still going to work. We will come back to this notion of robustness later in the course (Lesson 12).
Every experiment design has inputs. Back to the cake baking example: we have our ingredients such as flour, sugar, milk, eggs, etc. Regardless of the quality of these ingredients we still want our cake to come out successfully. In every experiment there are inputs and in addition, there are factors (such as time of baking, temperature, geometry of the cake pan, etc.), some of which you can control and others that you can't control. The experimenter must think about factors that affect the outcome. We also talk about the output and the yield or the response to your experiment. For the cake, the output might be measured as texture, flavor, height, size, or flavor.
Four Eras in the History of DOE Section
Here's a quick timeline:
- The agricultural origins, 1918 – 1940s
- R. A. Fisher & his co-workers
- Profound impact on agricultural science
- Factorial designs, ANOVA
- The first industrial era, 1951 – late 1970s
- Box & Wilson, response surfaces
- Applications in the chemical & process industries
- The second industrial era, late 1970s – 1990
- Quality improvement initiatives in many companies
- CQI and TQM were important ideas and became management goals
- Taguchi and robust parameter design, process robustness
- The modern era, beginning circa 1990, when economic competitiveness and globalization are driving all sectors of the economy to be more competitive.
Immediately following World War II the first industrial era marked another resurgence in the use of DOE. It was at this time that Box and Wilson (1951) wrote the key paper in response surface designs thinking of the output as a response function and trying to find the optimum conditions for this function. George Box died early in 2013. And, an interesting fact here - he married Fisher's daughter! He worked in the chemical industry in England in his early career and then came to America and worked at the University of Wisconsin for most of his career.
The Second Industrial Era - or the Quality Revolution
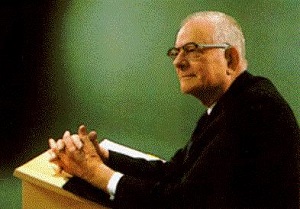
W. Edwards Deming
The importance of statistical quality control was taken to Japan in the 1950s by W Edward Deming. This started what Montgomery calls a second Industrial Era, and sometimes the quality revolution. After the second world war, Japanese products were of terrible quality. They were cheaply made and not very good. In the 1960s their quality started improving. The Japanese car industry adopted statistical quality control procedures and conducted experiments which started this new era. Total Quality Management (TQM), Continuous Quality Improvement (CQI) are management techniques that have come out of this statistical quality revolution - statistical quality control and design of experiments.
Taguchi, a Japanese engineer, discovered and published a lot of the techniques that were later brought to the West, using an independent development of what he referred to as orthogonal arrays. In the West, these were referred to as fractional factorial designs. These are both very similar and we will discuss both of these in this course. He came up with the concept of robust parameter design and process robustness.
The Modern Era
Around 1990 Six Sigma, a new way of representing CQI, became popular. Now it is a company and they employ a technique which has been adopted by many of the large manufacturing companies. This is a technique that uses statistics to make decisions based on quality and feedback loops. It incorporates a lot of previous statistical and management techniques.
Clinical Trials
Montgomery omits in this brief history a major part of design of experimentation that evolved - clinical trials. This evolved in the 1960s when medical advances were previously based on anecdotal data; a doctor would examine six patients and from this wrote a paper and published it. The incredible biases resulting from these kinds of anecdotal studies became known. The outcome was a move toward making the randomized double-blind clinical trial the gold standard for approval of any new product, medical device, or procedure. The scientific application of the statistical procedures became very important.