Women’s Health Survey: One-Sample Hotelling's T-Square Section
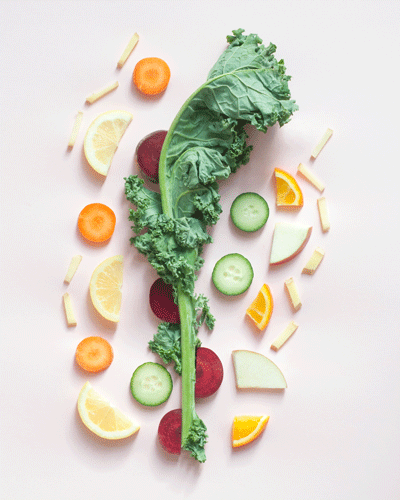
In 1985, the USDA commissioned a study of women’s nutrition. Nutrient intake was measured for a random sample of 737 women aged 25-50 years. Five nutritional components were measured: calcium, iron, protein, vitamin A, and vitamin C. In previous analyses of these data, the sample mean vector was calculated. The table below shows the recommended daily intake and the sample means for all the variables:
Variable | Recommended Intake \((\mu_{o})\) | Mean |
---|---|---|
Calcium | 1000 mg | 624.0 mg |
Iron | 15mg | 11.1 mg |
Protein | 60g | 65.8 g |
Vitamin A | 800 μg | 839.6 μg |
Vitamin C | 75 mg | 78.9 mg |
One of the questions of interest is whether women meet the federal nutritional intake guidelines. If they fail to meet the guidelines, then we might ask for which nutrients the women fail to meet the guidelines.
The hypothesis of interest is that women meet nutritional standards for all nutritional components. This null hypothesis would be rejected if women fail to meet nutritional standards on any one or more of these nutritional variables. In mathematical notation, the null hypothesis is the population mean vector \(μ\) equals the hypothesized mean vector \(\mu_{o}\) as shown below:
\(H_{o}\colon \mu = \mu_{o}\)
Let us first compare the univariate case with the analogous multivariate case in the following tables.
Focus of Analysis Section
Measuring only a single nutritional component (e.g. Calcium).
Data: scalar quantities \(X _ { 1 } , X _ { 2 } , \ldots , X _ { n }\)
Measuring multiple (say p) nutritional components (e.g. Calcium, Iron, etc).
Data: p × 1 random vectors
\(\mathbf{X} _ { 1 } , \mathbf{X} _ { 2 } , \ldots , \mathbf{X} _ { n }\)
Assumptions Made In Each Case Section
Distribution
The data all have a common mean \(\mu\) mathematically, \(E \left( X _ { i } \right) = \mu; i = 1,2, \dots, n\) This implies that there is a single population of subjects and no sub-populations with different means.
The data have a common mean vector \(\boldsymbol{\mu}\); i.e., \(E \left( \boldsymbol { X } _ { i } \right) =\boldsymbol{\mu}; i = 1,2, . , n \) This also implies that there are no sub-populations with different mean vectors.
Homoskedasticity
The data have common variance \(\sigma^{2}\) ; mathematically, \(\operatorname { var } \left( X _ { i } \right) = \sigma ^ { 2 } ; i = 1,2 , . , n\)
The data for all subjects have common variance-covariance matrix \(Σ\) ; i.e., \(\operatorname { var } \left( \boldsymbol{X} _ { i } \right) = \Sigma ; i = 1,2 , \dots , n\)
Independence
The subjects are independently sampled.
The subjects are independently sampled.
Normality
The subjects are sampled from a normal distribution
The subjects are sampled from a multivariate normal distribution.
Hypothesis Testing in Each Case Section
Consider hypothesis testing:
\(H _ { 0 } \colon \mu = \mu _ { 0 }\)
against alternative
\(H _ { \mathrm { a } } \colon \mu \neq \mu _ { 0 }\)
Consider hypothesis testing:
\(H _ { 0 } \colon \boldsymbol{\mu} = \boldsymbol{\mu _ { 0 }}\) against \(H _ { \mathrm { a } } \colon \boldsymbol{\mu} \neq \boldsymbol{\mu _ { 0 }}\)
Here our null hypothesis is that mean vector \(\boldsymbol{\mu}\) is equal to some specified vector \(\boldsymbol{\mu_{0}}\). The alternative is that these two vectors are not equal.
We can also write this expression as shown below:
\(H_0\colon \left(\begin{array}{c}\mu_1\\\mu_2\\\vdots \\ \mu_p\end{array}\right) = \left(\begin{array}{c}\mu^0_1\\\mu^0_2\\\vdots \\ \mu^0_p\end{array}\right)\)
The alternative, again is that these two vectors are not equal.
\(H_a\colon \left(\begin{array}{c}\mu_1\\\mu_2\\\vdots \\ \mu_p\end{array}\right) \ne \left(\begin{array}{c}\mu^0_1\\\mu^0_2\\\vdots \\ \mu^0_p\end{array}\right)\)
Another way of writing this null hypothesis is shown below:
\(H_0\colon \mu_1 = \mu^0_1\) and \(\mu_2 = \mu^0_2\) and \(\dots\) and \(\mu_p = \mu^0_p\)
The alternative is that μj is not equal to \(\mu^0_j\) for at least one j.
\(H_a\colon \mu_j \ne \mu^0_j \) for at least one \(j \in \{1,2, \dots, p\}\)
Univariate Statistics: \(t\)-test Section
In your introductory statistics course, you learned to test this null hypothesis with a t-statistic as shown in the expression below:
\(t = \dfrac{\bar{x}-\mu_0}{\sqrt{s^2/n}} \sim t_{n-1}\)
Under \(H _ { 0 } \) this t-statistic has a t distribution with n-1 degrees of freedom. We reject \(H _ { 0 } \) at level \(α\) if the absolute value of the test statistic t is greater than the critical value from the t-table, evaluated at \(α/2\) as shown below:
\(|t| > t_{n-1, \alpha/2}\)